Batch Calibration: Rethinking Calibration for In-Context Learning and Prompt Engineering
ICLR 2024(2023)
摘要
Prompting and in-context learning (ICL) have become efficient learning
paradigms for large language models (LLMs). However, LLMs suffer from prompt
brittleness and various bias factors in the prompt, including but not limited
to the formatting, the choice verbalizers, and the ICL examples. To address
this problem that results in unexpected performance degradation, calibration
methods have been developed to mitigate the effects of these biases while
recovering LLM performance. In this work, we first conduct a systematic
analysis of the existing calibration methods, where we both provide a unified
view and reveal the failure cases. Inspired by these analyses, we propose Batch
Calibration (BC), a simple yet intuitive method that controls the contextual
bias from the batched input, unifies various prior approaches, and effectively
addresses the aforementioned issues. BC is zero-shot, inference-only, and
incurs negligible additional costs. In the few-shot setup, we further extend BC
to allow it to learn the contextual bias from labeled data. We validate the
effectiveness of BC with PaLM 2-(S, M, L) and CLIP models and demonstrate
state-of-the-art performance over previous calibration baselines across more
than 10 natural language understanding and image classification tasks.
更多查看译文
关键词
large language model,in-context learning,calibration,prompt
AI 理解论文
溯源树
样例
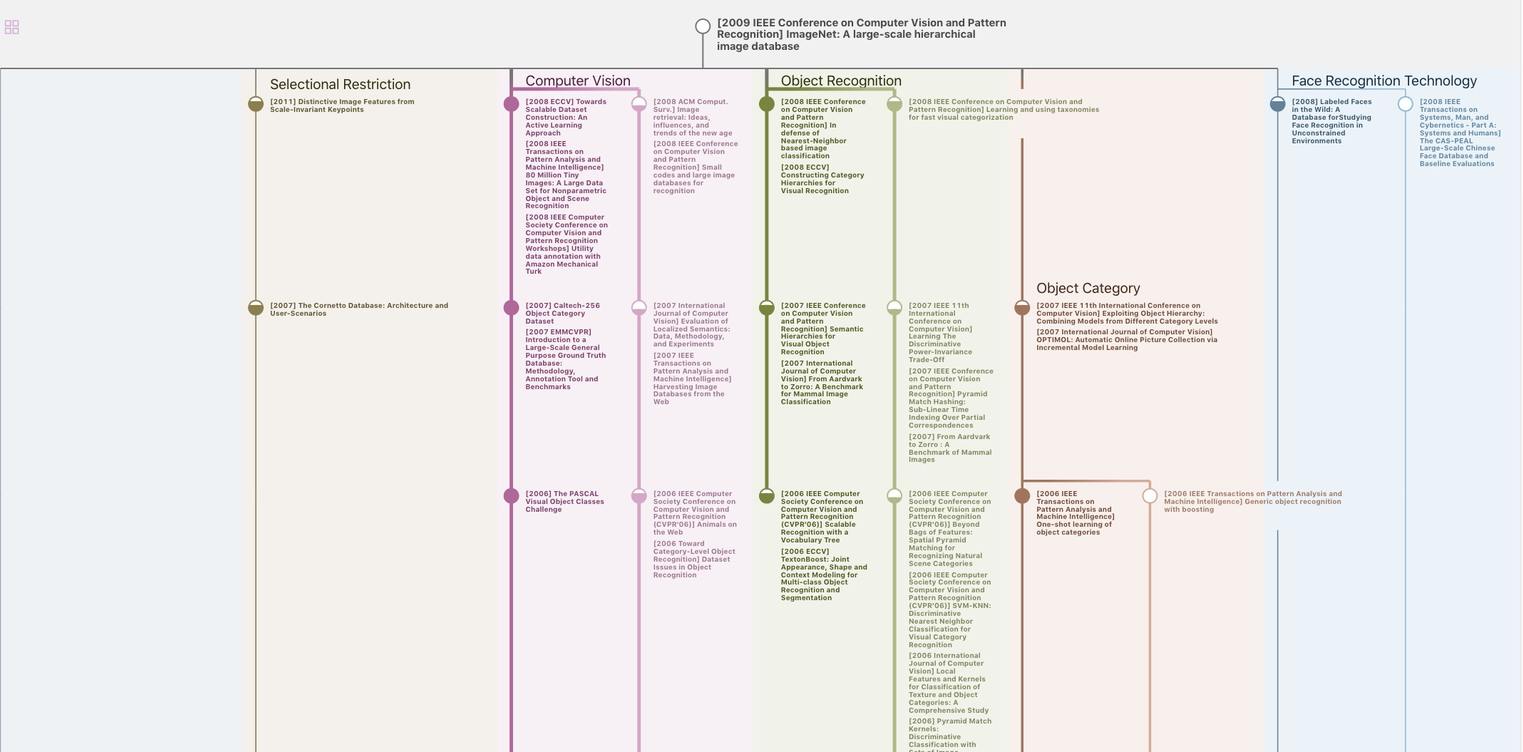
生成溯源树,研究论文发展脉络
Chat Paper
正在生成论文摘要