Low Resource Summarization using Pre-trained Language Models
CoRR(2023)
摘要
With the advent of Deep Learning based Artificial Neural Networks models, Natural Language Processing (NLP) has witnessed significant improvements in textual data processing in terms of its efficiency and accuracy. However, the research is mostly restricted to high-resource languages such as English and low-resource languages still suffer from a lack of available resources in terms of training datasets as well as models with even baseline evaluation results. Considering the limited availability of resources for low-resource languages, we propose a methodology for adapting self-attentive transformer-based architecture models (mBERT, mT5) for low-resource summarization, supplemented by the construction of a new baseline dataset (76.5k article, summary pairs) in a low-resource language Urdu. Choosing news (a publicly available source) as the application domain has the potential to make the proposed methodology useful for reproducing in other languages with limited resources. Our adapted summarization model \textit{urT5} with up to 44.78\% reduction in size as compared to \textit{mT5} can capture contextual information of low resource language effectively with evaluation score (up to 46.35 ROUGE-1, 77 BERTScore) at par with state-of-the-art models in high resource language English \textit{(PEGASUS: 47.21, BART: 45.14 on XSUM Dataset)}. The proposed method provided a baseline approach towards extractive as well as abstractive summarization with competitive evaluation results in a limited resource setup.
更多查看译文
关键词
resource,pre-trained
AI 理解论文
溯源树
样例
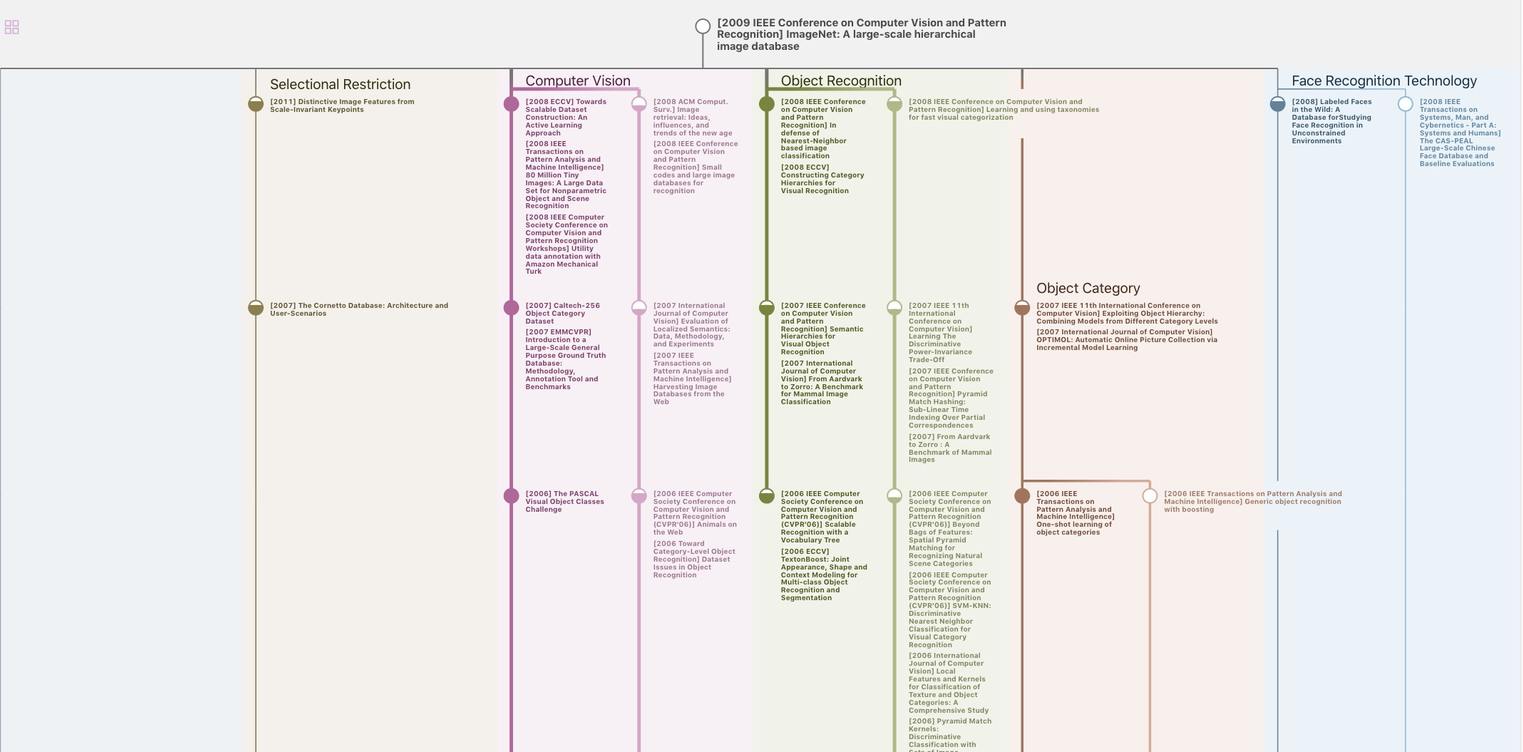
生成溯源树,研究论文发展脉络
Chat Paper
正在生成论文摘要