On Squared-Variable Formulations
arxiv(2023)
摘要
We revisit a formulation technique for inequality constrained optimization problems that has been known for decades: the substitution of squared variables for nonnegative variables. Using this technique, inequality constraints are converted to equality constraints via the introduction of a squared-slack variable. Such formulations have the superficial advantage that inequality constraints can be dispensed with altogether. But there are clear disadvantages, not least being that first-order optimal points for the squared-variable reformulation may not correspond to first-order optimal points for the original problem, because the Lagrange multipliers may have the wrong sign. Extending previous results, this paper shows that points satisfying approximate second-order optimality conditions for the squared-variable reformulation also, under certain conditions, satisfy approximate second-order optimality conditions for the original formulation, and vice versa. Such results allow us to adapt complexity analysis of methods for equality constrained optimization to account for inequality constraints. On the algorithmic side, we examine squared-variable formulations for several interesting problem classes, including bound-constrained quadratic programming and linear programming. We show that algorithms built on these formulations are surprisingly competitive with standard methods. For linear programming, we examine the relationship between the squared-variable approach and primal-dual interior-point methods.
更多查看译文
AI 理解论文
溯源树
样例
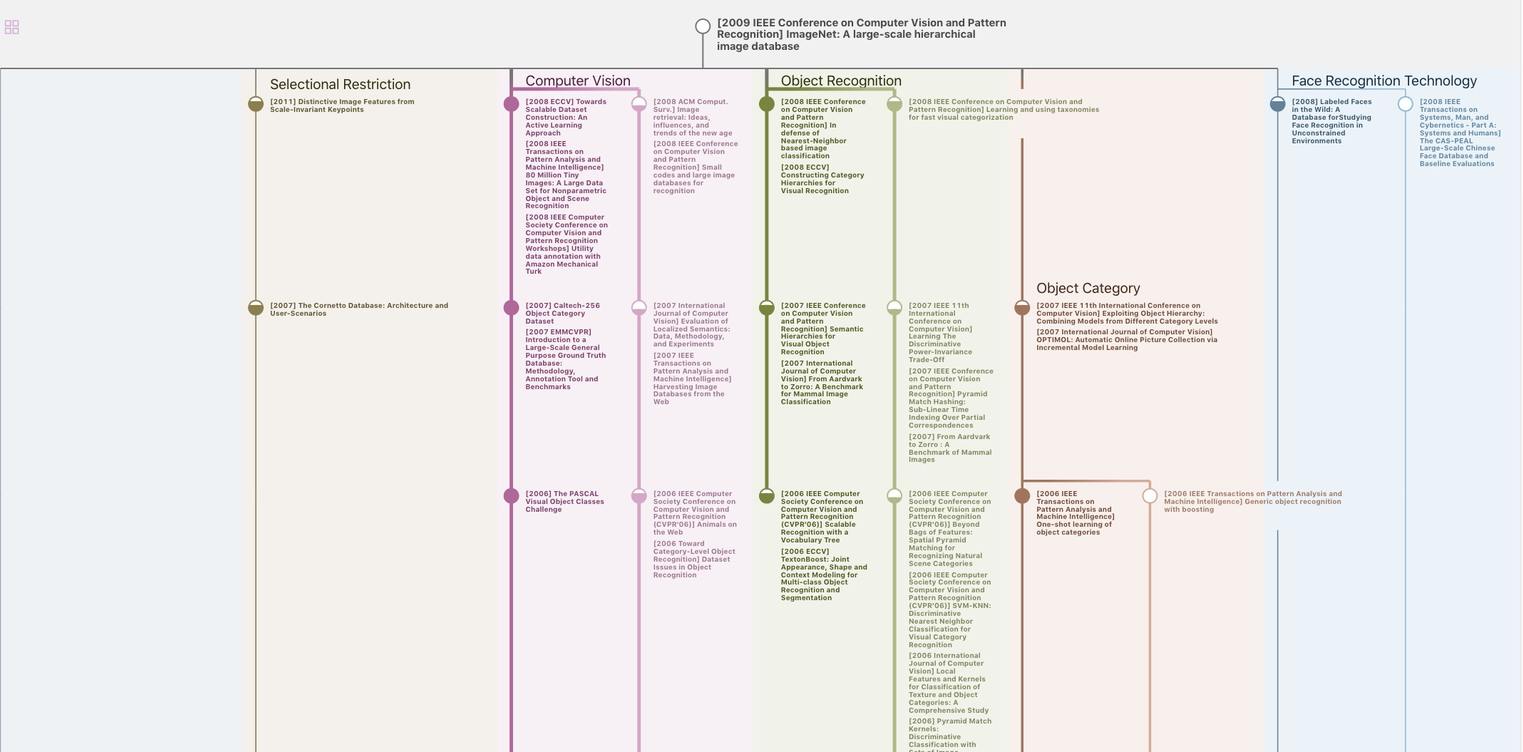
生成溯源树,研究论文发展脉络
Chat Paper
正在生成论文摘要