Comparison of Radiologists and Deep Learning for US Grading of Hepatic Steatosis
RADIOLOGY(2023)
摘要
Background: Screening for nonalcoholic fatty liver disease (NAFLD) is suboptimal due to the subjective interpretation of US images. Purpose: To evaluate the agreement and diagnostic performance of radiologists and a deep learning model in grading hepatic steatosis in NAFLD at US, with biopsy as the reference standard. Materials and Methods: This retrospective study included patients with NAFLD and control patients without hepatic steatosis who underwent abdominal US and contemporaneous liver biopsy from September 2010 to October 2019. Six readers visually graded steatosis on US images twice, 2 weeks apart. Reader agreement was assessed with use of kappa statistics. Three deep learning techniques applied to B-mode US images were used to classify dichotomized steatosis grades. Classification performance of human radiologists and the deep learning model for dichotomized steatosis grades (S0, S1, S2, and S3) was assessed with area under the receiver operating characteristic curve (AUC) on a separate test set. Results: The study included 199 patients (mean age, 53 years +/- 13 [SD]; 101 men). On the test set (n = 52), radiologists had fair interreader agreement (0.34 [95% CI: 0.31, 0.37]) for classifying steatosis grades S0 versus S1 or higher, while AUCs were between 0.49 and 0.84 for radiologists and 0.85 (95% CI: 0.83, 0.87) for the deep learning model. For S0 or S1 versus S2 or S3, radiologists had fair interreader agreement (0.30 [95% CI: 0.27, 0.33]), while AUCs were between 0.57 and 0.76 for radiologists and 0.73 (95% CI: 0.71, 0.75) for the deep learning model. For S2 or lower versus S3, radiologists had fair interreader agreement (0.37 [95% CI: 0.33, 0.40]), while AUCs were between 0.52 and 0.81 for radiologists and 0.67 (95% CI: 0.64, 0.69) for the deep learning model. Conclusion: Deep learning approaches applied to B-mode US images provided comparable performance with human readers for detection and grading of hepatic steatosis.
更多查看译文
AI 理解论文
溯源树
样例
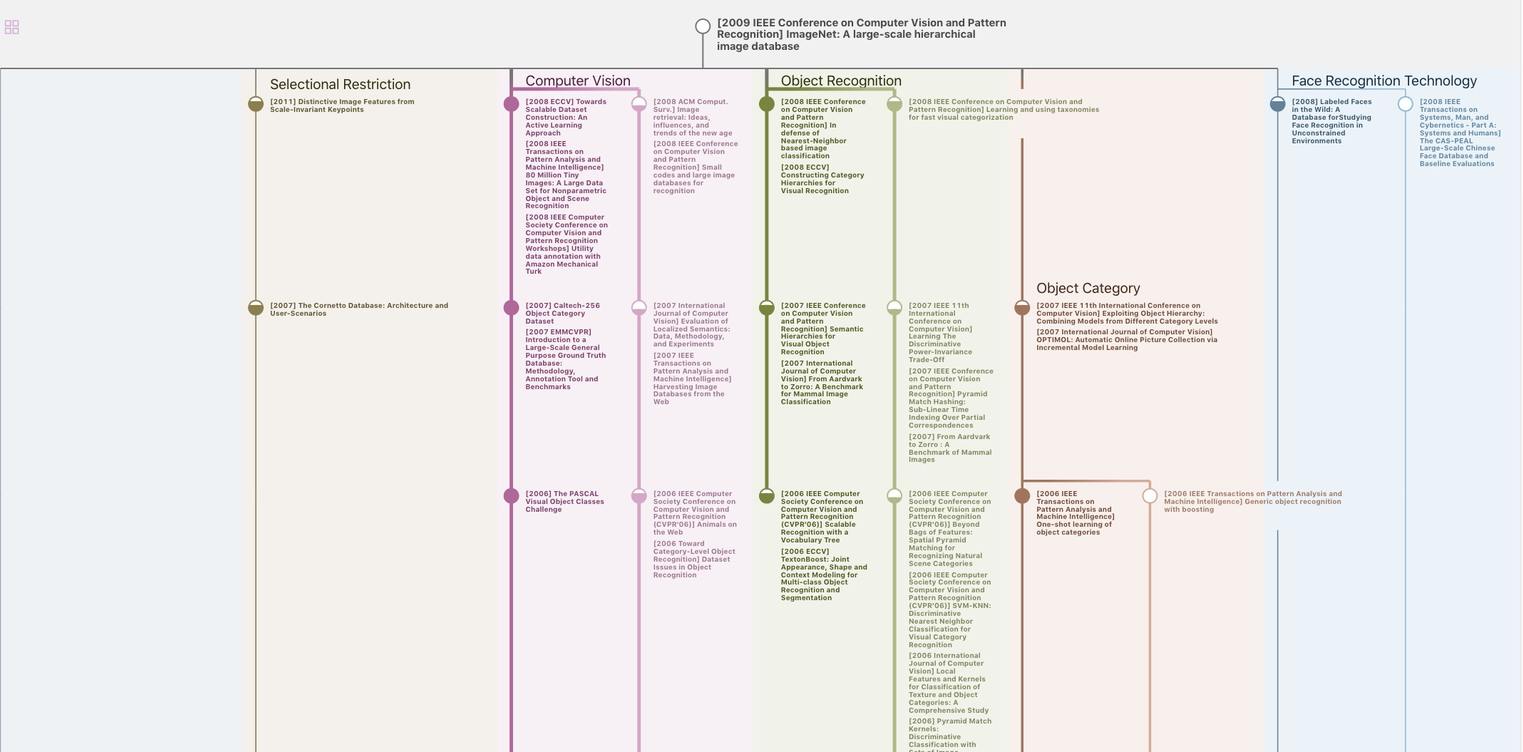
生成溯源树,研究论文发展脉络
Chat Paper
正在生成论文摘要