Cluster Persistence for Weighted Graphs
ENTROPY(2023)
摘要
Persistent homology is a natural tool for probing the topological characteristics of weighted graphs, essentially focusing on their 0-dimensional homology. While this area has been thoroughly studied, we present a new approach to constructing a filtration for cluster analysis via persistent homology. The key advantages of the new filtration is that (a) it provides richer signatures for connected components by introducing non-trivial birth times, and (b) it is robust to outliers. The key idea is that nodes are ignored until they belong to sufficiently large clusters. We demonstrate the computational efficiency of our filtration, its practical effectiveness, and explore into its properties when applied to random graphs.
更多查看译文
关键词
topological data analysis,universality,clustering
AI 理解论文
溯源树
样例
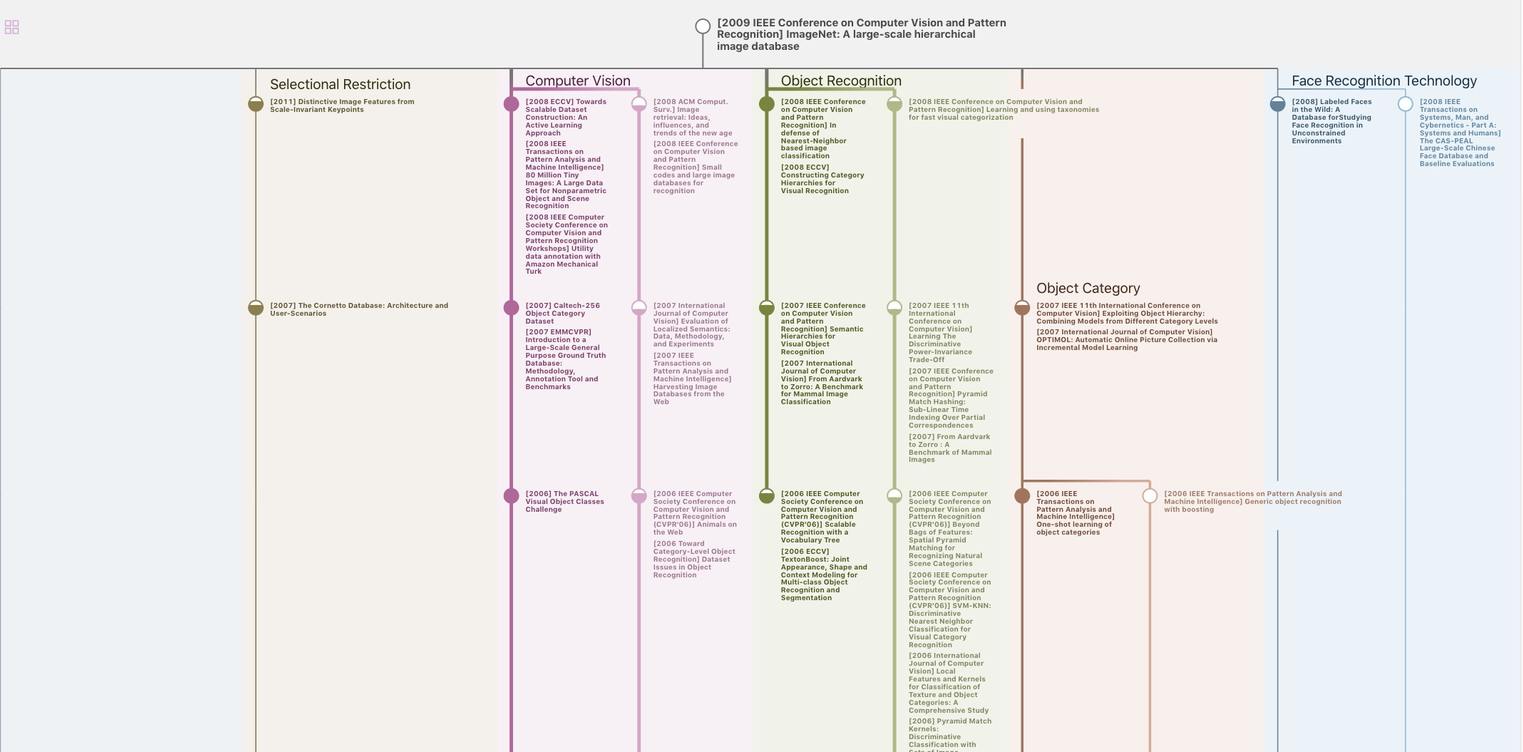
生成溯源树,研究论文发展脉络
Chat Paper
正在生成论文摘要