Joint localization and classification of breast masses on ultrasound images using an auxiliary attention-based framework.
Medical image analysis(2023)
摘要
Multi-task learning (MTL) methods have been extensively employed for joint localization and classification of breast lesions on ultrasound images to assist in cancer diagnosis and personalized treatment. One typical paradigm in MTL is a shared trunk network architecture. However, such a model design may suffer information-sharing conflicts and only achieve suboptimal performance for individual tasks. Additionally, the model relies on fully-supervised learning methodologies, imposing heavy burdens on data annotation. In this study, we propose a novel joint localization and classification model based on attention mechanisms and a sequential semi-supervised learning strategy to address these challenges. Our proposed framework offers three primary advantages. First, a lesion-aware network with multiple attention modules is designed to improve model performance on lesion localization. An attention-based classifier explicitly establishes correlations between the two tasks, alleviating information-sharing conflicts while leveraging location information to assist in classification. Second, a two-stage sequential semi-supervised learning strategy is designed for model training to achieve optimal performance on both tasks and substantially reduces the need for data annotation. Third, the asymmetric and modular model architecture allows for the flexible interchangeability of individual components, rendering the model adaptable to various applications. Experimental results from two different breast ultrasound image datasets under varied conditions have demonstrated the effectiveness of the proposed method. Furthermore, we conduct comprehensive investigations into the impacts of various factors on model performance, gaining in-depth insights into the mechanism of our proposed framework. The code is available at https://github.com/comp-imaging-sci/lanet-bus.git.
更多查看译文
AI 理解论文
溯源树
样例
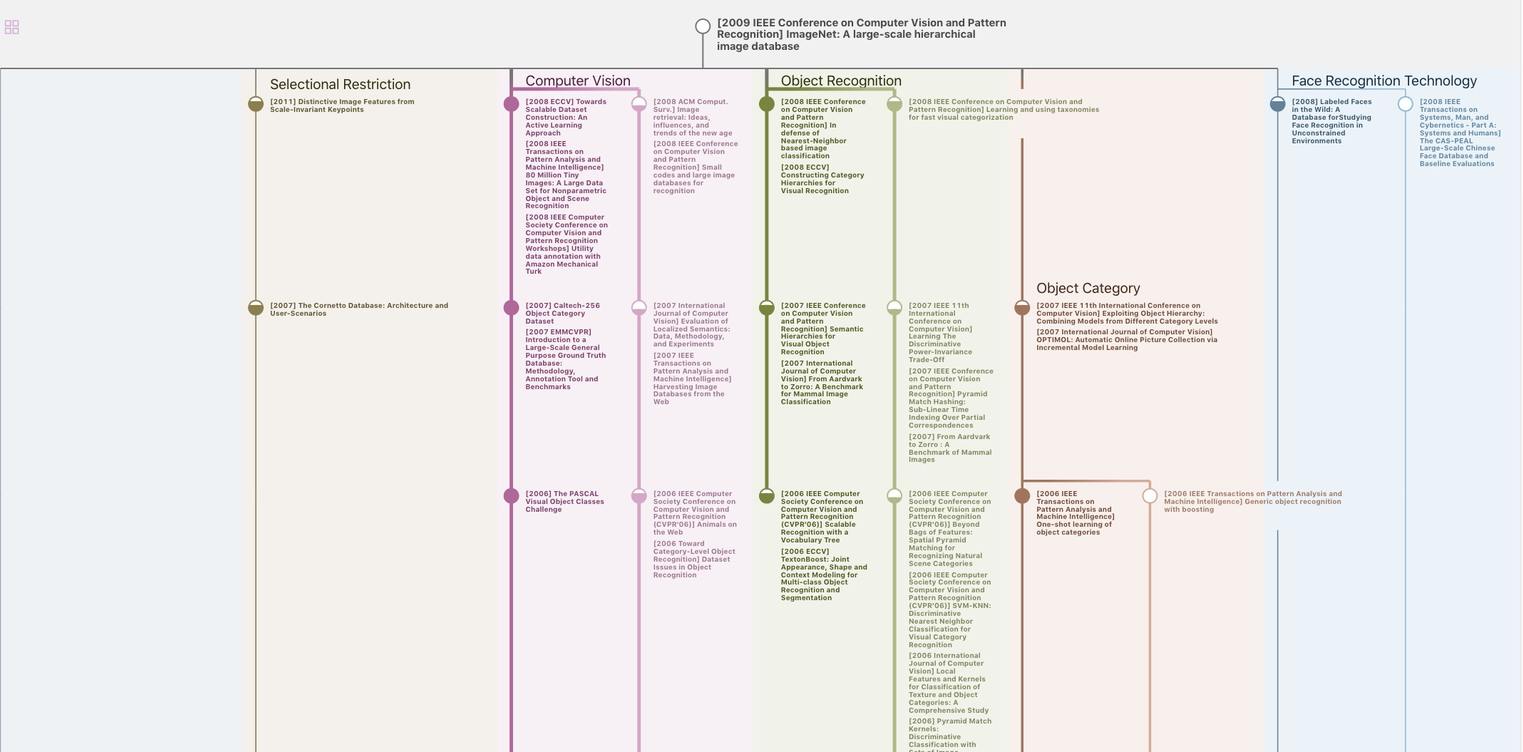
生成溯源树,研究论文发展脉络
Chat Paper
正在生成论文摘要