FLEDGE: Ledger-based Federated Learning Resilient to Inference and Backdoor Attacks
39TH ANNUAL COMPUTER SECURITY APPLICATIONS CONFERENCE, ACSAC 2023(2023)
摘要
Federated learning (FL) is a distributed learning process that uses a trusted aggregation server to allow multiple parties (or clients) to collaboratively train a machine learning model without having them share their private data. Recent research, however, has demonstrated the effectiveness of inference and poisoning attacks on FL. Mitigating both attacks simultaneously is very challenging. Stateof-the-art solutions have proposed the use of poisoning defenses with Secure Multi-Party Computation (SMPC) and/or Differential Privacy (DP). However, these techniques are not efficient and fail to address the malicious intent behind the attacks, i.e., adversaries (curious servers and/or compromised clients) seek to exploit a system for monetization purposes. To overcome these limitations, we present a ledger-based FL framework known as FLEDGE that allows making parties accountable for their behavior and achieve reasonable efficiency for mitigating inference and poisoning attacks. Our solution leverages crypto-currency to increase party accountability by penalizing malicious behavior and rewarding benign conduct. We conduct an extensive evaluation on four public datasets: Reddit, MNIST, Fashion-MNIST, and CIFAR-10. Our experimental results demonstrate that (1) FLEDGE provides strong privacy guarantees for model updates without sacrificing model utility; (2) FLEDGE can successfully mitigate different poisoning attacks without degrading the performance of the global model; and (3) FLEDGE offers unique reward mechanisms to promote benign behavior during model training and/or model aggregation.
更多查看译文
关键词
blockchain,federated learning,homomorphic encryption,security and privacy
AI 理解论文
溯源树
样例
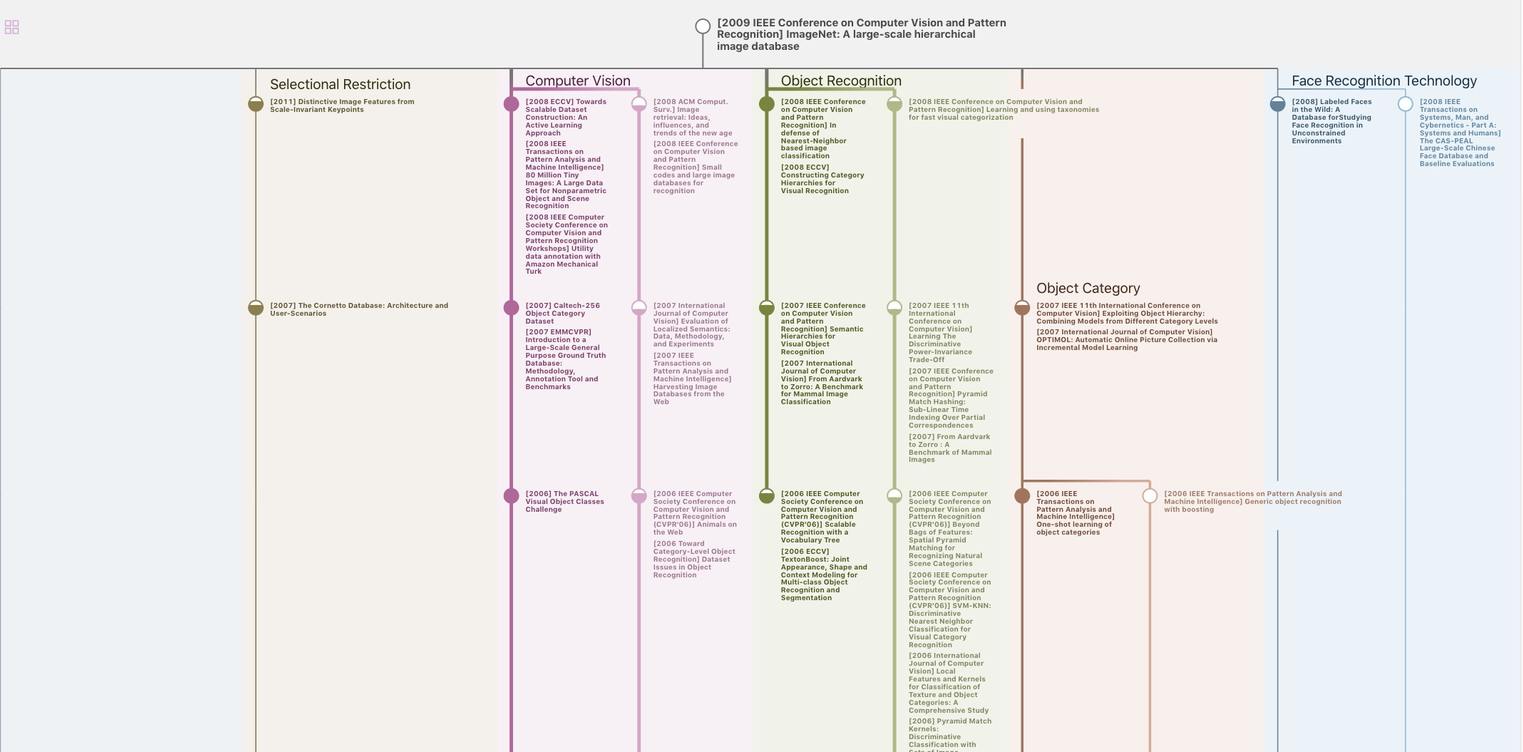
生成溯源树,研究论文发展脉络
Chat Paper
正在生成论文摘要