PreCog: Near-Storage Accelerator for Heterogeneous CNN Inference
2023 IEEE 34th International Conference on Application-specific Systems, Architectures and Processors (ASAP)(2023)
摘要
Computational Storage Devices (CSD) with near-storage acceleration is gaining popularity for data-intensive applications, by moving power-efficient hardware acceleration closer to data. However, because the power-constrained near-storage accelerator is often not powerful enough by itself to handle all computation requirements of an application, it must intelligently cooperate with other computation and acceleration units in the system. In this work, we explore how a near-storage accelerator can best fit into a larger computer system in the context of CNN inference. We demonstrate that an attractive configuration is using the near-storage accelerator to offload only the first convolution and pooling layers, where the accelerator can achieve almost an order of magnitude better performance compared to a general convolution accelerator. Targeting only the first layer allows some FPGA-specific floating-point computation optimizations such as pre-determine the range of output exponents, performing a costly floating point normalization task only once, as well as pack more input into the datapath to mitigate the performance impact of wide strides of the convolution filter. We package these optimizations into a flexible library we call Static Range Float (SRFloat), and construct a prototype system called PreCog. We evaluate PreCog implemented on the Samsung SmartSSD platform, and demonstrate over
$\mathbf{3}\times$
performance efficiency compared to conventional convolution accelerators on prominent CNN models without introducing a communications bottleneck.
更多查看译文
AI 理解论文
溯源树
样例
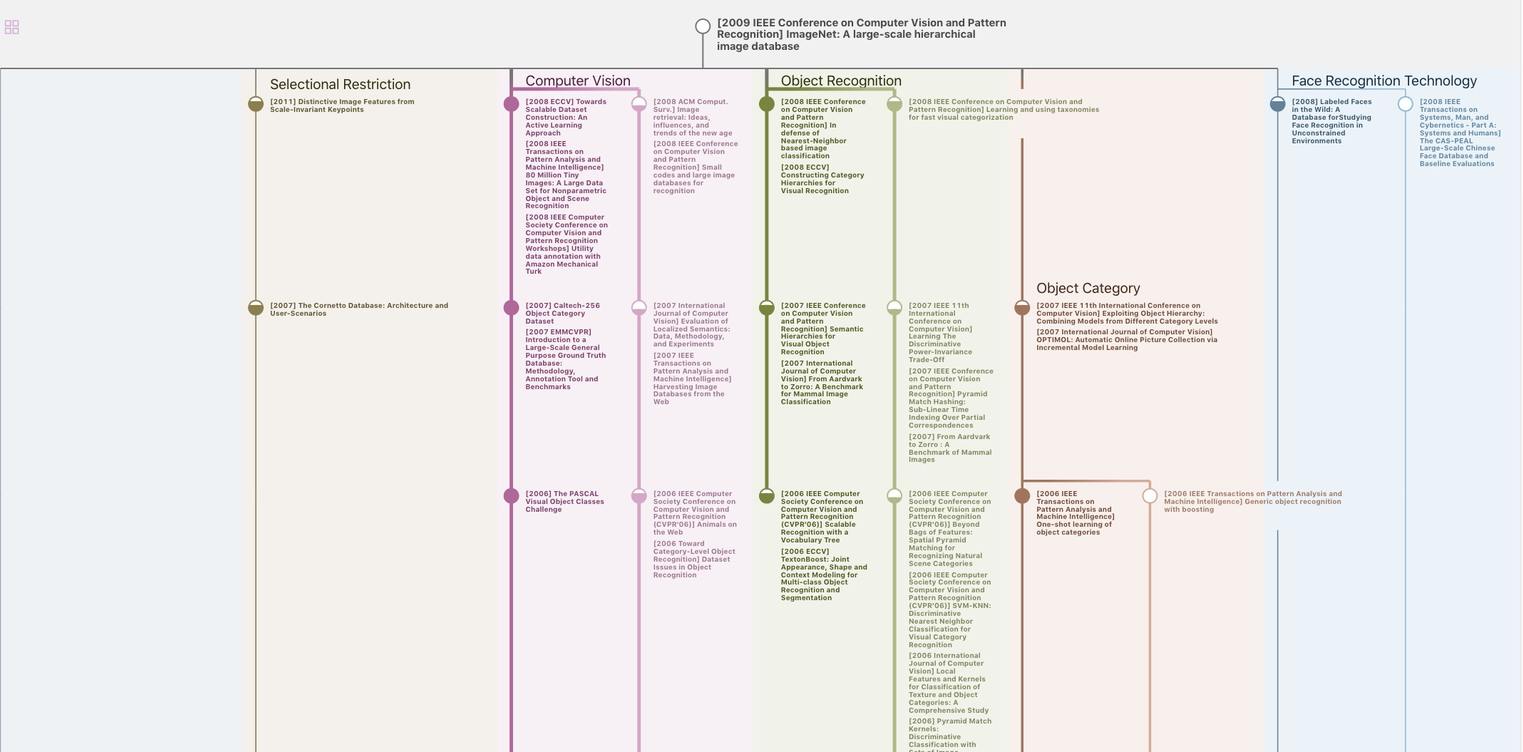
生成溯源树,研究论文发展脉络
Chat Paper
正在生成论文摘要