TADIS: Steering Models for Deep-Thinking about Demonstration Examples
CoRR(2023)
摘要
Instruction tuning has been demonstrated that could significantly improve the zero-shot generalization capability to unseen tasks by an apparent margin. By incorporating additional context (e.g., task definition, examples) during the fine-tuning process, Large Language Models (LLMs) achieved much higher performance than before. However, recent work reported that delusive task examples can achieve almost the same performance as correct task examples, indicating the input-label correspondence is less important than previously thought. Intrigued by this counter-intuitive observation, we suspect models have the same illusion of competence as humans. Therefore, we propose a novel method called TADIS that steers LLMs for "Deep-Thinking'' about demonstration examples instead of merely seeing. To alleviate the illusion of competence of models, we first ask the model to verify the correctness of shown examples. Then, using the verification results as conditions to elicit models for a better answer. Our experimental results show that TADIS consistently outperforms competitive baselines on in-domain and out-domain tasks (improving 2.79 and 4.03 average ROUGLE-L on out-domain and in-domain datasets, respectively). Despite the presence of generated examples (not all of the thinking labels are accurate), TADIS can notably enhance performance in zero-shot and few-shot settings. This also suggests that our approach can be adopted on a large scale to improve the instruction following capabilities of models without any manual labor. Moreover, we construct three types of thinking labels with different model sizes and find that small models learn from the format of TADIS but larger models can be steered for "Deep-Thinking''.
更多查看译文
关键词
examples,steering models,deep-thinking
AI 理解论文
溯源树
样例
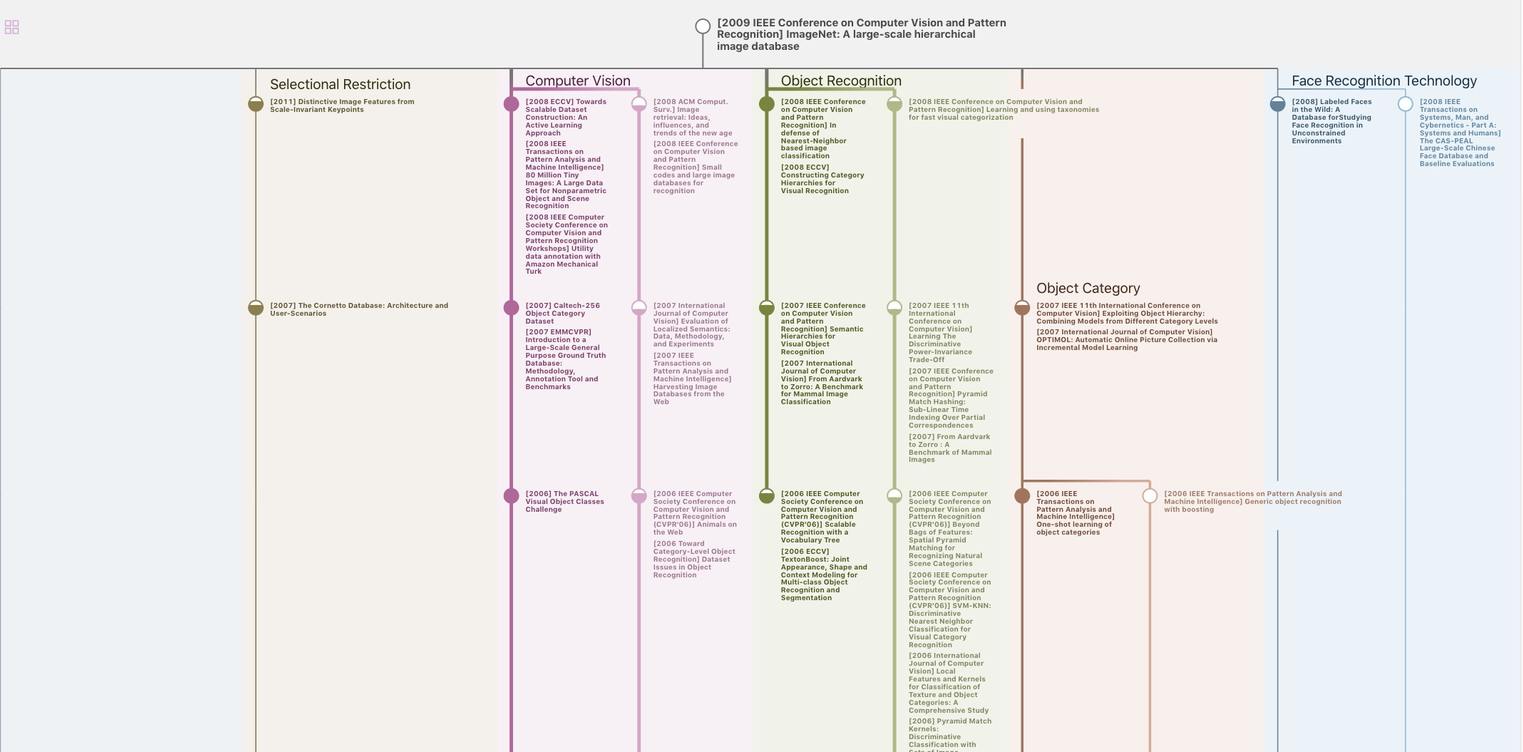
生成溯源树,研究论文发展脉络
Chat Paper
正在生成论文摘要