Joint Participation Incentive and Network Pricing Design for Federated Learning
INFOCOM(2023)
摘要
Federated learning protects users' data privacy through sharing users' local model parameters (instead of raw data) with a server. However, when massive users train a large machine learning model through federated learning, the dynamically varying and often heavy communication overhead can put significant pressure on the network operator. The operator may choose to dynamically change the network prices in response, which will eventually affect the payoffs of the server and users. This paper considers the under-explored yet important issue of the joint design of participation incentives (for encouraging users' contribution to federated learning) and network pricing (for managing network resources). Due to heterogeneous users' private information and multi-dimensional decisions, the optimization problems in Stage I of multi-stage games are non-convex. Nevertheless, we are able to analytically derive the corresponding optimal contract and pricing mechanism through proper transformations of constraints, variables, and functions, under both vertical and horizontal interaction structures of the participants. We show that the vertical structure is better than the horizontal one, as it avoids the interests misalignment between the server and the network operator. Numerical results based on real-world datasets show that our proposed mechanisms decrease server's cost by up to 24.87% comparing with the state-of-the-art benchmarks.
更多查看译文
关键词
Federated learning,incentive mechanism,dynamic network pricing,interaction structure comparison
AI 理解论文
溯源树
样例
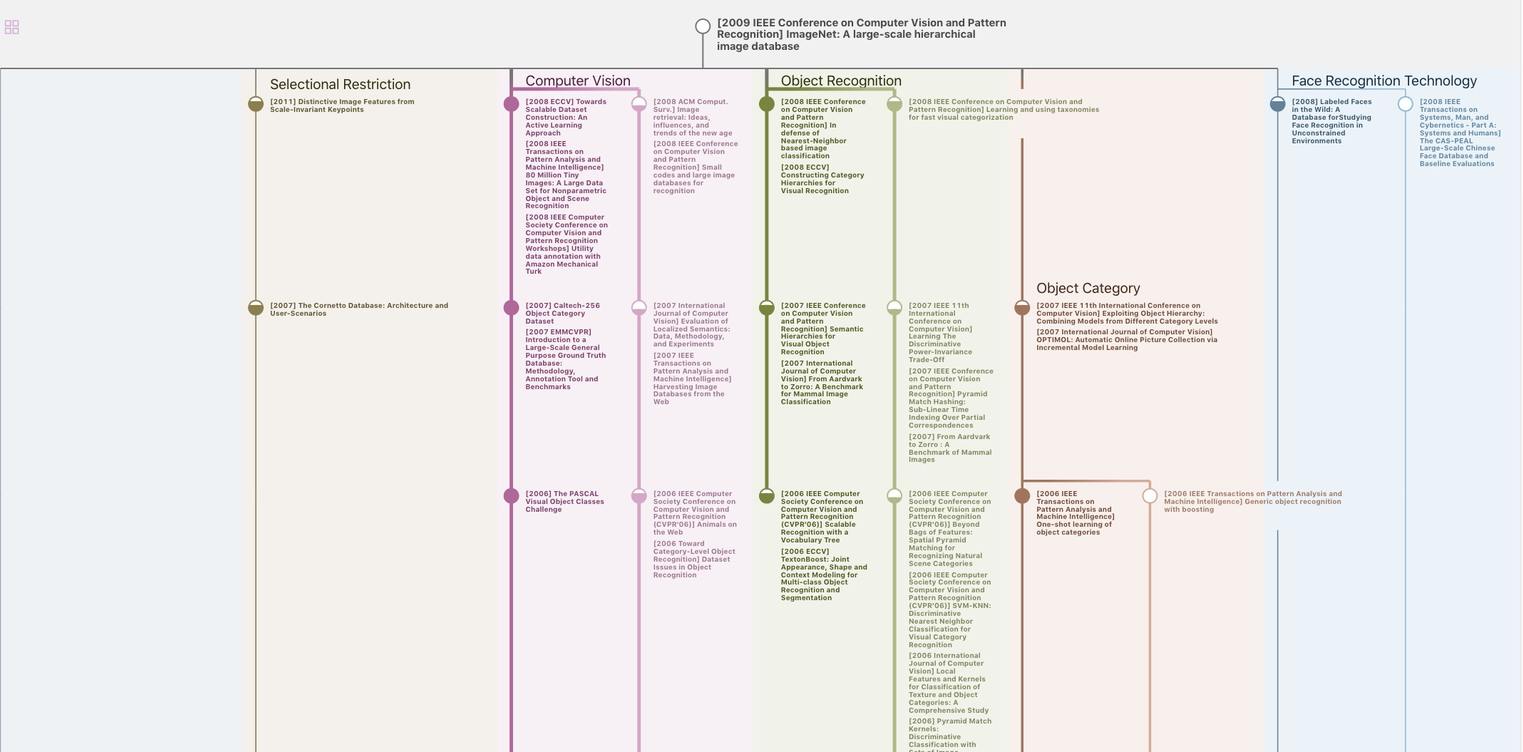
生成溯源树,研究论文发展脉络
Chat Paper
正在生成论文摘要