A combining earthquake forecasting model between deep learning and Epidemic-Type Aftershock Sequence (ETAS) model
arXiv (Cornell University)(2023)
摘要
The scientific process of earthquake forecasting involves estimating the probability and intensity of earthquakes in a specific area within a certain timeframe, based on seismic activity laws and observational data. Epidemic-Type Aftershock Sequence (ETAS) models, which rely on seismic empirical laws, is one of the most commonly used methods for earthquake forecasting. However, it underestimates feature in short-term time scale and overestimates in long-term time scale. Convolutional Long Short-Term Memory (ConvLSTM), has emerged as a promising approach capable of extracting spatio-temporal features. Hence, we propose a novel composite model named CL-ETAS model, which combines the strengths of ConvLSTM and ETAS model. We conduct experimental verification on real seismic data in Southern California. Our results show that CL-ETAS model outperforms both ETAS model and ConvLstm in accurately forecasting the number of earthquake events, their magnitude, and spatial distribution. Additionally, CL-ETAS model demonstrates notable enhancements in forecast stability and interpretability. These results offer valuable insights and recommendations for future earthquake forecasting endeavors.
更多查看译文
关键词
earthquake forecasting model,deep learning,epidemic-type
AI 理解论文
溯源树
样例
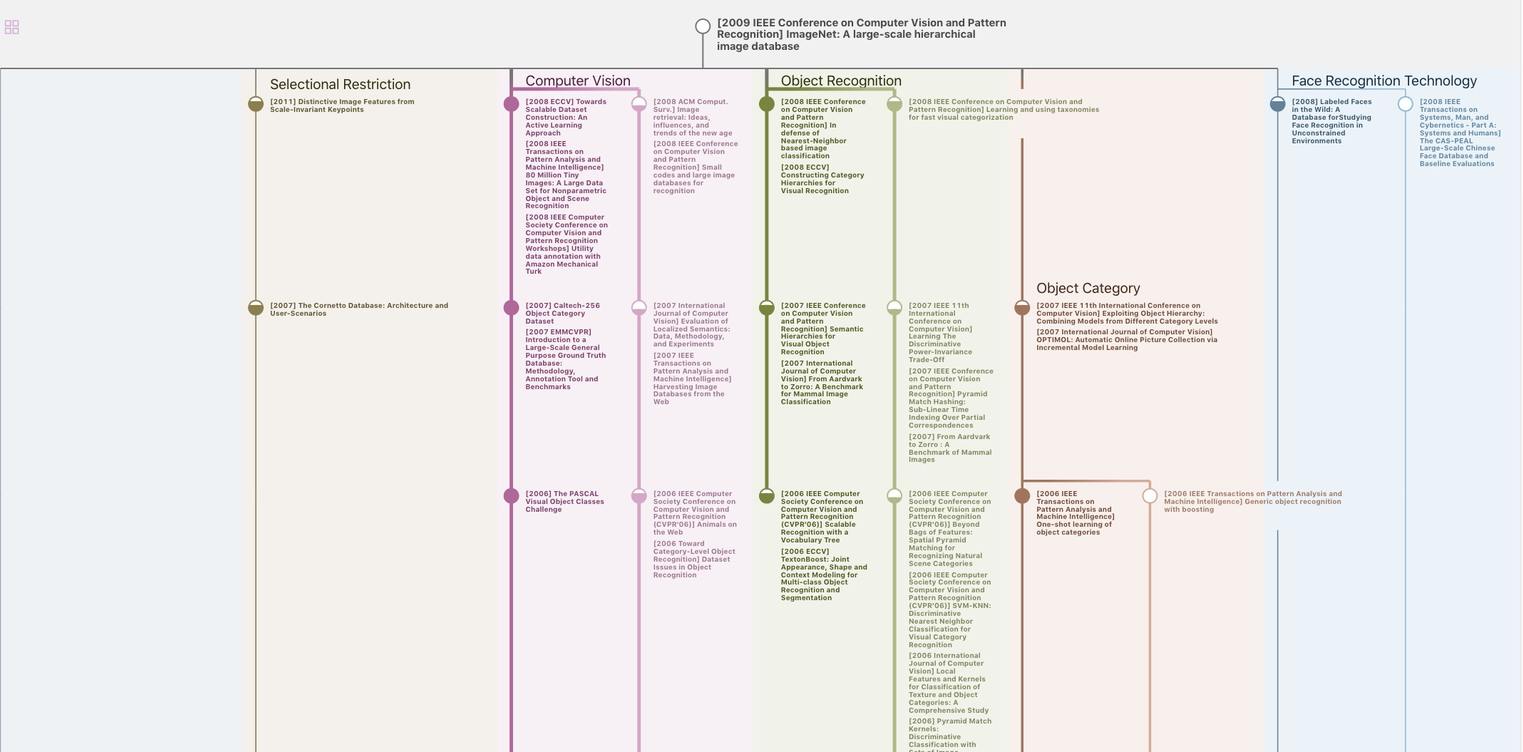
生成溯源树,研究论文发展脉络
Chat Paper
正在生成论文摘要