Joint Self-supervised Depth and Optical Flow Estimation towards Dynamic Objects
NEURAL PROCESSING LETTERS(2023)
摘要
Significant attention has been attracted to deep learning-based depth estimates. Dynamic objects become the most hard problems in inter-frame-supervised depth estimates due to the uncertainty in adjacent frames. Thus, integrating optical flow information with depth estimation is a feasible solution, as the optical flow is an essential motion representation. In this work, we construct a joint inter-frame-supervised depth and optical flow estimation framework, which predicts depths in various motions by minimizing pixel wrap errors in bilateral photometric re-projections and optical vectors. For motion segmentation, we adaptively segment the preliminary estimated optical flow map with large areas of connectivity. In self-supervised depth estimation, different motion regions are predicted independently and then composite into a complete depth. Further, the pose and depth estimations re-synthesize the optical flow maps, serving to compute reconstruction errors with the preliminary predictions. Our proposed joint depth and optical flow estimation outperforms existing depth estimators on the KITTI Depth dataset, both with and without Cityscapes pretraining. Additionally, our optical flow results demonstrate competitive performance on the KITTI Flow 2015 dataset.
更多查看译文
关键词
optical flow estimation,depth,objects,self-supervised
AI 理解论文
溯源树
样例
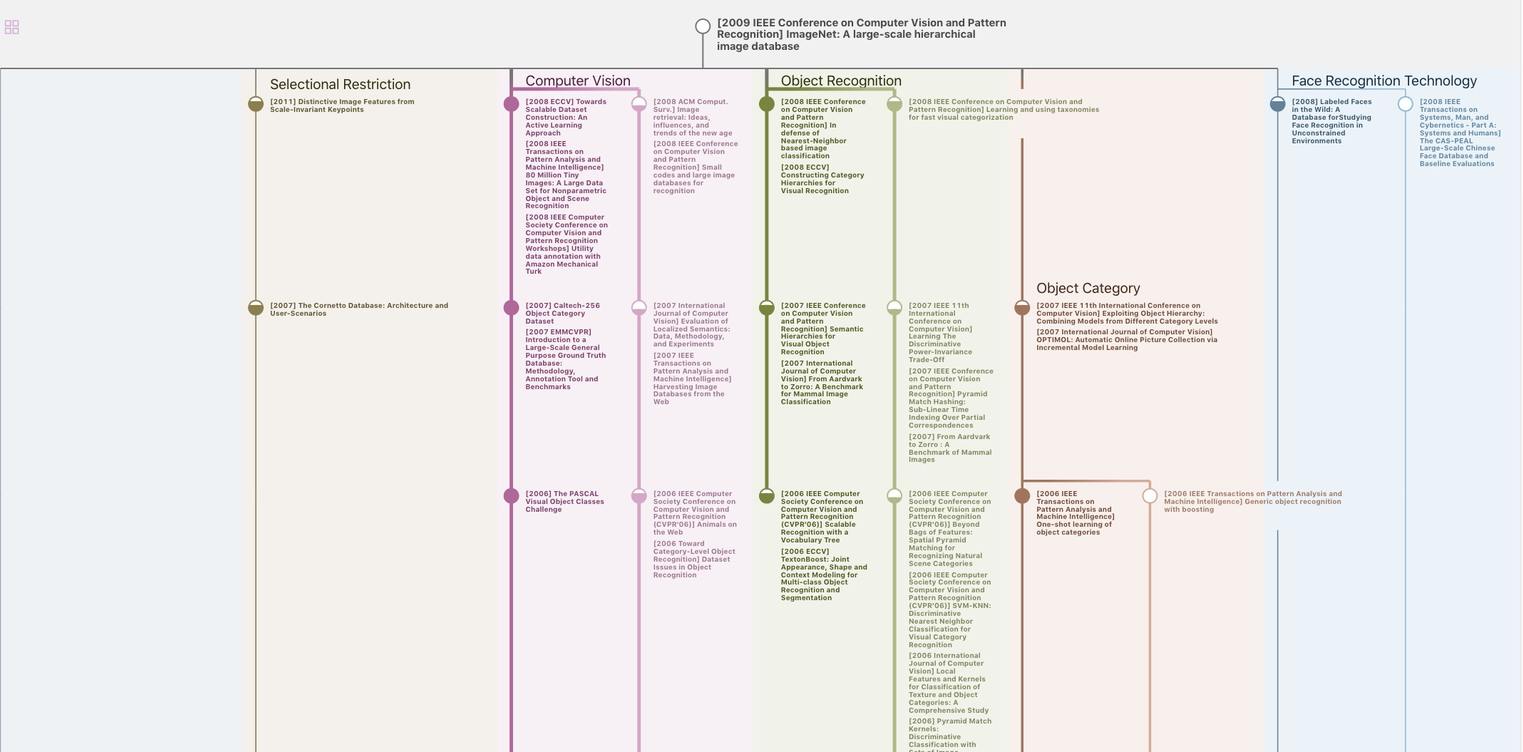
生成溯源树,研究论文发展脉络
Chat Paper
正在生成论文摘要