A Task-oriented Dialog Model with Task-progressive and Policy-aware Pre-training
NLPCC (1)(2023)
摘要
Pre-trained conversation models (PCMs) have achieved promising progress in recent years. However, existing PCMs for Task-oriented dialog (TOD) are insufficient for capturing the sequential nature of the TOD-related tasks, as well as for learning dialog policy information. To alleviate these problems, this paper proposes a task-progressive PCM with two policy-aware pre-training tasks. The model is pre-trained through three stages where TOD-related tasks are progressively employed according to the task logic of the TOD system. A global policy consistency task is designed to capture the multi-turn dialog policy sequential relation, and an act-based contrastive learning task is designed to capture similarities among samples with the same dialog policy. Our model achieves better results on both MultiWOZ and In-Car end-to-end dialog modeling benchmarks with only 18\% parameters and 25\% pre-training data compared to the previous state-of-the-art PCM, GALAXY.
更多查看译文
关键词
dialog model,task-oriented,task-progressive,policy-aware,pre-training
AI 理解论文
溯源树
样例
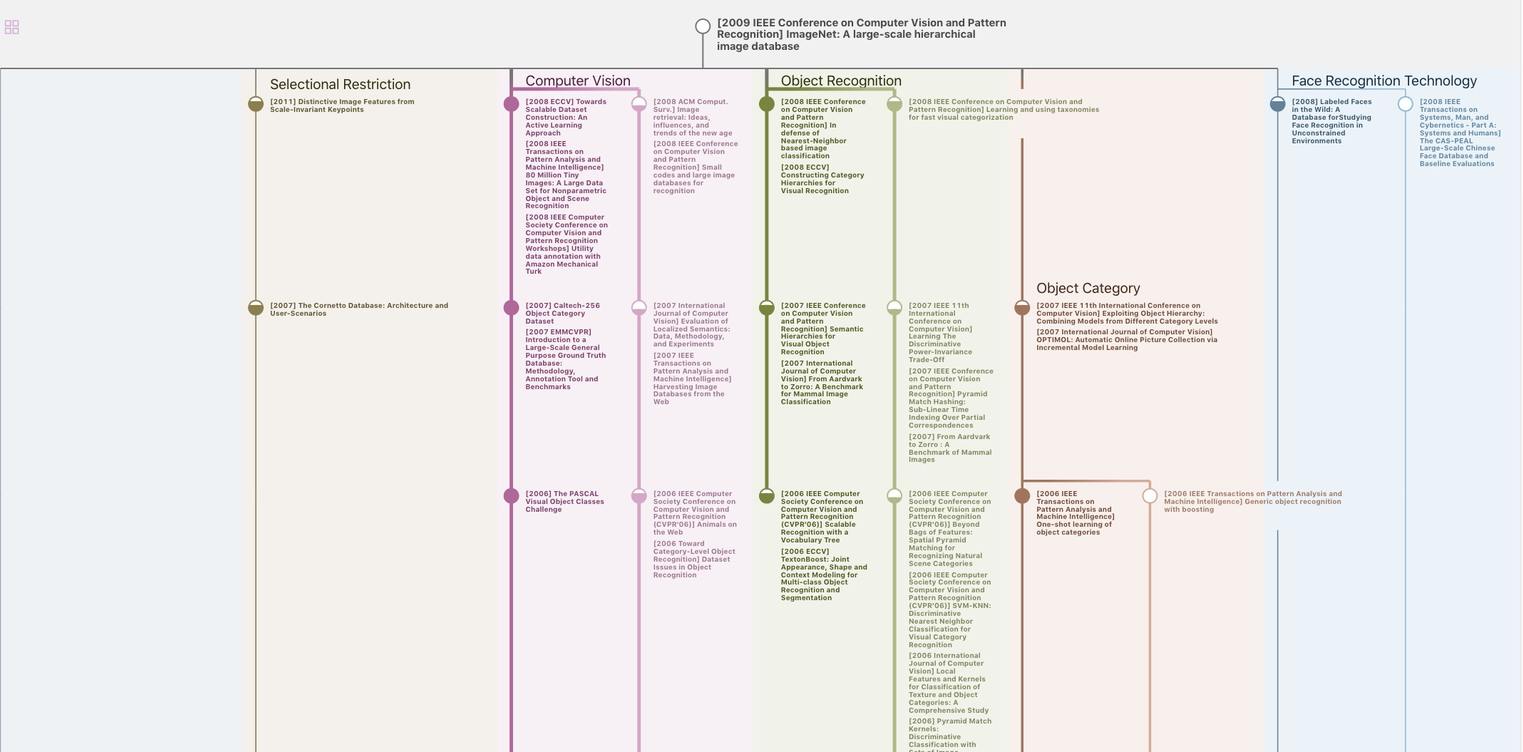
生成溯源树,研究论文发展脉络
Chat Paper
正在生成论文摘要