Delta-AI: Local objectives for amortized inference in sparse graphical models
arxiv(2023)
摘要
We present a new algorithm for amortized inference in sparse probabilistic
graphical models (PGMs), which we call Δ-amortized inference
(Δ-AI). Our approach is based on the observation that when the sampling
of variables in a PGM is seen as a sequence of actions taken by an agent,
sparsity of the PGM enables local credit assignment in the agent's policy
learning objective. This yields a local constraint that can be turned into a
local loss in the style of generative flow networks (GFlowNets) that enables
off-policy training but avoids the need to instantiate all the random variables
for each parameter update, thus speeding up training considerably. The
Δ-AI objective matches the conditional distribution of a variable given
its Markov blanket in a tractable learned sampler, which has the structure of a
Bayesian network, with the same conditional distribution under the target PGM.
As such, the trained sampler recovers marginals and conditional distributions
of interest and enables inference of partial subsets of variables. We
illustrate Δ-AI's effectiveness for sampling from synthetic PGMs and
training latent variable models with sparse factor structure.
更多查看译文
AI 理解论文
溯源树
样例
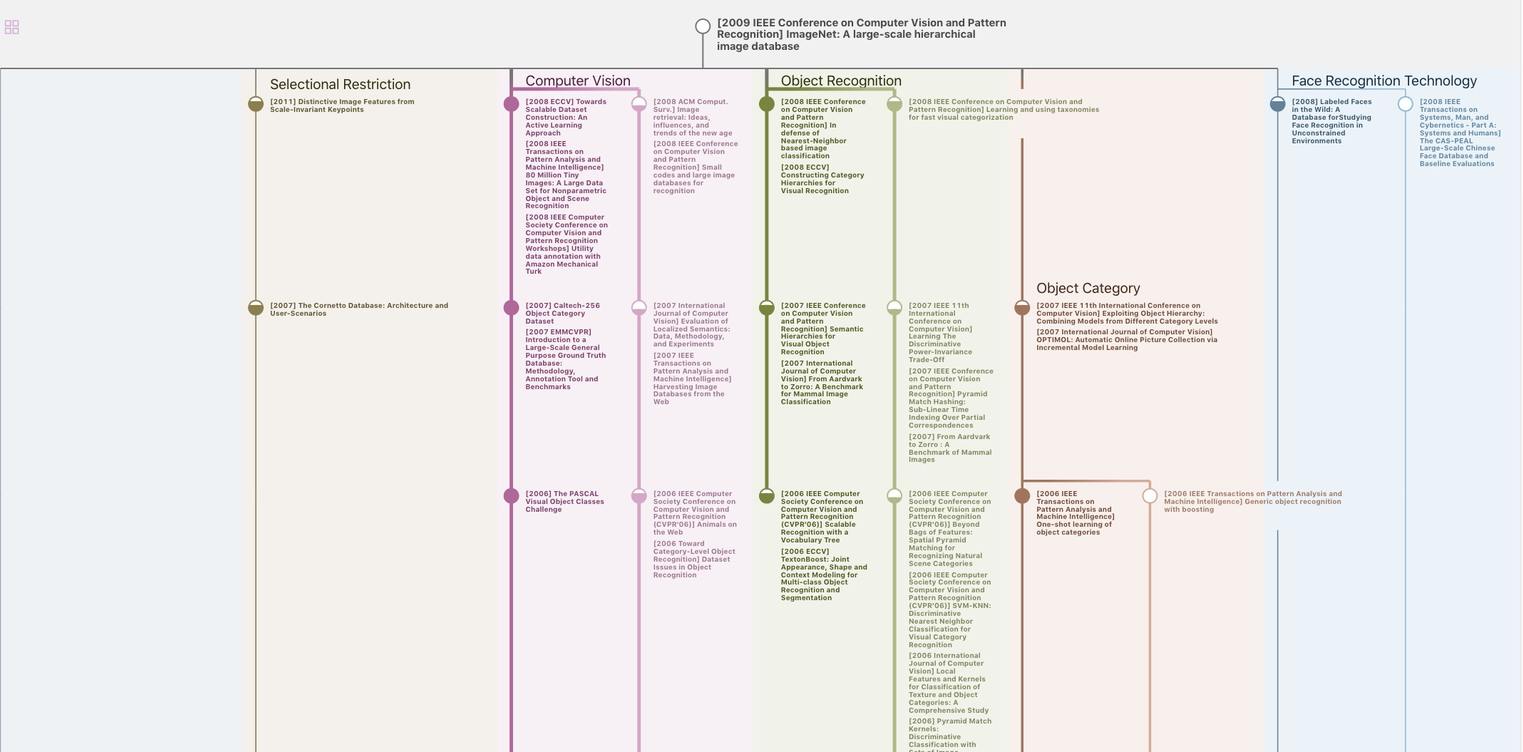
生成溯源树,研究论文发展脉络
Chat Paper
正在生成论文摘要