Distributed Event-Triggered Iterative Learning Control for Multiple High-Speed Trains With Switching Topologies: A Data-Driven Approach
IEEE Transactions on Intelligent Transportation Systems(2023)
摘要
This paper studies the distributed data-driven event-triggered model free adaptive iterative learning control (ETMFAILC) of multiple high-speed trains (MHSTs) under iteration-varying topologies, which breaks away from the dependence on the train dynamics. Firstly, the nonlinear MHSTs with unknown dynamics are converted into a linear model. Then, combining the proposed event-based triggering condition and the linear model, the ETMFAILC scheme under the fixed topology is designed. Next, theoretical analysis proves the bounded input bounded output (BIBO) stability of MHSTs. Finally, the study is extended to the switching topologies and the validity of the ETMFAILC is verified by a numerical example.
更多查看译文
AI 理解论文
溯源树
样例
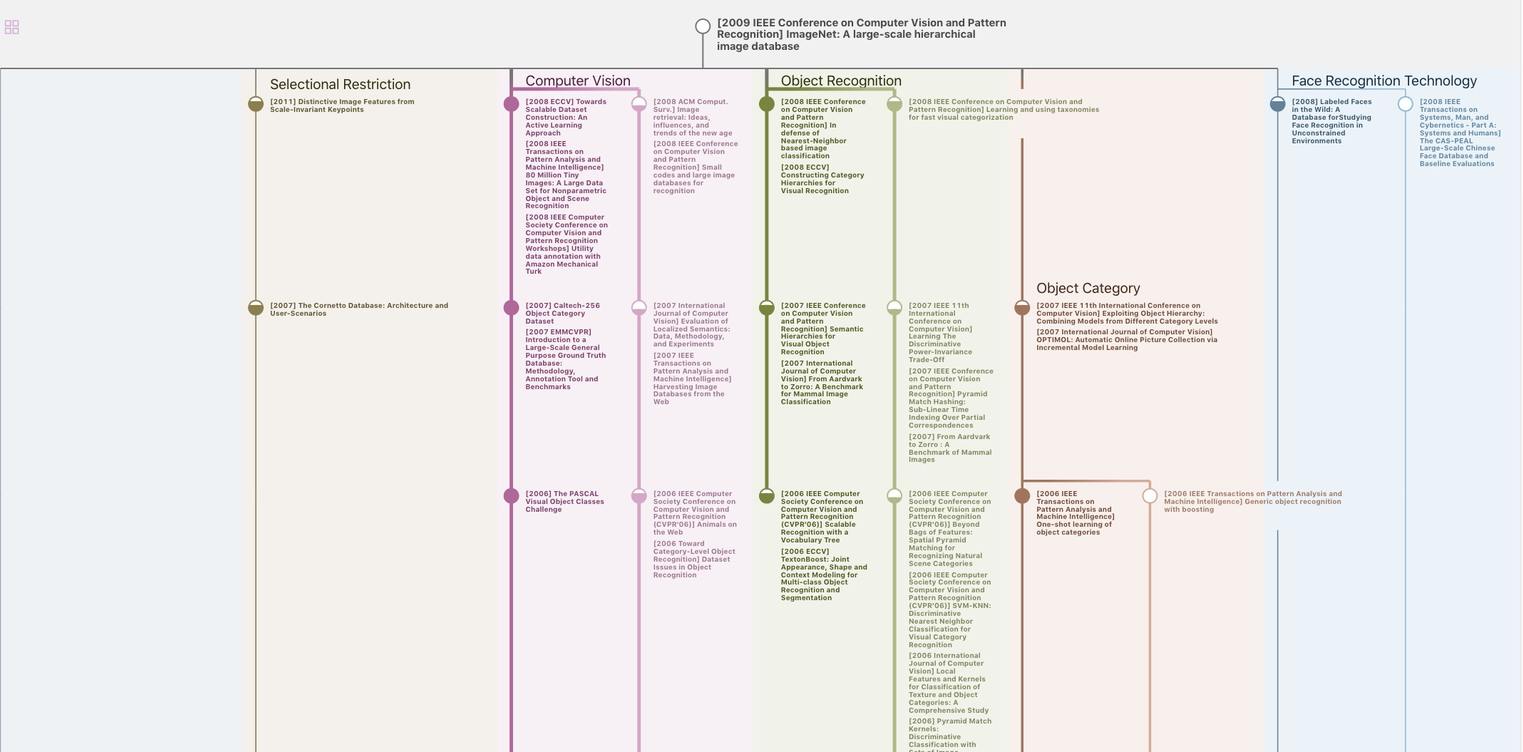
生成溯源树,研究论文发展脉络
Chat Paper
正在生成论文摘要