Bayes Filter-Based Occupancy Detection Using Networked SLEEPIR Sensors
IEEE Sensors Journal(2023)
摘要
We have previously developed a synchronized low-energy electronically chopped passive infrared (SLEEPIR) sensor node that can detect both stationary and moving occupants. In this article, we present a Bayes filter (BF)-based network-level algorithm that uses a network of SLEEPIR sensor nodes deployed at a residential apartment to estimate the occupancy of the entire apartment. The method processes the incoming observations from each of the sensor nodes via a sensor model and transforms these observations into Bayesian updates. The sensor model uses a Markov decision process (MDP) formulation to estimate the temporal bounds on the rate of occupancy flow between one occupancy state to another. The overall BF output is a probability density function (pdf) that represents the occupancy state of the entire observed space. The sensor node adjacency matrix and observation frequency are the key parameters that contribute to the sensor model design. The sensor model uses estimated transition time and probability between occupancy states to filter out observations that do not conform to the constraints set forth by the parameters. Occupancy is established through a thresholding function applied to the output pdf of the BF. A dataset was collected at a residential unit over a period of one month using the SLEEPIR sensor system. Results indicate an average 23.68% occupancy accuracy improvement when compared to the accuracy state delivered by individual SLEEPIR nodes. Results also indicate a 7.74% occupancy accuracy improvement when compared to the accuracy state determined by an already proposed particle filter (PF)-based occupancy estimation algorithm.
更多查看译文
关键词
occupancy detection,sensors,filter-based
AI 理解论文
溯源树
样例
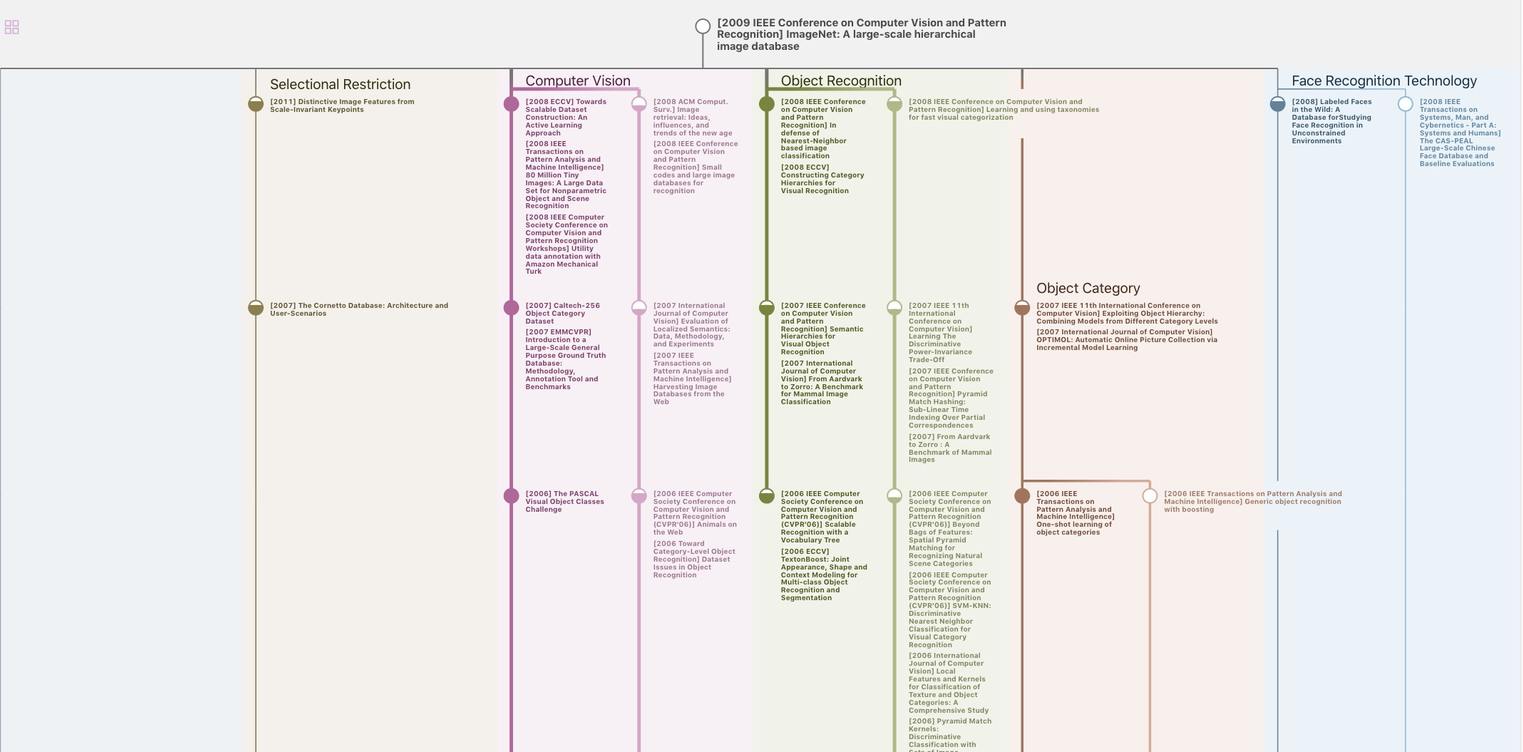
生成溯源树,研究论文发展脉络
Chat Paper
正在生成论文摘要