DURENDAL: Graph deep learning framework for temporal heterogeneous networks
arxiv(2023)
摘要
Temporal heterogeneous networks (THNs) are evolving networks that
characterize many real-world applications such as citation and events networks,
recommender systems, and knowledge graphs. Although different Graph Neural
Networks (GNNs) have been successfully applied to dynamic graphs, most of them
only support homogeneous graphs or suffer from model design heavily influenced
by specific THNs prediction tasks. Furthermore, there is a lack of temporal
heterogeneous networked data in current standard graph benchmark datasets.
Hence, in this work, we propose DURENDAL, a graph deep learning framework for
THNs. DURENDAL can help to easily repurpose any heterogeneous graph learning
model to evolving networks by combining design principles from snapshot-based
and multirelational message-passing graph learning models. We introduce two
different schemes to update embedding representations for THNs, discussing the
strengths and weaknesses of both strategies. We also extend the set of
benchmarks for TNHs by introducing two novel high-resolution temporal
heterogeneous graph datasets derived from an emerging Web3 platform and a
well-established e-commerce website. Overall, we conducted the experimental
evaluation of the framework over four temporal heterogeneous network datasets
on future link prediction tasks in an evaluation setting that takes into
account the evolving nature of the data. Experiments show the prediction power
of DURENDAL compared to current solutions for evolving and dynamic graphs, and
the effectiveness of its model design.
更多查看译文
AI 理解论文
溯源树
样例
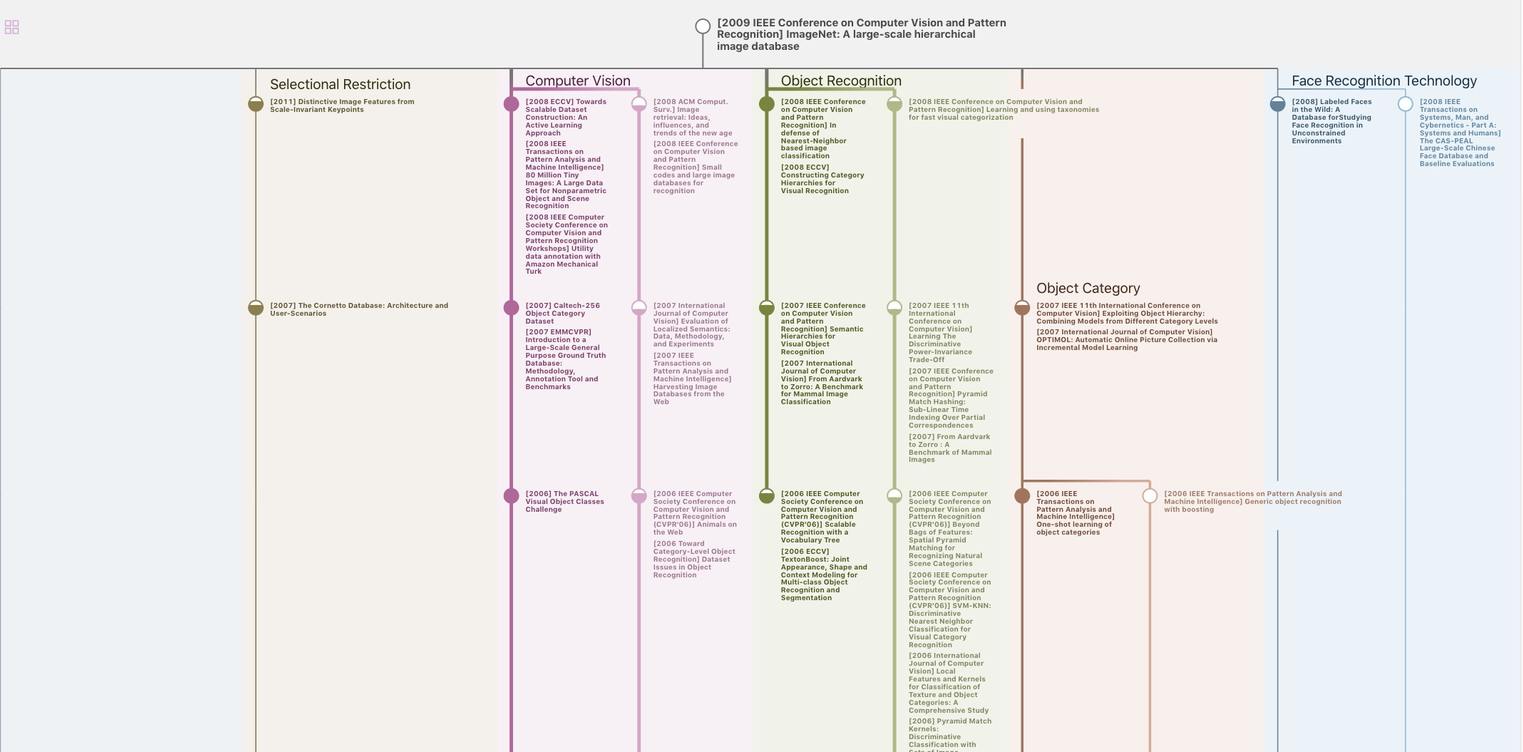
生成溯源树,研究论文发展脉络
Chat Paper
正在生成论文摘要