RoFormer for Position Aware Multiple Instance Learning in Whole Slide Image Classification
MACHINE LEARNING IN MEDICAL IMAGING, MLMI 2023, PT II(2024)
摘要
Whole slide image (WSI) classification is a critical task in computational pathology. However, the gigapixel-size of such images remains a major challenge for the current state of deep-learning. Current methods rely on multiple-instance learning (MIL) models with frozen feature extractors. Given the the high number of instances in each image, MIL methods have long assumed independence and permutation-invariance of patches, disregarding the tissue structure and correlation between patches. Recent works started studying this correlation between instances but the computational workload of such a high number of tokens remained a limiting factor. In particular, relative position of patches remains unaddressed. We propose to apply a straightforward encoding module, namely a RoFormer layer, relying onmemory-efficient exact self-attention and relative positional encoding. This module can perform full self-attention with relative position encoding on patches of large and arbitrary shapedWSIs, solving the need for correlation between instances and spatial modeling of tissues. We demonstrate that our method outperforms state-of-the-art MIL models on three commonly used public datasets (TCGA-NSCLC, BRACS and Camelyon16)) on weakly supervised classification tasks. Code is available at https://github.com/Sanofi-Public/DDS-RoFor merMIL.
更多查看译文
关键词
whole slide
AI 理解论文
溯源树
样例
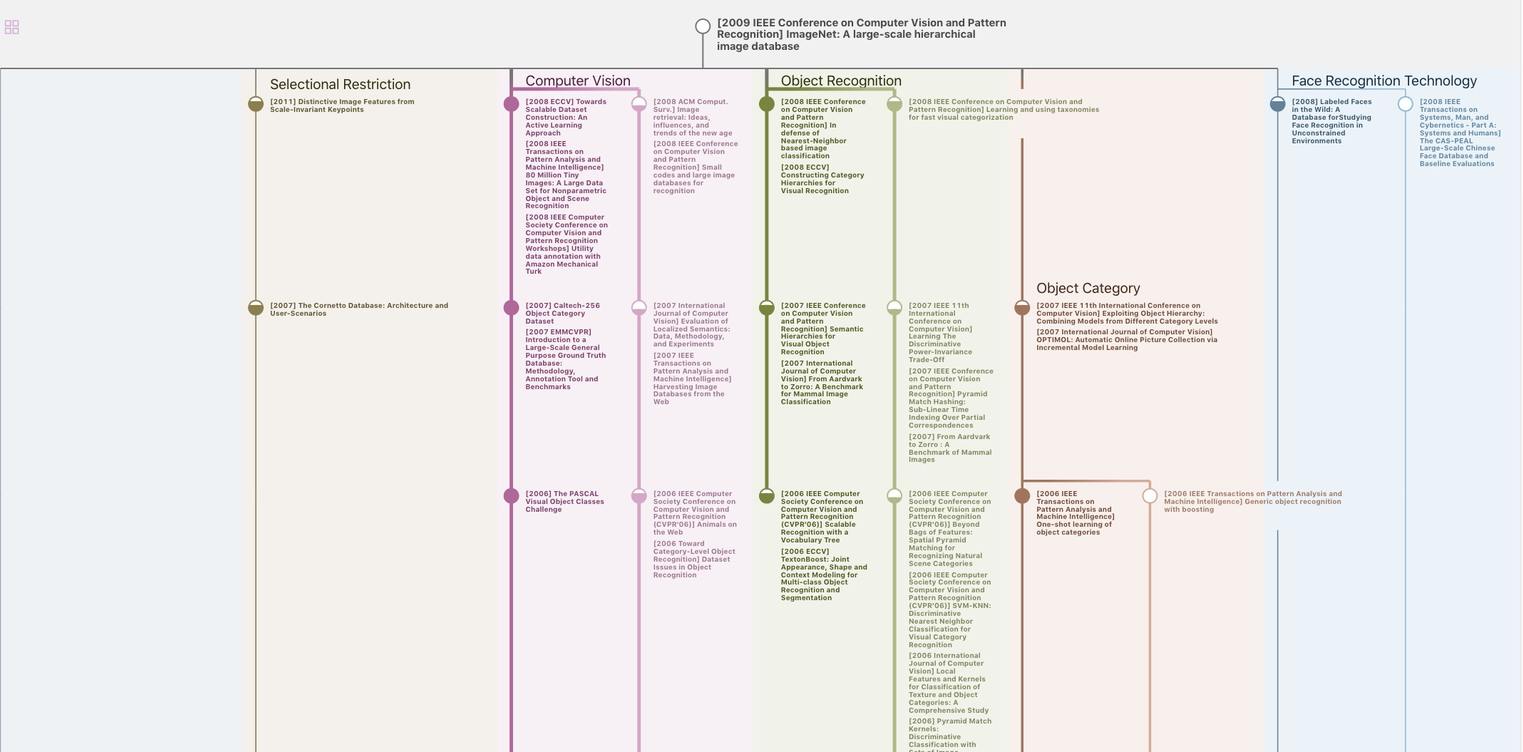
生成溯源树,研究论文发展脉络
Chat Paper
正在生成论文摘要