Learning Decentralized Flocking Controllers with Spatio-Temporal Graph Neural Network
CoRR(2023)
摘要
Recently a line of researches has delved the use of graph neural networks (GNNs) for decentralized control in swarm robotics. However, it has been observed that relying solely on the states of immediate neighbors is insufficient to imitate a centralized control policy. To address this limitation, prior studies proposed incorporating $L$-hop delayed states into the computation. While this approach shows promise, it can lead to a lack of consensus among distant flock members and the formation of small clusters, consequently resulting in the failure of cohesive flocking behaviors. Instead, our approach leverages spatiotemporal GNN, named STGNN that encompasses both spatial and temporal expansions. The spatial expansion collects delayed states from distant neighbors, while the temporal expansion incorporates previous states from immediate neighbors. The broader and more comprehensive information gathered from both expansions results in more effective and accurate predictions. We develop an expert algorithm for controlling a swarm of robots and employ imitation learning to train our decentralized STGNN model based on the expert algorithm. We simulate the proposed STGNN approach in various settings, demonstrating its decentralized capacity to emulate the global expert algorithm. Further, we implemented our approach to achieve cohesive flocking, leader following and obstacle avoidance by a group of Crazyflie drones. The performance of STGNN underscores its potential as an effective and reliable approach for achieving cohesive flocking, leader following and obstacle avoidance tasks.
更多查看译文
关键词
decentralized flocking controllers,graph
AI 理解论文
溯源树
样例
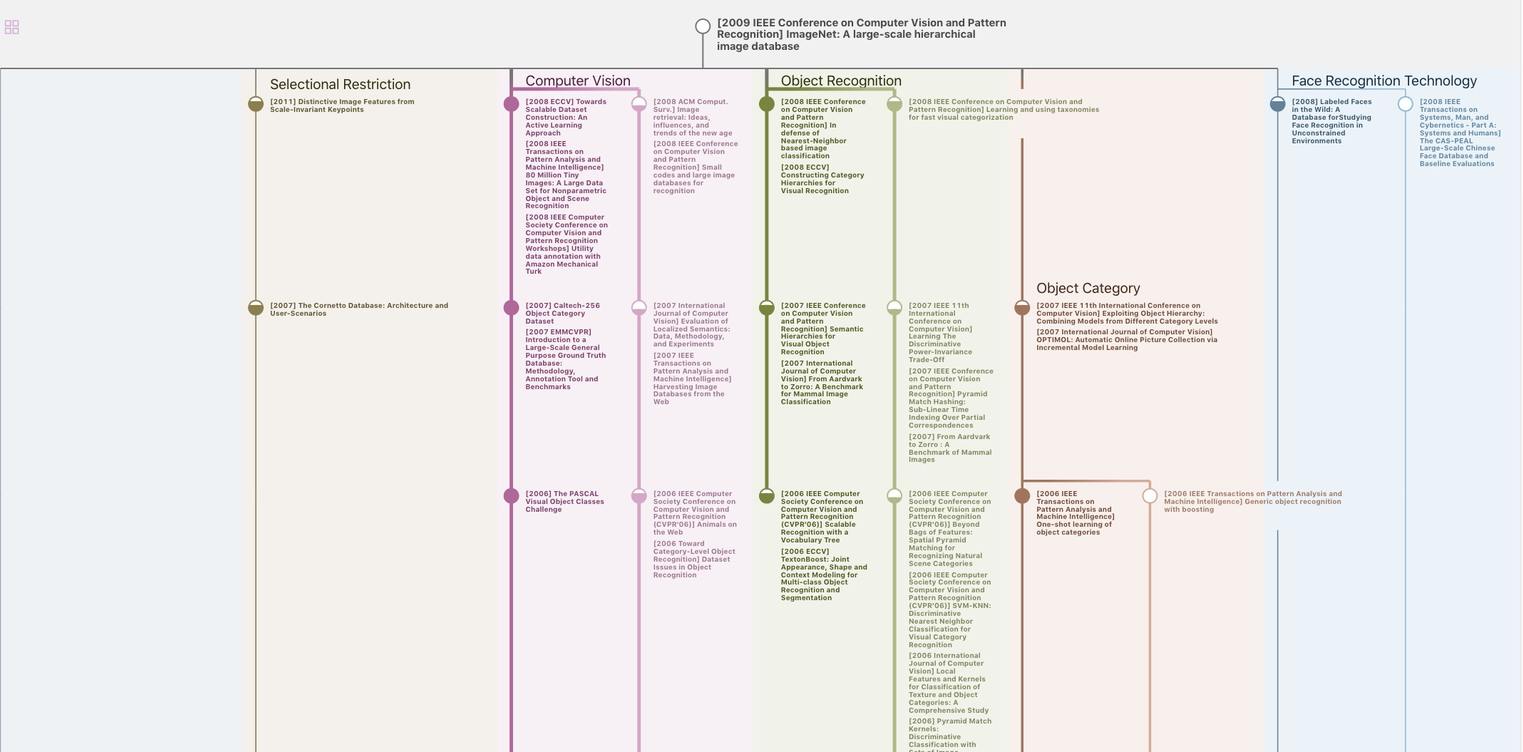
生成溯源树,研究论文发展脉络
Chat Paper
正在生成论文摘要