Algorithmic Recourse for Anomaly Detection in Multivariate Time Series
CoRR(2023)
摘要
Anomaly detection in multivariate time series has received extensive study due to the wide spectrum of applications. An anomaly in multivariate time series usually indicates a critical event, such as a system fault or an external attack. Therefore, besides being effective in anomaly detection, recommending anomaly mitigation actions is also important in practice yet under-investigated. In this work, we focus on algorithmic recourse in time series anomaly detection, which is to recommend fixing actions on abnormal time series with a minimum cost so that domain experts can understand how to fix the abnormal behavior. To this end, we propose an algorithmic recourse framework, called RecAD, which can recommend recourse actions to flip the abnormal time steps. Experiments on two synthetic and one real-world datasets show the effectiveness of our framework.
更多查看译文
关键词
anomaly detection,time series,algorithmic recourse,multivariate
AI 理解论文
溯源树
样例
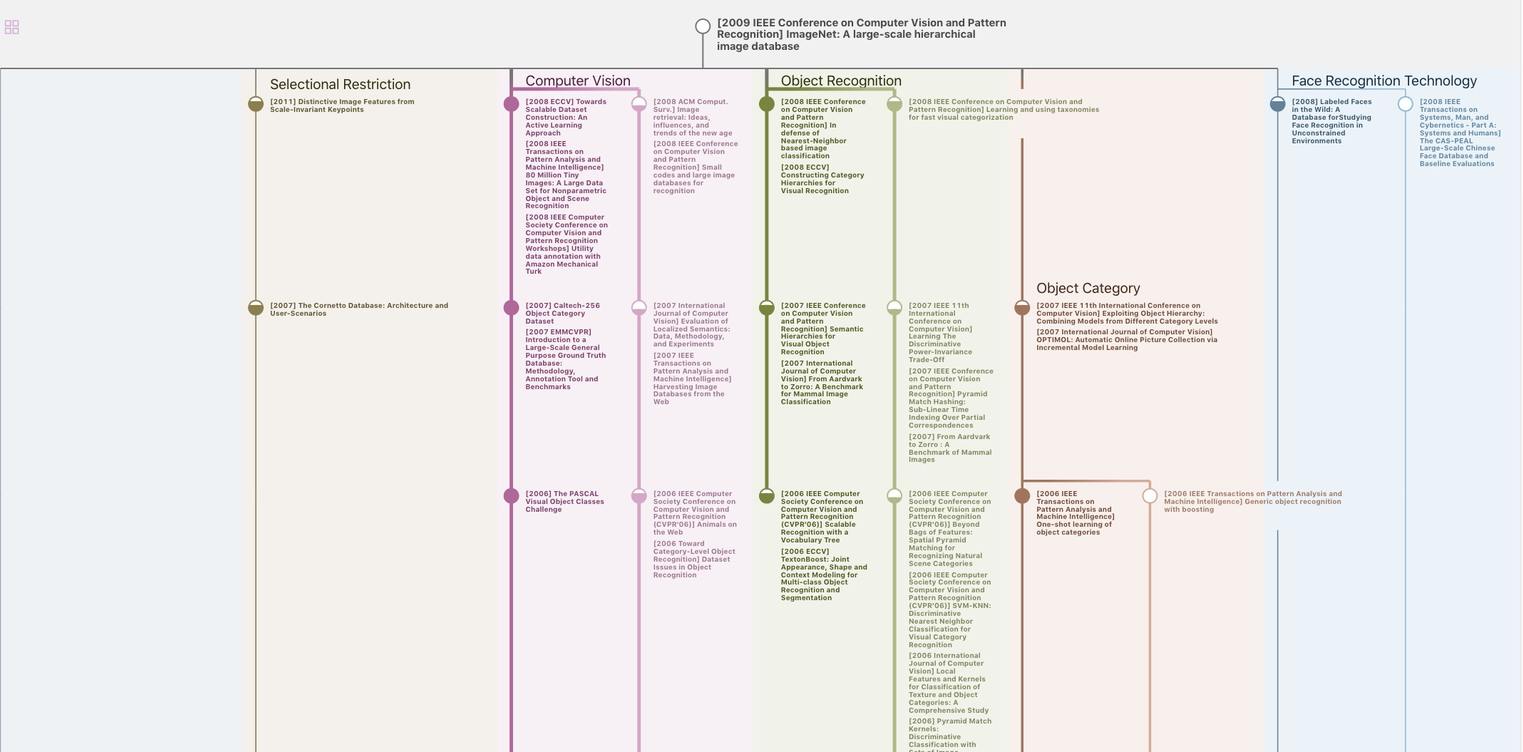
生成溯源树,研究论文发展脉络
Chat Paper
正在生成论文摘要