JsonTuning: Towards Generalizable, Robust, and Controllable Instruction Tuning
CoRR(2023)
摘要
Instruction tuning has emerged as a crucial process for harnessing the
capabilities of large language models (LLMs) by providing explicit task
instructions, leading to improved performance in various tasks. However,
prevalent text-to-text instruction tuning (TextTuning) methods suffer from
limitations in generalization, robustness, and controllability due to the
ambiguity and lack of explicit structure in tasks. In this paper, we propose
JsonTuning, a novel structure-to-structure approach for instruction tuning. By
leveraging the versatility and structured nature of JSON to represent tasks,
JsonTuning enhances generalization by helping the model understand essential
task elements and their relations, improves robustness by minimizing ambiguity,
and increases controllability by providing explicit control over the output. We
conduct a comprehensive comparative study with diverse language models and
evaluation benchmarks. Experimental results show that JsonTuning outperforms
TextTuning in various applications, showcasing improved performance,
adaptability, robustness, and controllability. By overcoming the limitations of
TextTuning, JsonTuning demonstrates significant potential for more effective
and reliable LLMs capable of handling diverse scenarios.
更多查看译文
关键词
controllable instruction
AI 理解论文
溯源树
样例
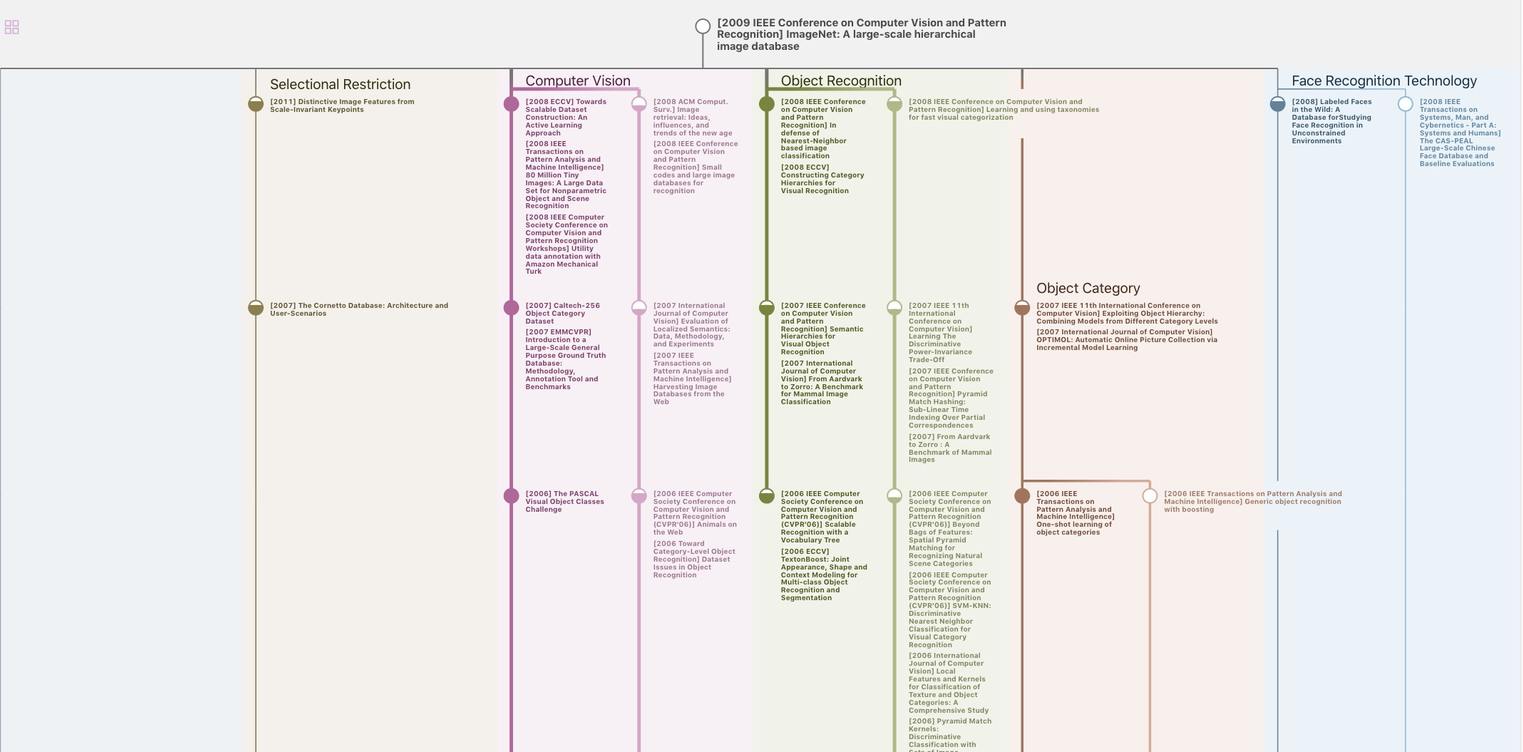
生成溯源树,研究论文发展脉络
Chat Paper
正在生成论文摘要