Forward Flow for Novel View Synthesis of Dynamic Scenes
Proceedings of the IEEE/CVF International Conference on Computer Vision (ICCV)(2023)
摘要
This paper proposes a neural radiance field (NeRF) approach for novel view synthesis of dynamic scenes using forward warping. Existing methods often adopt a static NeRF to represent the canonical space, and render dynamic images at other time steps by mapping the sampled 3D points back to the canonical space with the learned backward flow field. However, this backward flow field is non-smooth and discontinuous, which is difficult to be fitted by commonly used smooth motion models. To address this problem, we propose to estimate the forward flow field and directly warp the canonical radiance field to other time steps. Such forward flow field is smooth and continuous within the object region, which benefits the motion model learning. To achieve this goal, we represent the canonical radiance field with voxel grids to enable efficient forward warping, and propose a differentiable warping process, including an average splatting operation and an inpaint network, to resolve the many-to-one and one-to-many mapping issues. Thorough experiments show that our method outperforms existing methods in both novel view rendering and motion modeling, demonstrating the effectiveness of our forward flow motion modeling. Project page: https://npucvr.github.io/ForwardFlowDNeRF
更多查看译文
关键词
novel view synthesis,flow,dynamic,forward
AI 理解论文
溯源树
样例
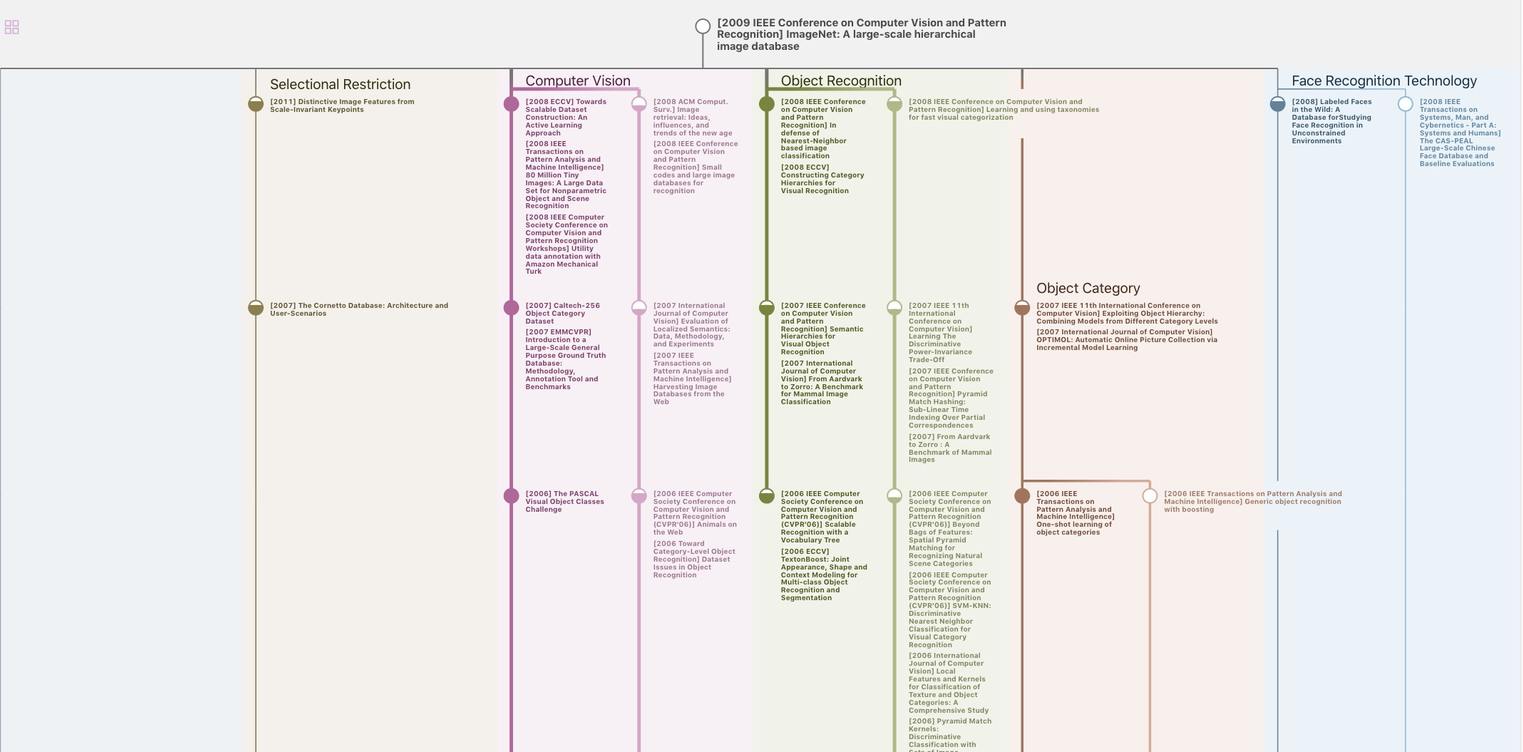
生成溯源树,研究论文发展脉络
Chat Paper
正在生成论文摘要