Reinforcement Learning of CPG-Regulated Locomotion Controller for a Soft Snake Robot
IEEE Transactions on Robotics(2023)
摘要
Intelligent control of soft robots is challenging due to the nonlinear and difficult-to-model dynamics. One promising model-free approach for soft robot control is reinforcement learning (RL). However, model-free RL methods tend to be computationally expensive and data-inefficient and may not yield natural and smooth locomotion patterns for soft robots. In this work, we develop a bioinspired design of a learning-based goal-tracking controller for a soft snake robot. The controller is composed of two modules: An RL module for learning goal-tracking behaviors given the unmodeled and stochastic dynamics of the robot, and a central pattern generator (CPG) with the Matsuoka oscillators for generating stable and diverse locomotion patterns. We theoretically investigate the maneuverability of Matsuoka CPG's oscillation bias, frequency, and amplitude for steering control, velocity control, and sim-to-real adaptation of the soft snake robot. Based on this analysis, we proposed a composition of RL and CPG modules such that the RL module regulates the tonic inputs to the CPG system given state feedback from the robot, and the output of the CPG module is then transformed into pressure inputs to pneumatic actuators of the soft snake robot. This design allows the RL agent to naturally learn to entrain the desired locomotion patterns determined by the CPG maneuverability. We validated the optimality and robustness of the control design in both simulation and real experiments, and performed extensive comparisons with state-of-art RL methods to demonstrate the benefit of our bioinspired control design.
更多查看译文
关键词
Biomimetics,deep reinforcement learning (RL),learning and adaptive systems,neural oscillator,soft robot control
AI 理解论文
溯源树
样例
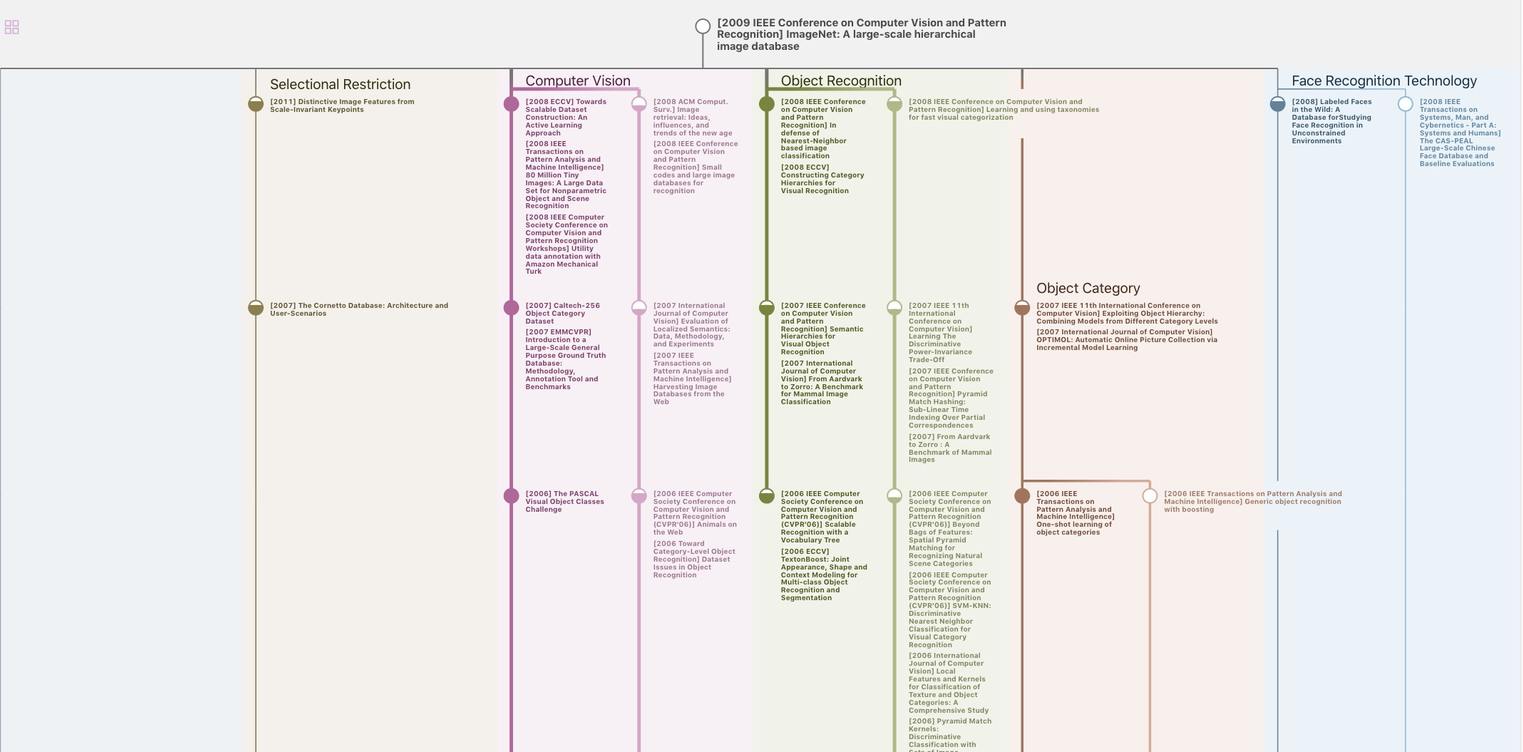
生成溯源树,研究论文发展脉络
Chat Paper
正在生成论文摘要