Hybridization of Evolutionary Algorithm and Deep Reinforcement Learning for Multiobjective Orienteering Optimization
IEEE TRANSACTIONS ON EVOLUTIONARY COMPUTATION(2023)
摘要
Multiobjective orienteering problems (MO-OPs) are classical multiobjective routing problems and have received much attention in recent decades. This study seeks to solve MO-OPs through a problem-decomposition framework, that is, an MO-OP is decomposed into a multiobjective knapsack problem (MOKP) and a traveling salesman problem (TSP). The MOKP and TSP are then solved by a multiobjective evolutionary algorithm (MOEA) and a deep reinforcement learning (DRL) method, respectively. While the MOEA module is for selecting cities, the DRL module is for planning a Hamiltonian path for these cities. An iterative use of these two modules drives the population toward the Pareto front of MO-OPs. The effectiveness of the proposed method is compared against NSGA-II and NSGA-III on various types of MO-OP instances. Experimental results show that our method performs best on almost all the test instances and has shown strong generalization ability.
更多查看译文
关键词
Decomposition,deep reinforcement learning (DRL),evolutionary algorithms (EAs),multiobjective optimization,orienteering problems (OPs),pointer networks (PNs)
AI 理解论文
溯源树
样例
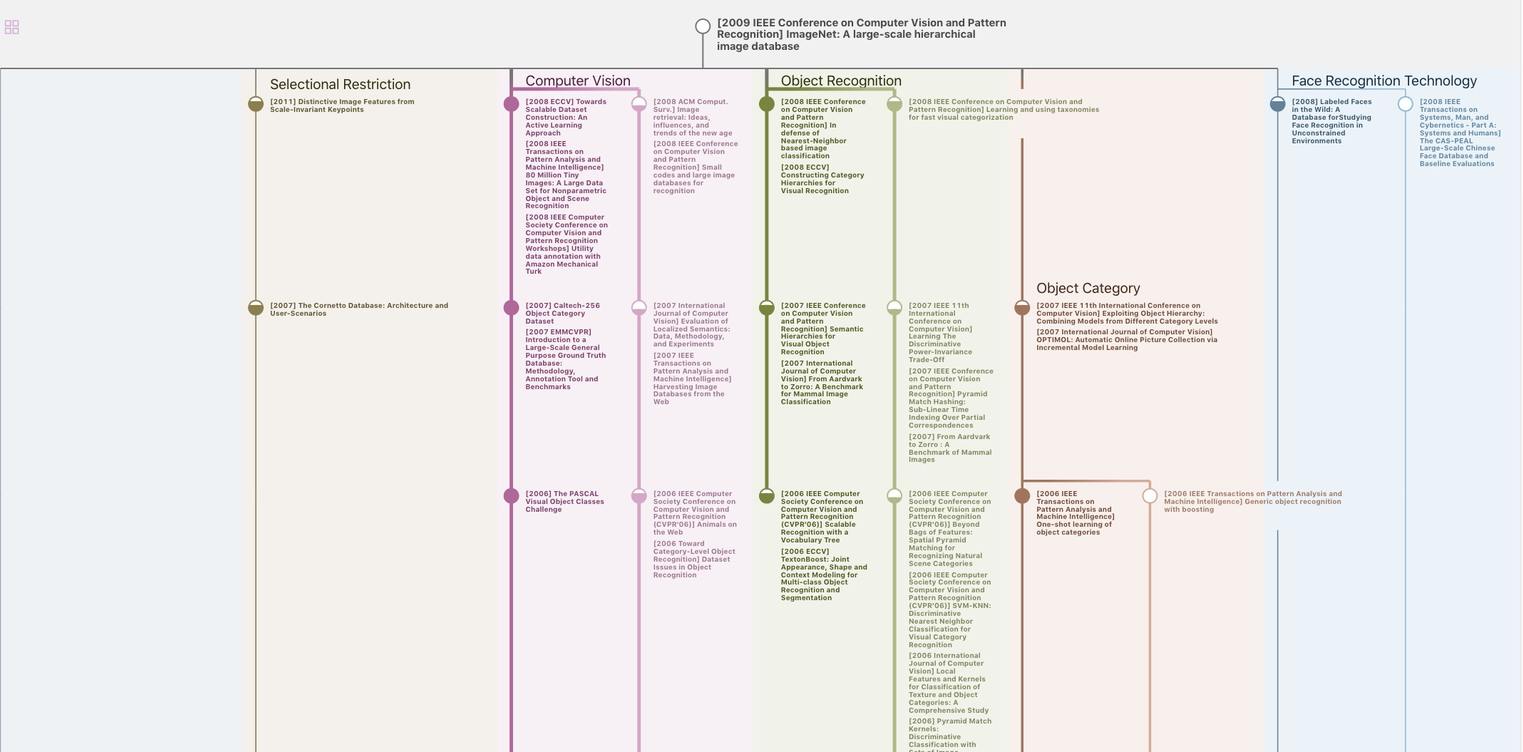
生成溯源树,研究论文发展脉络
Chat Paper
正在生成论文摘要