On Memorization and Privacy Risks of Sharpness Aware Minimization
CoRR(2023)
摘要
In many recent works, there is an increased focus on designing algorithms
that seek flatter optima for neural network loss optimization as there is
empirical evidence that it leads to better generalization performance in many
datasets. In this work, we dissect these performance gains through the lens of
data memorization in overparameterized models. We define a new metric that
helps us identify which data points specifically do algorithms seeking flatter
optima do better when compared to vanilla SGD. We find that the generalization
gains achieved by Sharpness Aware Minimization (SAM) are particularly
pronounced for atypical data points, which necessitate memorization. This
insight helps us unearth higher privacy risks associated with SAM, which we
verify through exhaustive empirical evaluations. Finally, we propose mitigation
strategies to achieve a more desirable accuracy vs privacy tradeoff.
更多查看译文
关键词
sharpness aware minimization,memorization,privacy risks
AI 理解论文
溯源树
样例
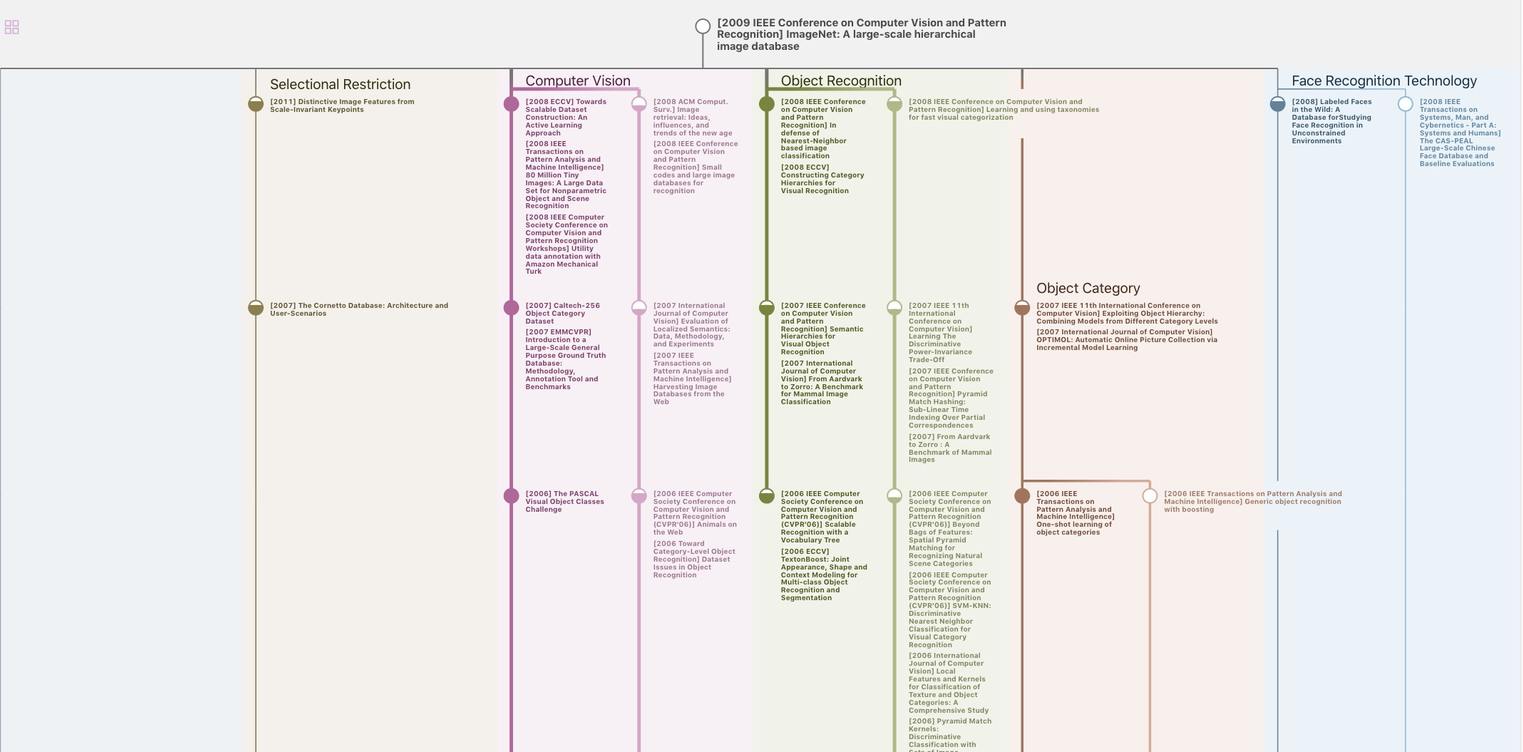
生成溯源树,研究论文发展脉络
Chat Paper
正在生成论文摘要