De Novo Drug Design with Joint Transformers
CoRR(2023)
摘要
De novo drug design requires simultaneously generating novel molecules outside of training data and predicting their target properties, making it a hard task for generative models. To address this, we propose Joint Transformer that combines a Transformer decoder, a Transformer encoder, and a predictor in a joint generative model with shared weights. We show that training the model with a penalized log-likelihood objective results in state-of-the-art performance in molecule generation, while decreasing the prediction error on newly sampled molecules, as compared to a fine-tuned decoder-only Transformer, by 42%. Finally, we propose a probabilistic black-box optimization algorithm that employs Joint Transformer to generate novel molecules with improved target properties, as compared to the training data, outperforming other SMILES-based optimization methods in de novo drug design.
更多查看译文
关键词
joint transformers,drug
AI 理解论文
溯源树
样例
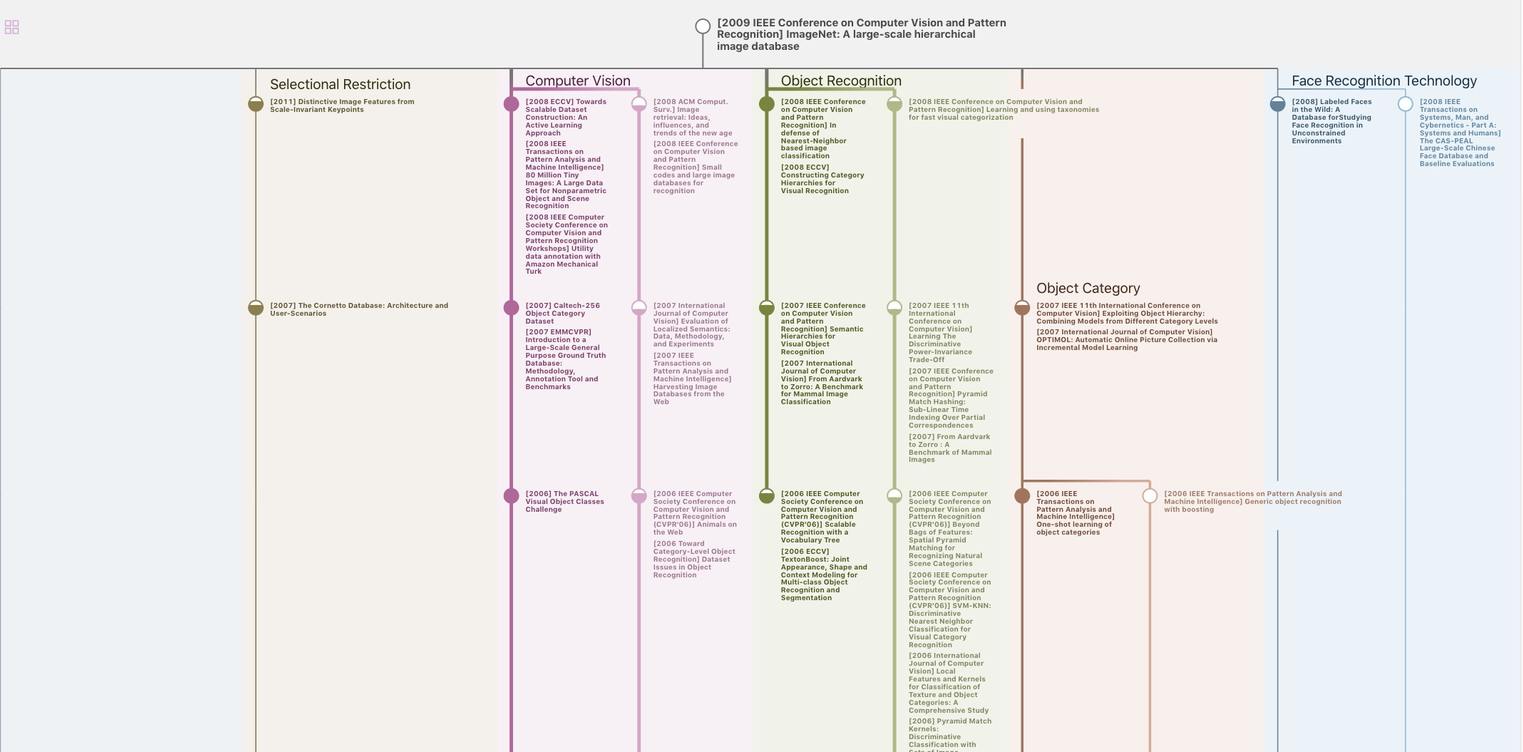
生成溯源树,研究论文发展脉络
Chat Paper
正在生成论文摘要