ContourPose: Monocular 6-D Pose Estimation Method for Reflective Textureless Metal Parts
IEEE Transactions on Robotics(2023)
摘要
Pose estimation is an essential technology for industrial robots to perform precise gripping and assembly. The state-of-the-art deep learning-based approach uses an indirect strategy, i.e., first finding local correspondence between the 2-D image and 3-D model, and then using the perspective-n-point and RANSAC methods to calculate the poses of ordinary objects. However, the metal parts in industry are reflective and textureless, making it difficult to identify distinguishable point features to establish 2-D–3-D correspondences. To address this problem, in this article, we propose a novel deep learning based two-stage method for pose estimation of reflective textureless metal parts, which accurately estimates the target pose using monocular red green blue (RGB) images. Since contours play an important role in both keypoints prediction and pose estimation stages, our method is named ContourPose. First, an additional contour decoder is adopted to implicitly constrain the keypoints prediction in the former stage, which improves the accuracy of the keypoints prediction. Then, the predicted contour of the previous stage is taken as geometric prior that is used to iteratively solve for the optimal pose. Experiments indicate that the proposed approach for reflective textureless metal parts has a significant improvement over the state-of-the-art approaches.
更多查看译文
关键词
Deep learning, reflective textureless metal parts, six degrees of freedom (6-D) pose estimation
AI 理解论文
溯源树
样例
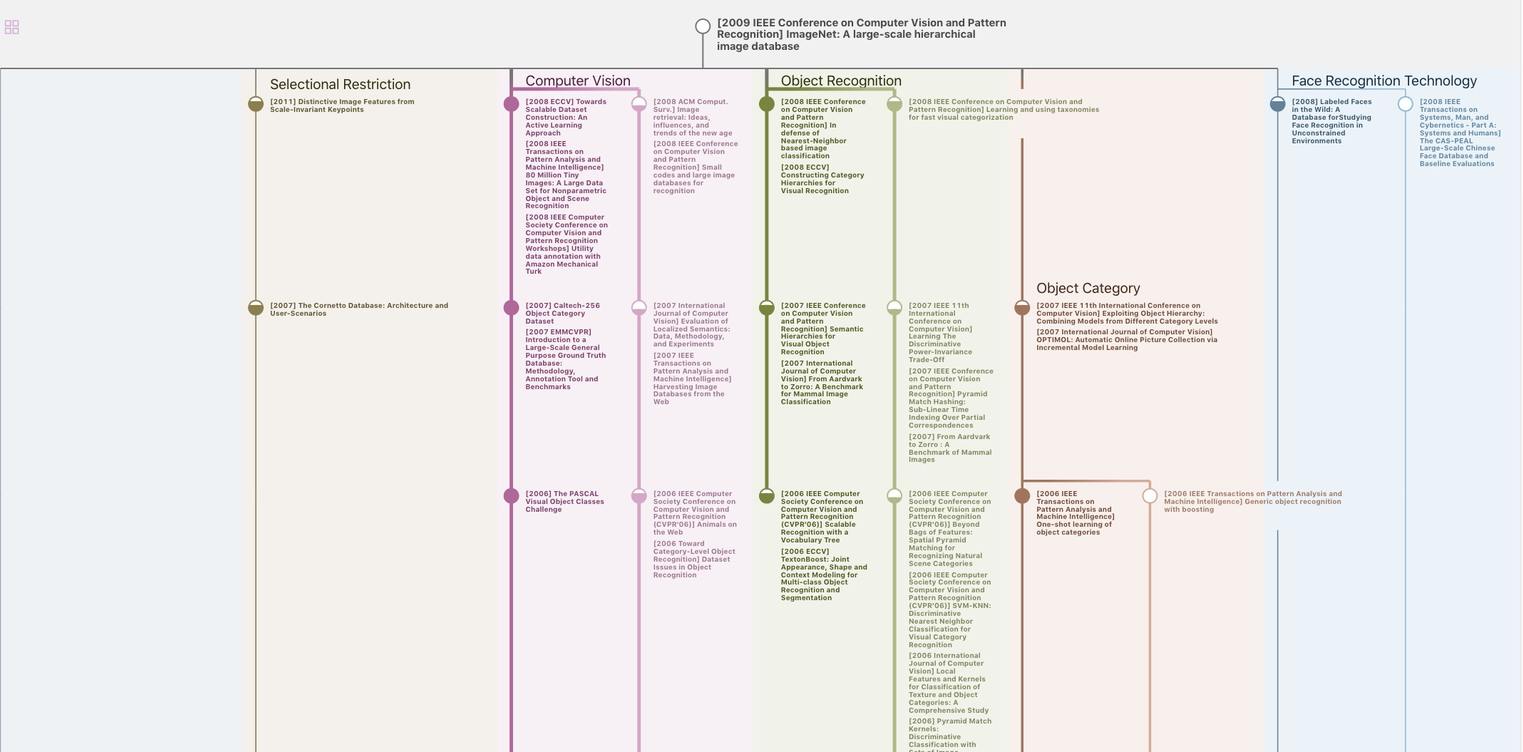
生成溯源树,研究论文发展脉络
Chat Paper
正在生成论文摘要