Optimal Bounds on Private Graph Approximation
ACM-SIAM Symposium on Discrete Algorithms(2023)
摘要
We propose an efficient $\epsilon$-differentially private algorithm, that given a simple {\em weighted} $n$-vertex, $m$-edge graph $G$ with a \emph{maximum unweighted} degree $\Delta(G) \leq n-1$, outputs a synthetic graph which approximates the spectrum with $\widetilde{O}(\min\{\Delta(G), \sqrt{n}\})$ bound on the purely additive error. To the best of our knowledge, this is the first $\epsilon$-differentially private algorithm with a non-trivial additive error for approximating the spectrum of the graph. One of the subroutines of our algorithm also precisely simulates the exponential mechanism over a non-convex set, which could be of independent interest given the recent interest in sampling from a {\em log-concave distribution} defined over a convex set. Spectral approximation also allows us to approximate all possible $(S,T)$-cuts, but it incurs an error that depends on the maximum degree, $\Delta(G)$. We further show that using our sampler, we can also output a synthetic graph that approximates the sizes of all $(S,T)$-cuts on $n$ vertices weighted graph $G$ with $m$ edges while preserving $(\epsilon,\delta)$-differential privacy and an additive error of $\widetilde{O}(\sqrt{mn}/\epsilon)$. We also give a matching lower bound (with respect to all the parameters) on the private cut approximation for weighted graphs. This removes the gap of $\sqrt{W_{\mathsf{avg}}}$ in the upper and lower bound in Eli{\'a}{\v{s}}, Kapralov, Kulkarni, and Lee (SODA 2020), where $W_{\mathsf{avg}}$ is the average edge weight.
更多查看译文
AI 理解论文
溯源树
样例
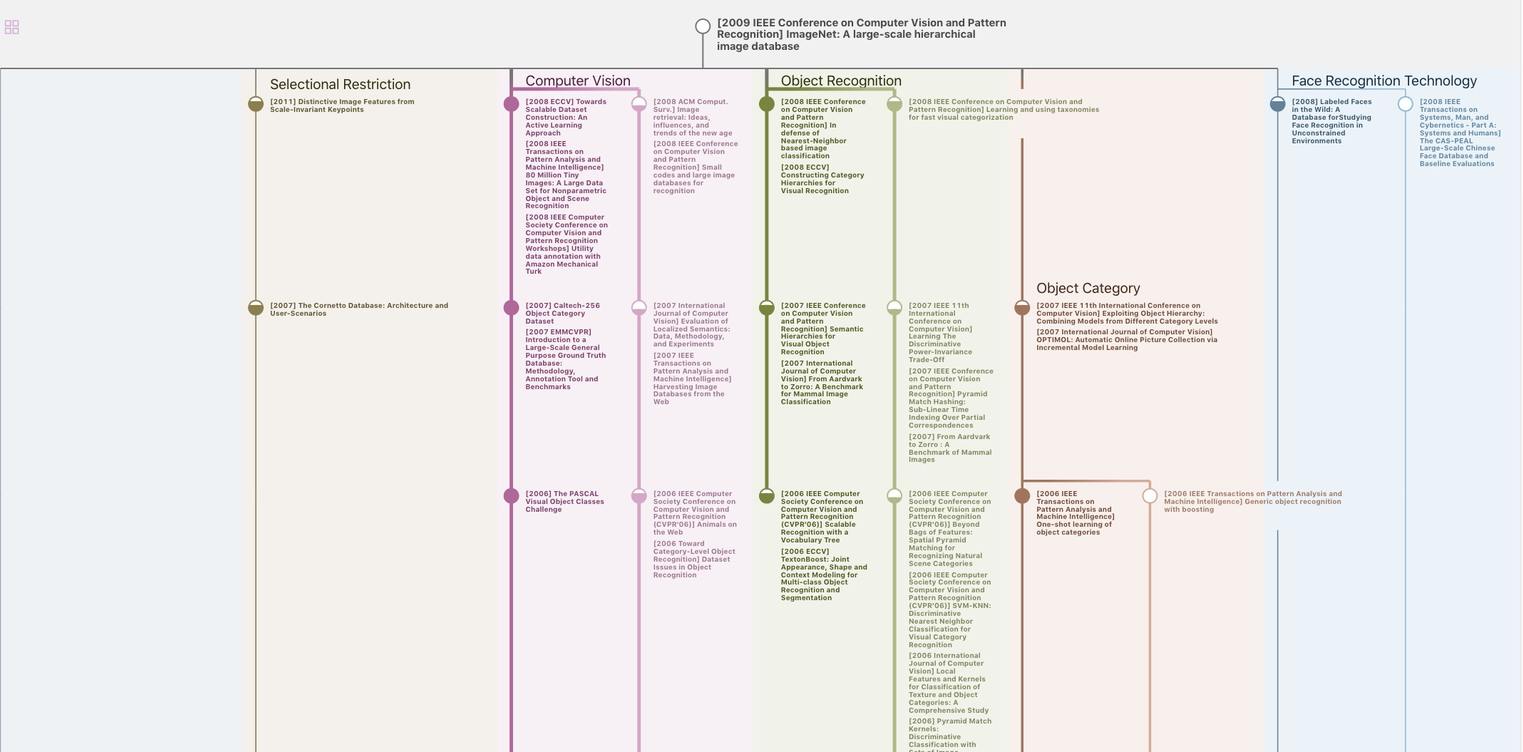
生成溯源树,研究论文发展脉络
Chat Paper
正在生成论文摘要