Utility-based Adaptive Teaching Strategies using Bayesian Theory of Mind
CoRR(2023)
摘要
Good teachers always tailor their explanations to the learners. Cognitive scientists model this process under the rationality principle: teachers try to maximise the learner's utility while minimising teaching costs. To this end, human teachers seem to build mental models of the learner's internal state, a capacity known as Theory of Mind (ToM). Inspired by cognitive science, we build on Bayesian ToM mechanisms to design teacher agents that, like humans, tailor their teaching strategies to the learners. Our ToM-equipped teachers construct models of learners' internal states from observations and leverage them to select demonstrations that maximise the learners' rewards while minimising teaching costs. Our experiments in simulated environments demonstrate that learners taught this way are more efficient than those taught in a learner-agnostic way. This effect gets stronger when the teacher's model of the learner better aligns with the actual learner's state, either using a more accurate prior or after accumulating observations of the learner's behaviour. This work is a first step towards social machines that teach us and each other, see https://teacher-with-tom.github.io.
更多查看译文
关键词
adaptive teaching strategies,teaching strategies,bayesian theory,utility-based
AI 理解论文
溯源树
样例
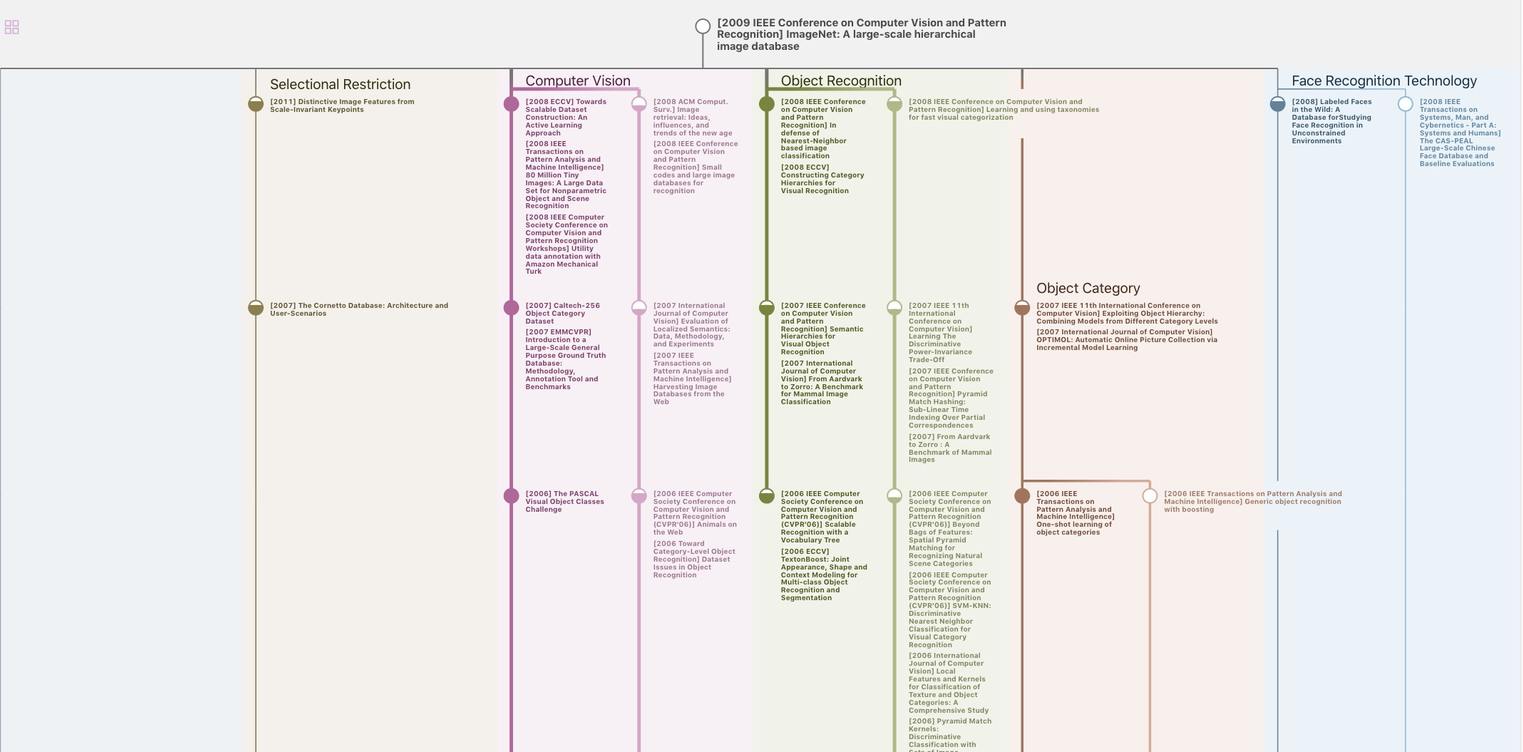
生成溯源树,研究论文发展脉络
Chat Paper
正在生成论文摘要