From Empirical Measurements to Augmented Data Rates: A Machine Learning Approach for MCS Adaptation in Sidelink Communication
2023 IEEE 98TH VEHICULAR TECHNOLOGY CONFERENCE, VTC2023-FALL(2023)
摘要
Due to the lack of a feedback channel in the C-V2X sidelink, finding a suitable modulation and coding scheme (MCS) is a difficult task. However, recent use cases for vehicle-to-everything (V2X) communication with higher demands on data rate necessitate choosing the MCS adaptively. In this paper, we propose a machine learning approach to predict suitable MCS levels. Additionally, we propose the use of quantile prediction and evaluate it in combination with different algorithms for the task of predicting the MCS level with the highest achievable data rate. Thereby, we show significant improvements over conventional methods of choosing the MCS level. Using a machine learning approach, however, requires larger real-world data sets than are currently publicly available for research. For this reason, this paper presents a data set that was acquired in extensive drive tests, and that we make publicly available.
更多查看译文
关键词
SDR,V2X,Sidelink,MCS Prediction,Machine learning,Mode-4
AI 理解论文
溯源树
样例
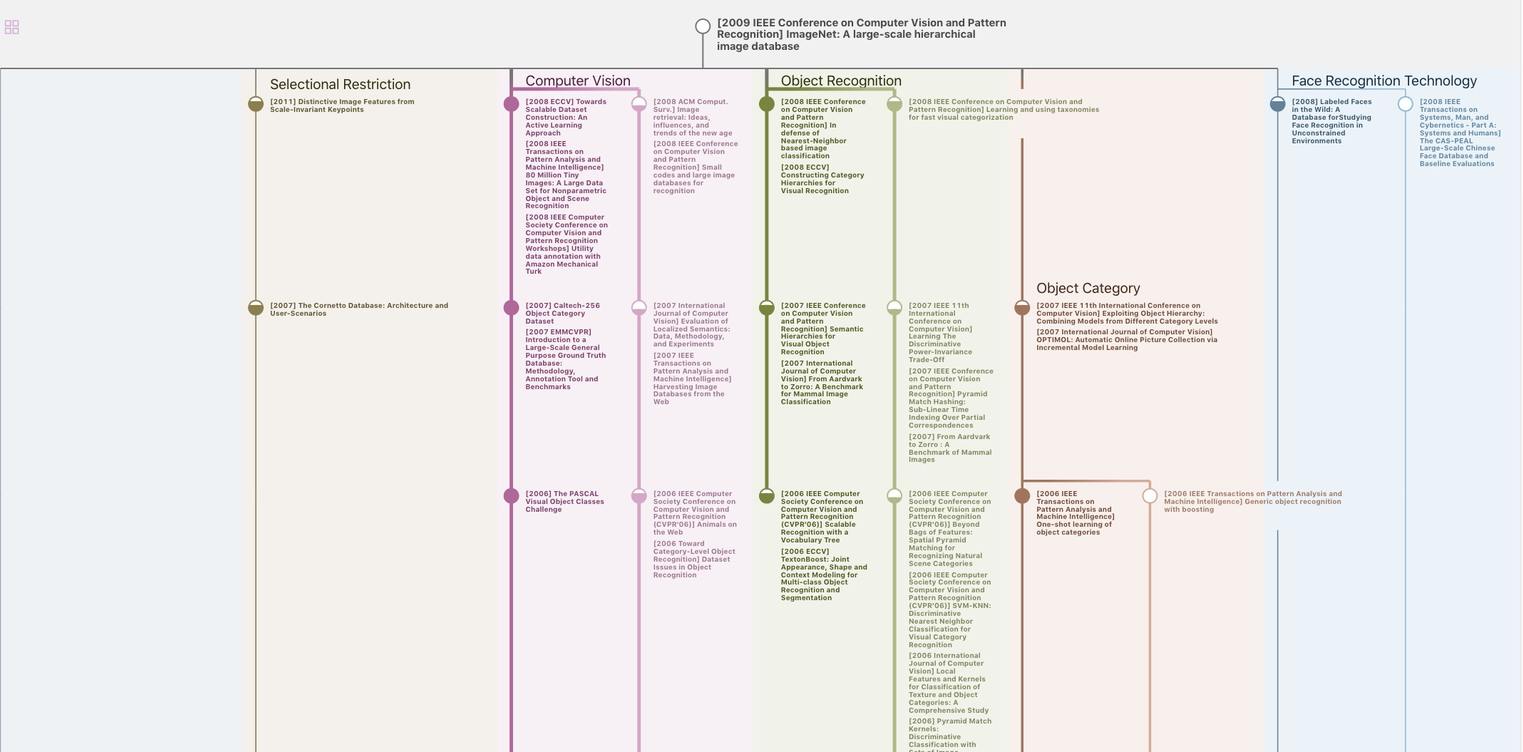
生成溯源树,研究论文发展脉络
Chat Paper
正在生成论文摘要