Benchmarking Collaborative Learning Methods Cost-Effectiveness for Prostate Segmentation
CoRR(2023)
摘要
Healthcare data is often split into medium/small-sized collections across multiple hospitals and access to it is encumbered by privacy regulations. This brings difficulties to use them for the development of machine learning and deep learning models, which are known to be data-hungry. One way to overcome this limitation is to use collaborative learning (CL) methods, which allow hospitals to work collaboratively to solve a task, without the need to explicitly share local data. In this paper, we address a prostate segmentation problem from MRI in a collaborative scenario by comparing two different approaches: federated learning (FL) and consensus-based methods (CBM). To the best of our knowledge, this is the first work in which CBM, such as label fusion techniques, are used to solve a problem of collaborative learning. In this setting, CBM combine predictions from locally trained models to obtain a federated strong learner with ideally improved robustness and predictive variance properties. Our experiments show that, in the considered practical scenario, CBMs provide equal or better results than FL, while being highly cost-effective. Our results demonstrate that the consensus paradigm may represent a valid alternative to FL for typical training tasks in medical imaging.
更多查看译文
关键词
learning,segmentation,cost-effectiveness
AI 理解论文
溯源树
样例
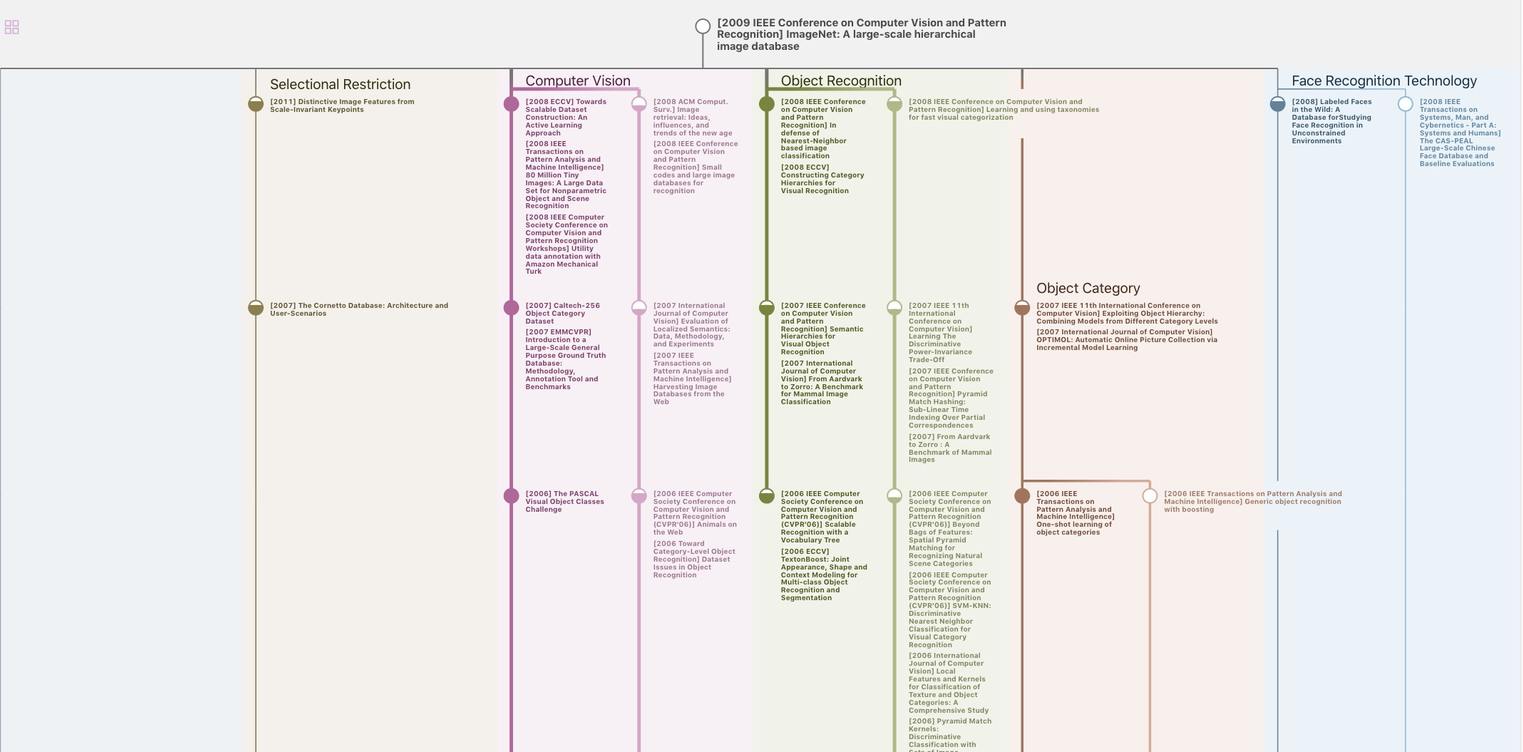
生成溯源树,研究论文发展脉络
Chat Paper
正在生成论文摘要