AdaptNet: Policy Adaptation for Physics-Based Character Control
ACM TRANSACTIONS ON GRAPHICS(2023)
摘要
Motivated by humans' ability to adapt skills in the learning of new ones, this paper presents AdaptNet, an approach for modifying the latent space of existing policies to allow new behaviors to be quickly learned from like tasks in comparison to learning from scratch. Building on top of a given reinforcement learning controller, AdaptNet uses a two-tier hierarchy that augments the original state embedding to support modest changes in a behavior and further modifies the policy network layers to make more substantive changes. The technique is shown to be effective for adapting existing physics-based controllers to a wide range of new styles for locomotion, new task targets, changes in character morphology and extensive changes in environment. Furthermore, it exhibits significant increase in learning efficiency, as indicated by greatly reduced training times when compared to training from scratch or using other approaches that modify existing policies. Code is available at https://motion-lab.github.io/AdaptNet.
更多查看译文
关键词
character animation,physics-based control,motion synthesis,reinforcement learning,motion style transfer,domain adaptation,GAN
AI 理解论文
溯源树
样例
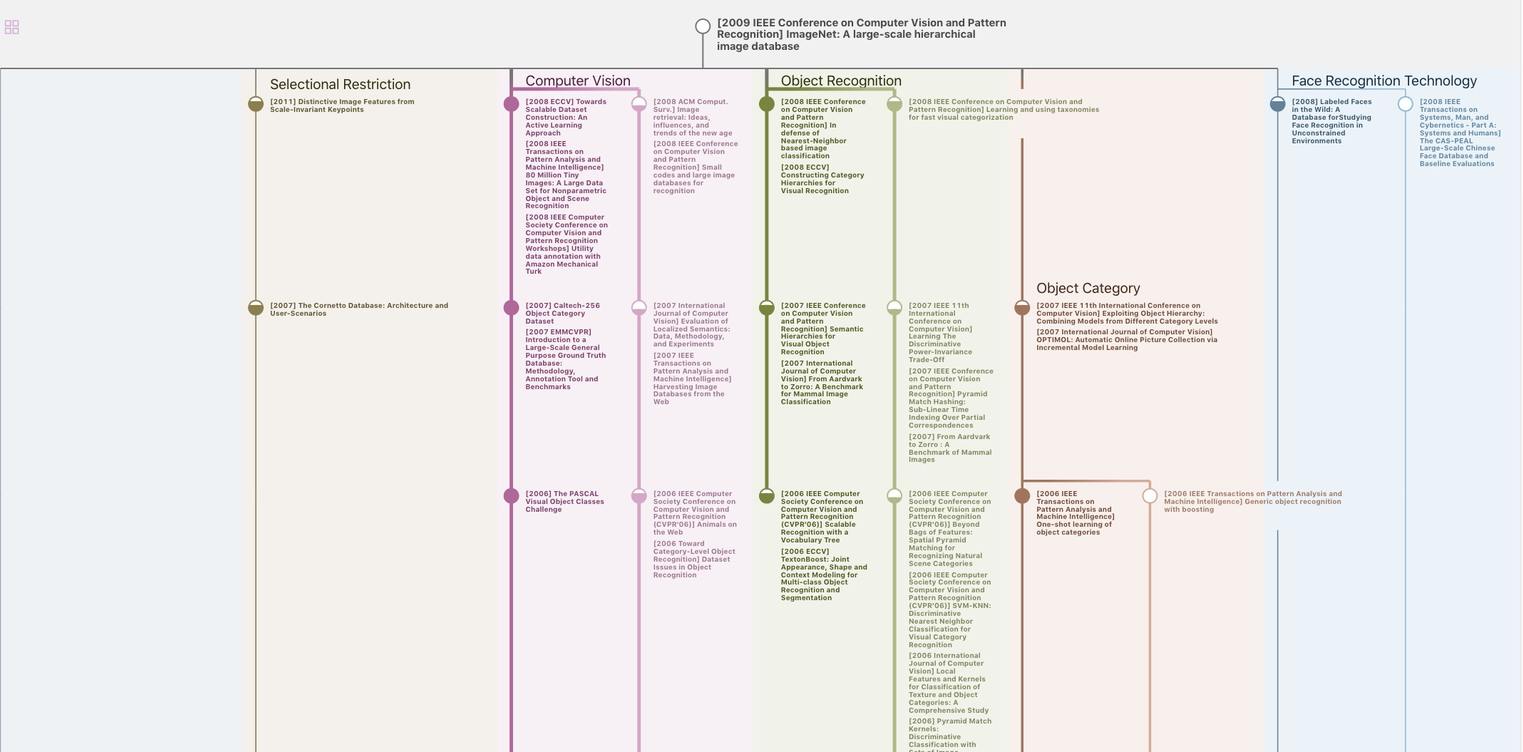
生成溯源树,研究论文发展脉络
Chat Paper
正在生成论文摘要