Unsupervised Large Language Model Alignment for Information Retrieval via Contrastive Feedback
arxiv(2023)
摘要
Large language models (LLMs) have demonstrated remarkable capabilities across
various research domains, including the field of Information Retrieval (IR).
However, the responses generated by off-the-shelf LLMs tend to be generic,
i.e., cannot capture the distinctiveness of each document with similar content.
This limits the performance of LLMs in IR because finding and distinguishing
relevant documents from substantial similar documents is a typical problem in
many IR tasks. To address this issue, we propose an unsupervised alignment
method, namely Reinforcement Learning from Contrastive Feedback (RLCF),
empowering LLMs to generate both high-quality and context-specific responses.
Our approach constructs unsupervised contrastive feedback signals based on
similar document groups, and adopts a reward function, named group-wise
reciprocal rank, to optimize LLMs within a standard Proximal Policy
Optimization. We conduct extensive experiments to evaluate the effectiveness of
RLCF on LLMs built with different languages and parameter sizes on multiple
downstream IR applications. RLCF significantly outperforms existing alignment
methods, and RLCF-optimized LLMs demonstrate considerable improvement in
generating responses with distinctiveness.
更多查看译文
AI 理解论文
溯源树
样例
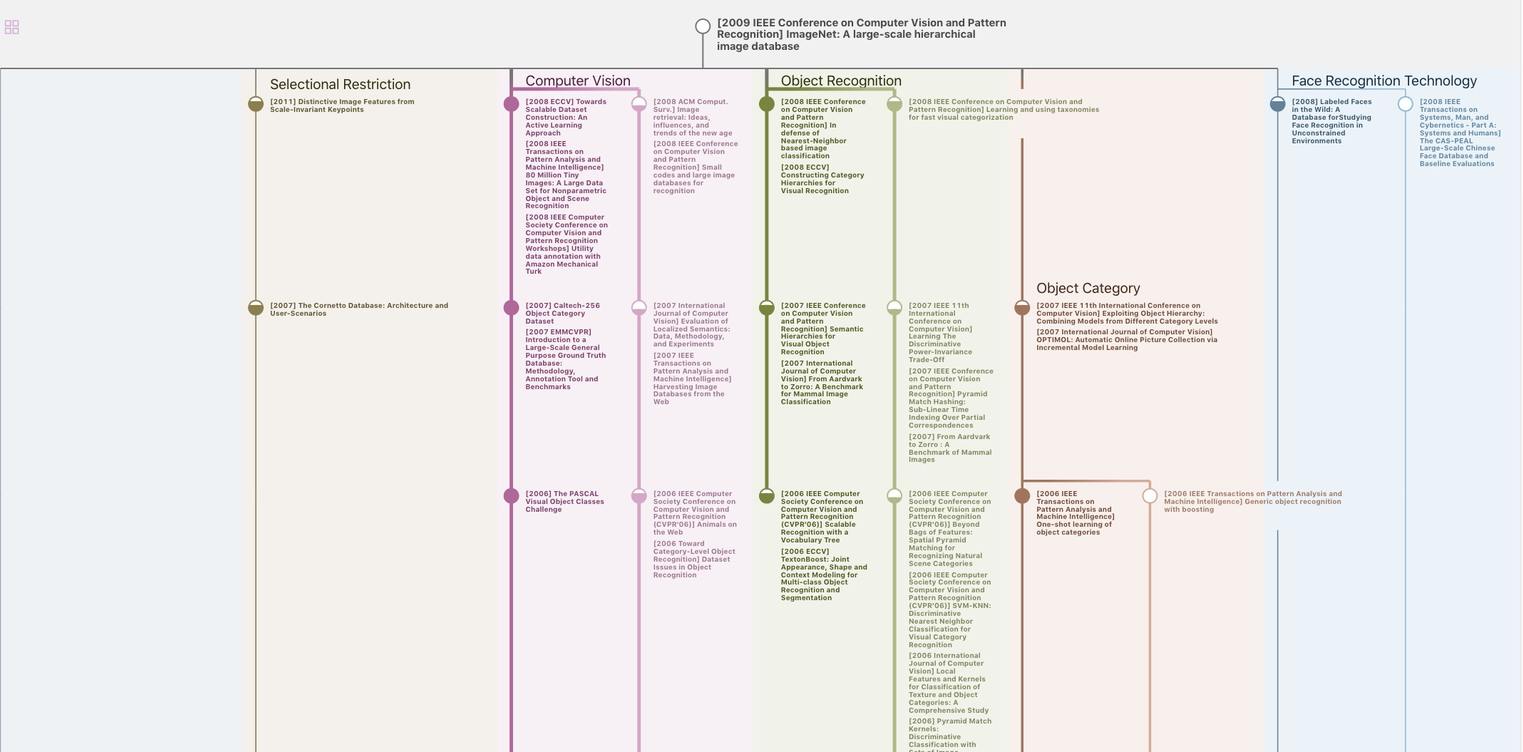
生成溯源树,研究论文发展脉络
Chat Paper
正在生成论文摘要