A novel asymmetrical autoencoder with a sparsifying discrete cosine Stockwell transform layer for gearbox sensor data compression
ENGINEERING APPLICATIONS OF ARTIFICIAL INTELLIGENCE(2024)
摘要
The lack of an efficient compression model remains a challenge for the wireless transmission of gearbox data in non-contact gear fault diagnosis problems. In this paper, we present a signal-adaptive asymmetrical autoencoder with a transform domain layer to compress sensor signals. First, a new discrete cosine Stockwell transform (DCST) layer is introduced to replace linear layers in a multi-layer autoencoder. A trainable filter is implemented in the DCST domain by utilizing the multiplication property of the convolution. A trainable hard-thresholding layer is applied to reduce redundant data in the DCST layer to make the feature map sparse. In comparison to the linear layer, the DCST layer reduces the number of trainable parameters and improves the accuracy of data reconstruction. Second, training the autoencoder with a sparsifying DCST layer only requires a small number of datasets. The proposed method is superior to other autoencoder-based methods on the University of Connecticut (UoC) and Southeast University (SEU) gearbox datasets, as the average quality score is improved by 2.00% at the lowest and 32.35% at the highest with a limited number of training samples.
更多查看译文
关键词
Gearbox sensor data compression,Autoencoder,Discrete cosine stockwell transform,Transform domain layer,Limited samples
AI 理解论文
溯源树
样例
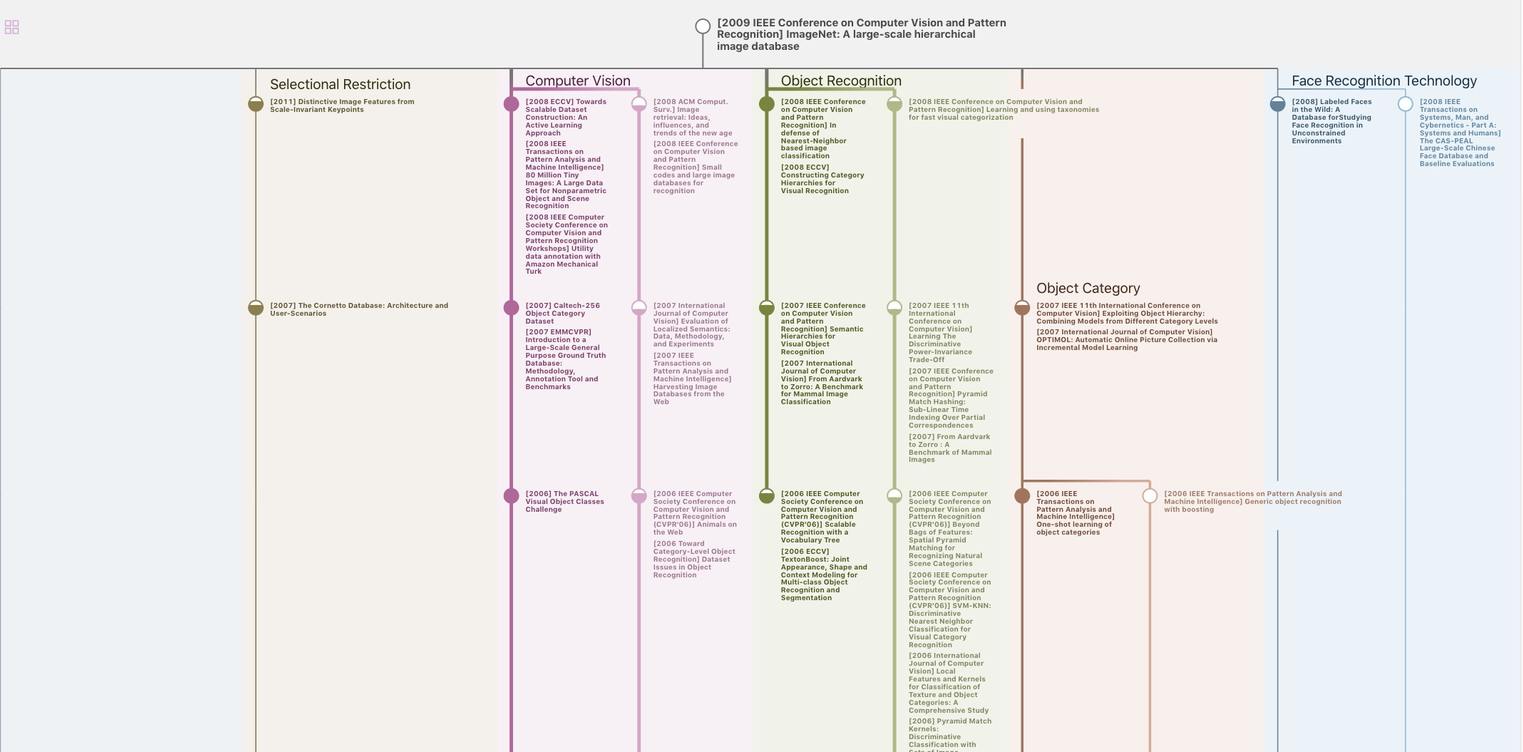
生成溯源树,研究论文发展脉络
Chat Paper
正在生成论文摘要