Cross-Modal Self-Supervised Feature Extraction for Anomaly Detection in Human Monitoring
2023 IEEE 19th International Conference on Automation Science and Engineering (CASE)(2023)
摘要
This paper proposes to extract cross-modal self-supervised features to detect anomalies in human monitoring. Our previous works that use deep captioning in addition to monitoring images were successful. However, their use of unimodally trained image and text features shows deficiencies in capturing contextual information across the modalities. We devise a self-supervised method that creates cross-modal features by maximizing the mutual information between both modalities in a common subspace. It allows capturing different complex distributions between modalities, improving the detection performance of clustering methods. Extensive experimental results show improvements in both AUC and AUPRC scores when compared to the best baselines on two real-world datasets. The AUC has improved from 0.895 to 0.969, and from 0.97 to 0.98. The AUPRC has improved from 0.681 to 0.850, and from 0.840 to 0.894.
更多查看译文
关键词
Cross-modal learning,Self-supervised learning,One-class anomaly detection
AI 理解论文
溯源树
样例
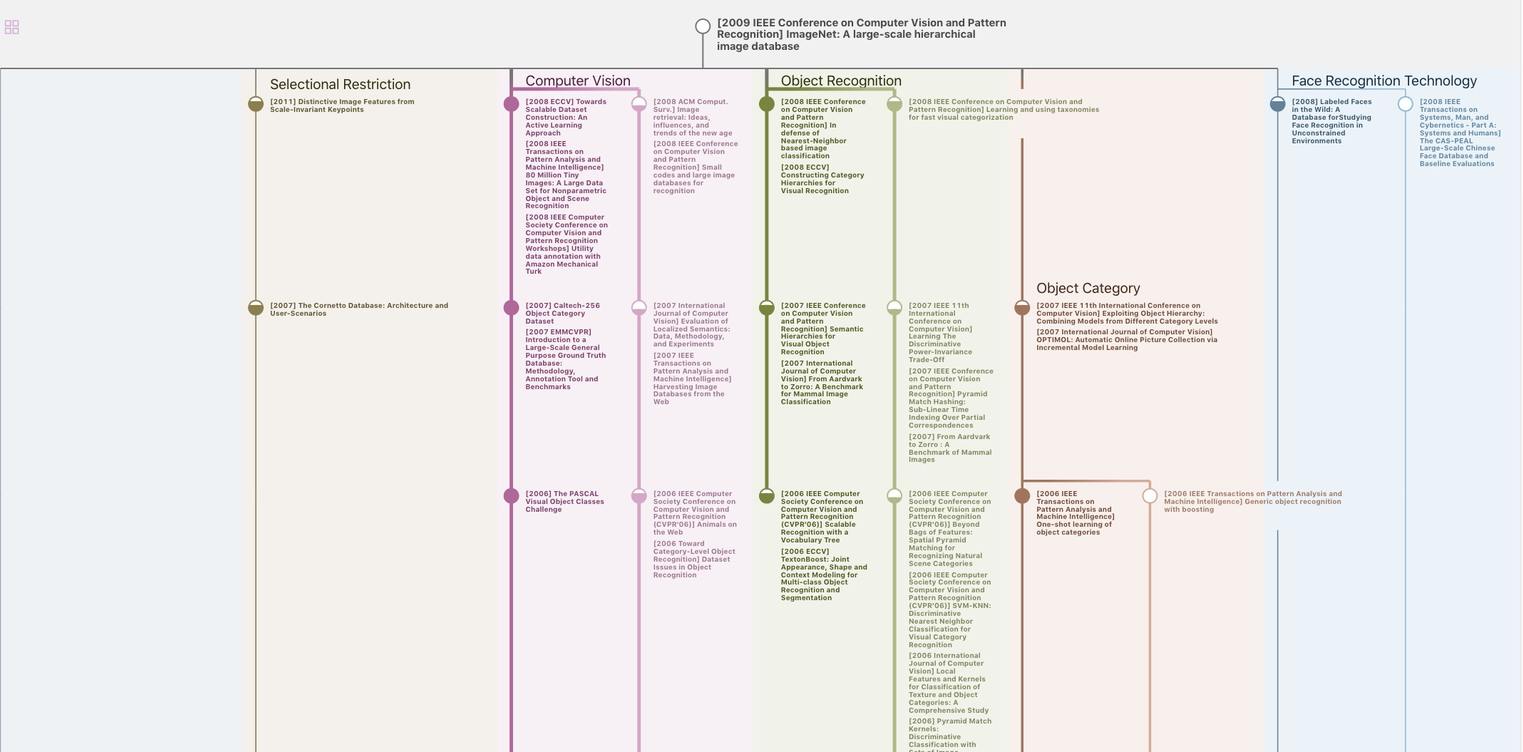
生成溯源树,研究论文发展脉络
Chat Paper
正在生成论文摘要