Statistical Limits of Adaptive Linear Models: Low-Dimensional Estimation and Inference
NeurIPS(2023)
摘要
Estimation and inference in statistics pose significant challenges when data are collected adaptively. Even in linear models, the Ordinary Least Squares (OLS) estimator may fail to exhibit asymptotic normality for single coordinate estimation and have inflated error. This issue is highlighted by a recent minimax lower bound, which shows that the error of estimating a single coordinate can be enlarged by a multiple of $\sqrt{d}$ when data are allowed to be arbitrarily adaptive, compared with the case when they are i.i.d. Our work explores this striking difference in estimation performance between utilizing i.i.d. and adaptive data. We investigate how the degree of adaptivity in data collection impacts the performance of estimating a low-dimensional parameter component in high-dimensional linear models. We identify conditions on the data collection mechanism under which the estimation error for a low-dimensional parameter component matches its counterpart in the i.i.d. setting, up to a factor that depends on the degree of adaptivity. We show that OLS or OLS on centered data can achieve this matching error. In addition, we propose a novel estimator for single coordinate inference via solving a Two-stage Adaptive Linear Estimating equation (TALE). Under a weaker form of adaptivity in data collection, we establish an asymptotic normality property of the proposed estimator.
更多查看译文
关键词
adaptive linear models,estimation
AI 理解论文
溯源树
样例
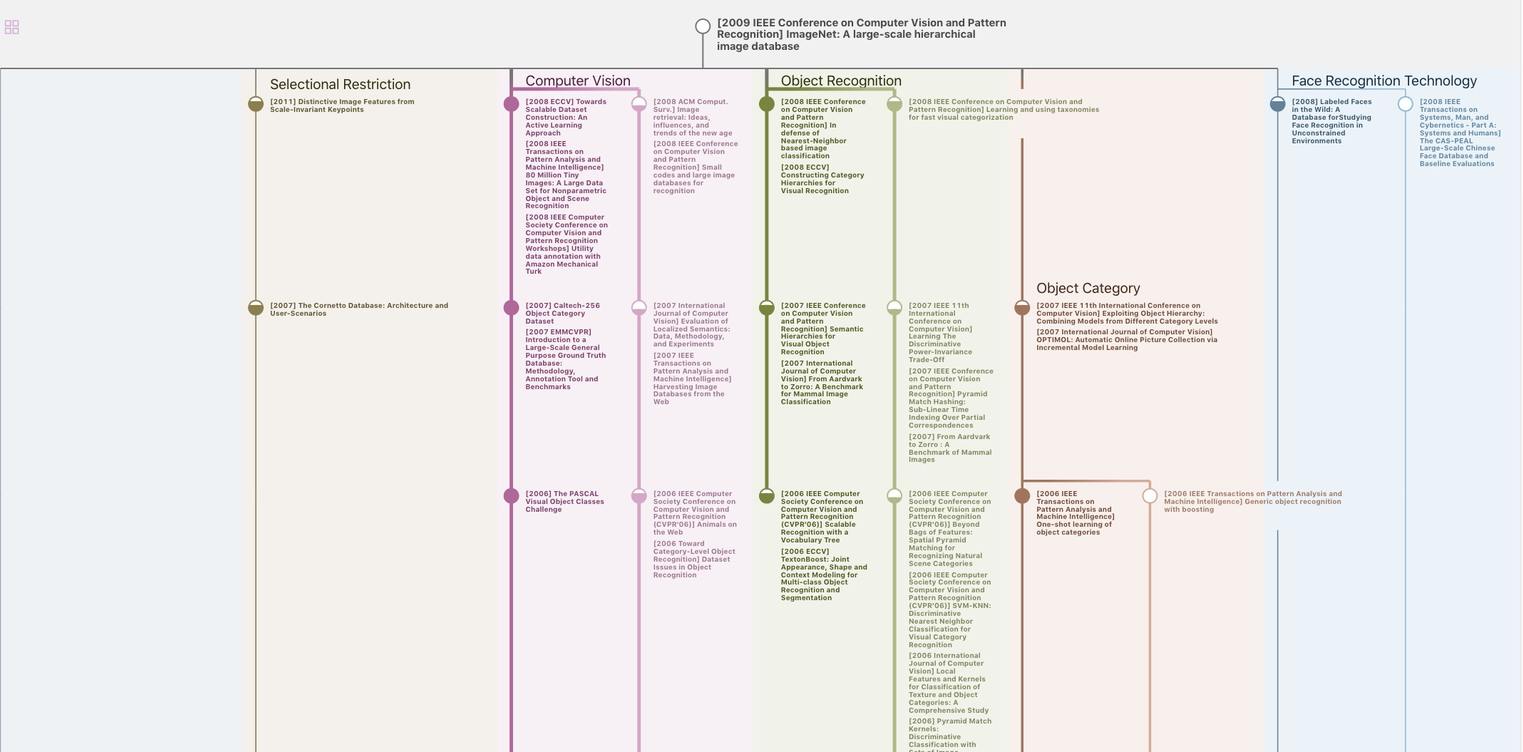
生成溯源树,研究论文发展脉络
Chat Paper
正在生成论文摘要