Deep Multi-Task Learning Based Fast Intra-Mode Decision for Versatile Video Coding
IEEE Transactions on Circuits and Systems for Video Technology(2023)
摘要
The latest Versatile Video Coding (VVC) standard has significantly coding efficiency improvement compared with its ancestor High Efficiency Video Coding (HEVC) standard, but at the expense of over-high complexity. As measured by the VVC test model (VTM), the intra-mode comparison and selection in the rate-distortion optimization (RDO) search consume most of the encoding time. In this paper, we propose a deep multi-task learning based fast intra-mode decision approach via adaptively pruning off most redundant modes. First, we create a large-scale intra-mode database for VVC, including both normal angular modes and the newly introduced tools, i.e., intra sub-partition (ISP) and matrix-based intra prediction (MIP). Next, we propose a multi-task intra-mode decision network (MID-Net) model to effectively predict the most probable angular modes and whether to skip ISP and MIP modes. Then, a fast intra-coding workflow is designed accordingly, involving rough mode decision (RMD) acceleration and candidate mode list (CML) pruning. For the workflow output, the learning-oriented probability and the statistics-oriented probability are synthesized together to further improve the prediction accuracy, ensuring that only unnecessary intra-modes are skipped. Finally, experimental results show that our approach can significantly reduce 40.48% of encoding time of VVC intra-coding with negligible rate-distortion degradation, outperforming other state-of-the-art approaches.
更多查看译文
关键词
Versatile video coding (VVC),deep multi-task learning,fast intra-mode decision,rate-distortion optimization
AI 理解论文
溯源树
样例
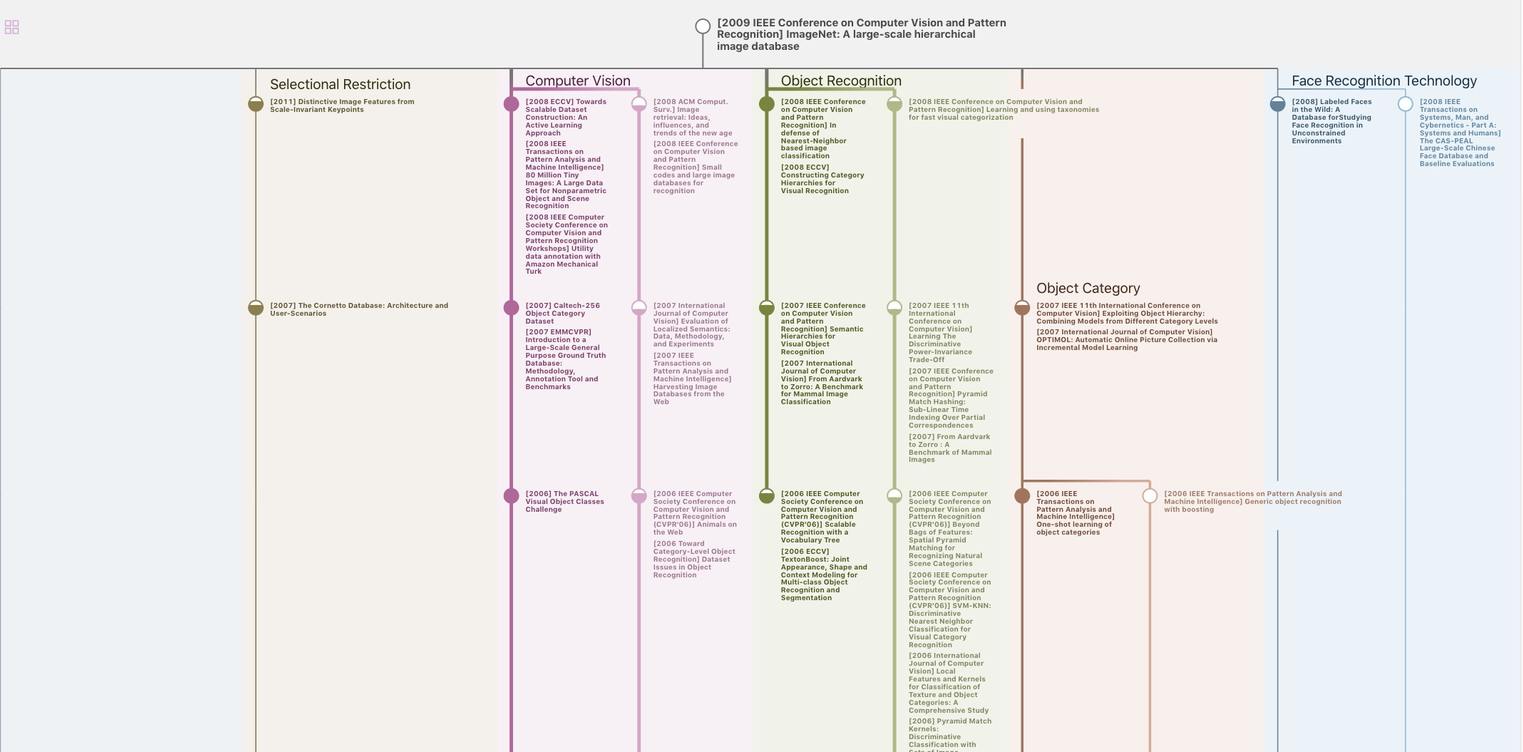
生成溯源树,研究论文发展脉络
Chat Paper
正在生成论文摘要