Mitigating the Effect of Incidental Correlations on Part-based Learning
arXiv (Cornell University)(2023)
摘要
Intelligent systems possess a crucial characteristic of breaking complicated problems into smaller reusable components or parts and adjusting to new tasks using these part representations. However, current part-learners encounter difficulties in dealing with incidental correlations resulting from the limited observations of objects that may appear only in specific arrangements or with specific backgrounds. These incidental correlations may have a detrimental impact on the generalization and interpretability of learned part representations. This study asserts that part-based representations could be more interpretable and generalize better with limited data, employing two innovative regularization methods. The first regularization separates foreground and background information's generative process via a unique mixture-of-parts formulation. Structural constraints are imposed on the parts using a weakly-supervised loss, guaranteeing that the mixture-of-parts for foreground and background entails soft, object-agnostic masks. The second regularization assumes the form of a distillation loss, ensuring the invariance of the learned parts to the incidental background correlations. Furthermore, we incorporate sparse and orthogonal constraints to facilitate learning high-quality part representations. By reducing the impact of incidental background correlations on the learned parts, we exhibit state-of-the-art (SoTA) performance on few-shot learning tasks on benchmark datasets, including MiniImagenet, TieredImageNet, and FC100. We also demonstrate that the part-based representations acquired through our approach generalize better than existing techniques, even under domain shifts of the background and common data corruption on the ImageNet-9 dataset. The implementation is available on GitHub: https://github.com/GauravBh1010tt/DPViT.git
更多查看译文
关键词
learning,incidental correlations,part-based
AI 理解论文
溯源树
样例
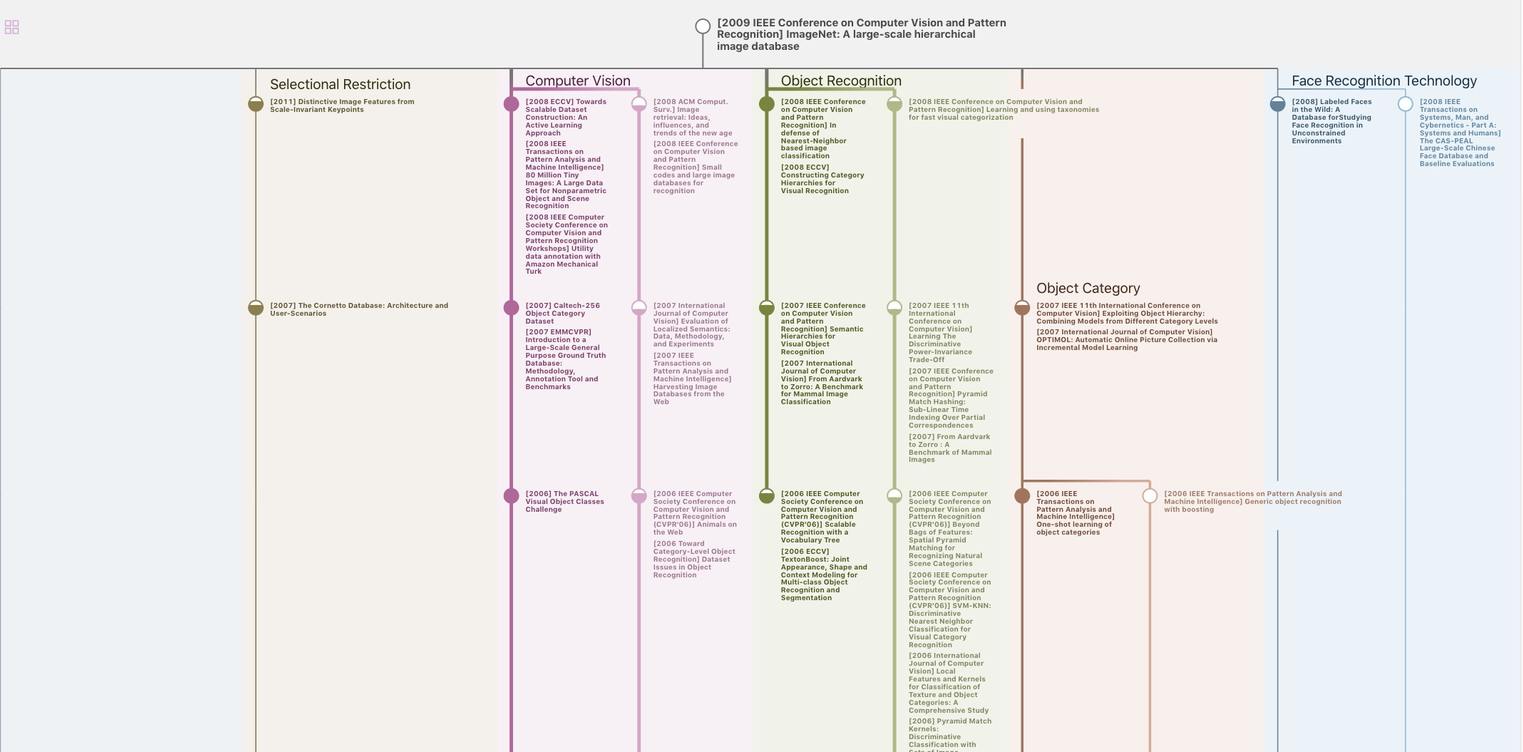
生成溯源树,研究论文发展脉络
Chat Paper
正在生成论文摘要