ReAcTable: Enhancing ReAct for Table Question Answering
CoRR(2023)
摘要
Table Question Answering (TQA) presents a substantial challenge at the intersection of natural language processing and data analytics. This task involves answering natural language (NL) questions on top of tabular data, demanding proficiency in logical reasoning, understanding of data semantics, and fundamental analytical capabilities. Due to its significance, a substantial volume of research has been dedicated to exploring a wide range of strategies aimed at tackling this challenge including approaches that leverage Large Language Models (LLMs) through in-context learning or Chain-of-Thought (CoT) prompting as well as approaches that train and fine-tune custom models. Nonetheless, a conspicuous gap exists in the research landscape, where there is limited exploration of how innovative foundational research, which integrates incremental reasoning with external tools in the context of LLMs, as exemplified by the ReAct paradigm, could potentially bring advantages to the TQA task. In this paper, we aim to fill this gap, by introducing ReAcTable (ReAct for Table Question Answering tasks), a framework inspired by the ReAct paradigm that is carefully enhanced to address the challenges uniquely appearing in TQA tasks such as interpreting complex data semantics, dealing with errors generated by inconsistent data and generating intricate data transformations. ReAcTable relies on external tools such as SQL and Python code executors, to progressively enhance the data by generating intermediate data representations, ultimately transforming it into a more accessible format for answering the questions with greater ease. We demonstrate that ReAcTable achieves remarkable performance even when compared to fine-tuned approaches. In particular, it outperforms the best prior result on the WikiTQ benchmark, achieving an accuracy of 68.0% without requiring training a new model or fine-tuning.
更多查看译文
关键词
reactable
AI 理解论文
溯源树
样例
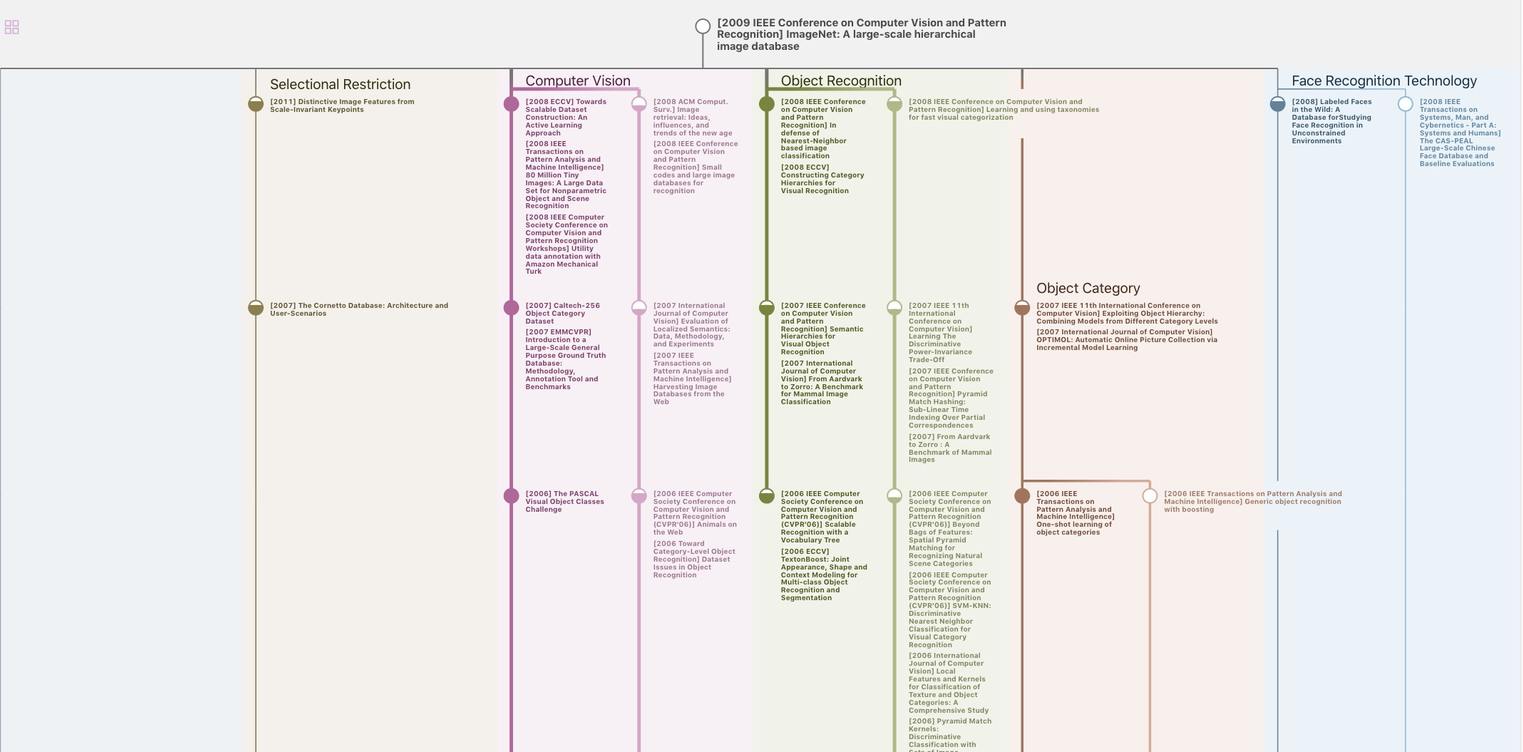
生成溯源树,研究论文发展脉络
Chat Paper
正在生成论文摘要