Emergent Communication in Multi-Agent Reinforcement Learning for Flying Base Stations
2023 IEEE International Mediterranean Conference on Communications and Networking (MeditCom)(2023)
摘要
In order to increase network capacity and coverage, flying base stations (FBSs) can be deployed in a variety of scenarios, such as in extremely crowded gatherings or for emergency communication and network access in areas without terrestrial network coverage. Due to their inherent low cost, ease of deployment and high mobility, unmanned aerial vehicles (UAVs) deployed as FBSs can provide cost-effective, fast and reliable network access services to remote ground users. To maximize network capacity, FBSs need to coordinate and exchange information about their observations to optimize their positions, under limited energy and bandwidth resources. In this paper, we investigate the problem of optimizing the positions of FBSs using the framework of emerging communications in multi-agent reinforcement learning (EC-MARL) and we evaluate two EC-MARL architectures, namely Multi-Agent Graph-Attention Communication and Teaming (MAGIC) and Targeted Multi-Agent Communication (TarMAC). We show that coordination between FBSs through learning a communication protocol increases the total achievable rate and coverage of ground users, compared to baselines without communication. Moreover, we consider challenging environments with a large number of FBSs and demonstrate the efficiency of the proposed method in terms of speed of convergence and robustness to the movement of users.
更多查看译文
关键词
Multi-agent reinforcement learning,Emergent communication,Flying base station,UAV
AI 理解论文
溯源树
样例
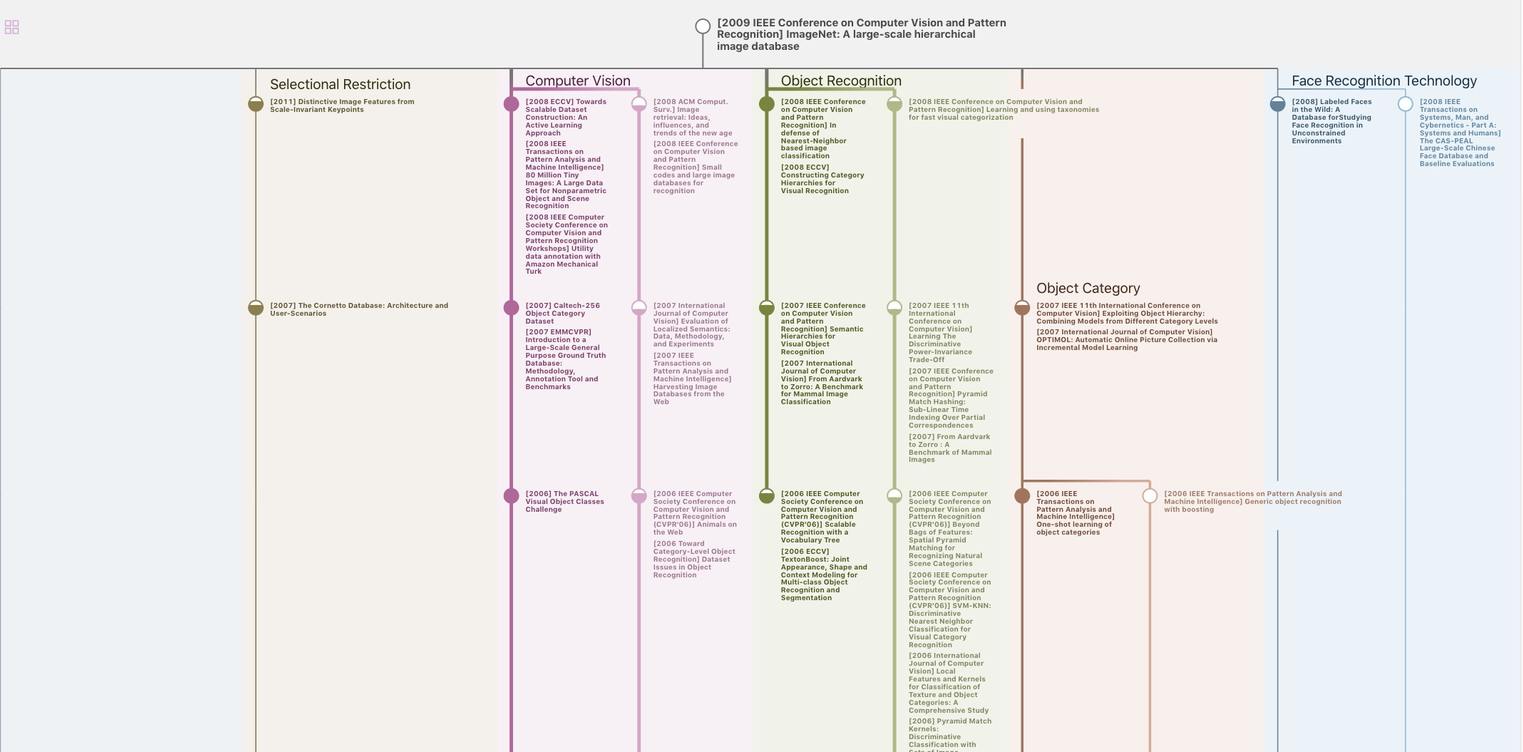
生成溯源树,研究论文发展脉络
Chat Paper
正在生成论文摘要