Self-Supervised Single-Line LiDAR Depth Completion
IEEE Robotics and Automation Letters(2023)
摘要
Depth completion plays a crucial role in enabling real-world applications such as obstacle avoidance and SLAM for robot navigation. This letter focuses on addressing the depth completion challenge for single-line LiDAR, commonly used in conjunction with visual cameras. The sparsity of valid depth points makes supervised methods inadequate, while existing self-supervised approaches are only applicable to 64-line LiDARs. In this letter, we propose a novel self-supervised approach for single-line LiDAR depth completion. Our approach makes two key contributions. Firstly, we introduce the Relative-to-Metric (R2M) depth distillation framework, which estimates a pixel-wise metric depth map using an RGB image and its corresponding single-line depth map. This is achieved by distilling relative depth predictions from a monocular depth estimator trained on RGB images. Secondly, we propose the Line Depth Prior (LDP), a model-agnostic geometry regularization technique that promotes depth completion. Through extensive experiments, we demonstrate that our proposed method can: i) accurately reconstruct complete depth maps from single-line depth inputs without requiring additional depth supervision, except for the observed entries, and ii) facilitate downstream SLAM tasks when using single-line LiDAR.
更多查看译文
关键词
depth,self-supervised,single-line
AI 理解论文
溯源树
样例
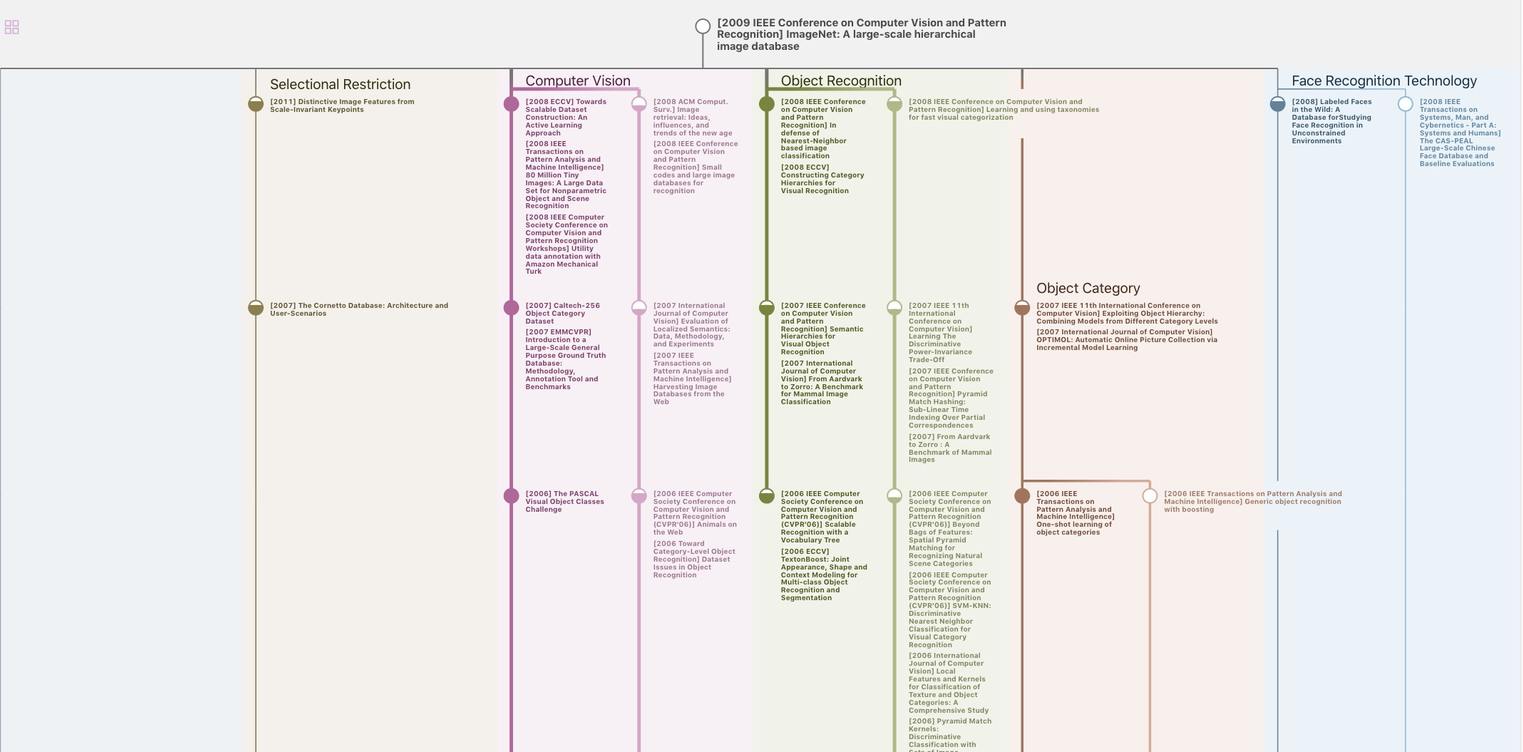
生成溯源树,研究论文发展脉络
Chat Paper
正在生成论文摘要