Mobile Application Privacy Risk Assessments from User-authored Scenarios
2023 IEEE 31st International Requirements Engineering Conference (RE)(2023)
摘要
Mobile applications (apps) provide users valuable benefits at the risk of exposing users to privacy harms. Improving privacy in mobile apps faces several challenges, in particular, that many apps are developed by low resourced software development teams, such as end-user programmers or in startups. In addition, privacy risks are primarily known to users, which can make it difficult for developers to prioritize privacy for sensitive data. In this paper, we introduce a novel, lightweight method that allows app developers to elicit scenarios and privacy risk scores from users directly using only an app screenshot. The technique relies on named entity recognition (NER) to identify information types in user-authored scenarios, which are then fed in real-time to a privacy risk survey that users complete. The best-performing NER model predicts information types with a weighted average precision of 0.70 and recall of 0.72, after post-processing to remove false positives. The model was trained on a labeled 300-scenario corpus, and evaluated in an end-to-end evaluation using an additional 203 scenarios yielding 2,338 user-provided privacy risk scores. Finally, we discuss how developers can use the risk scores to prioritize, select and apply privacy design strategies in the context of four user-authored scenarios.
更多查看译文
关键词
scenarios,entity extraction,privacy, risk
AI 理解论文
溯源树
样例
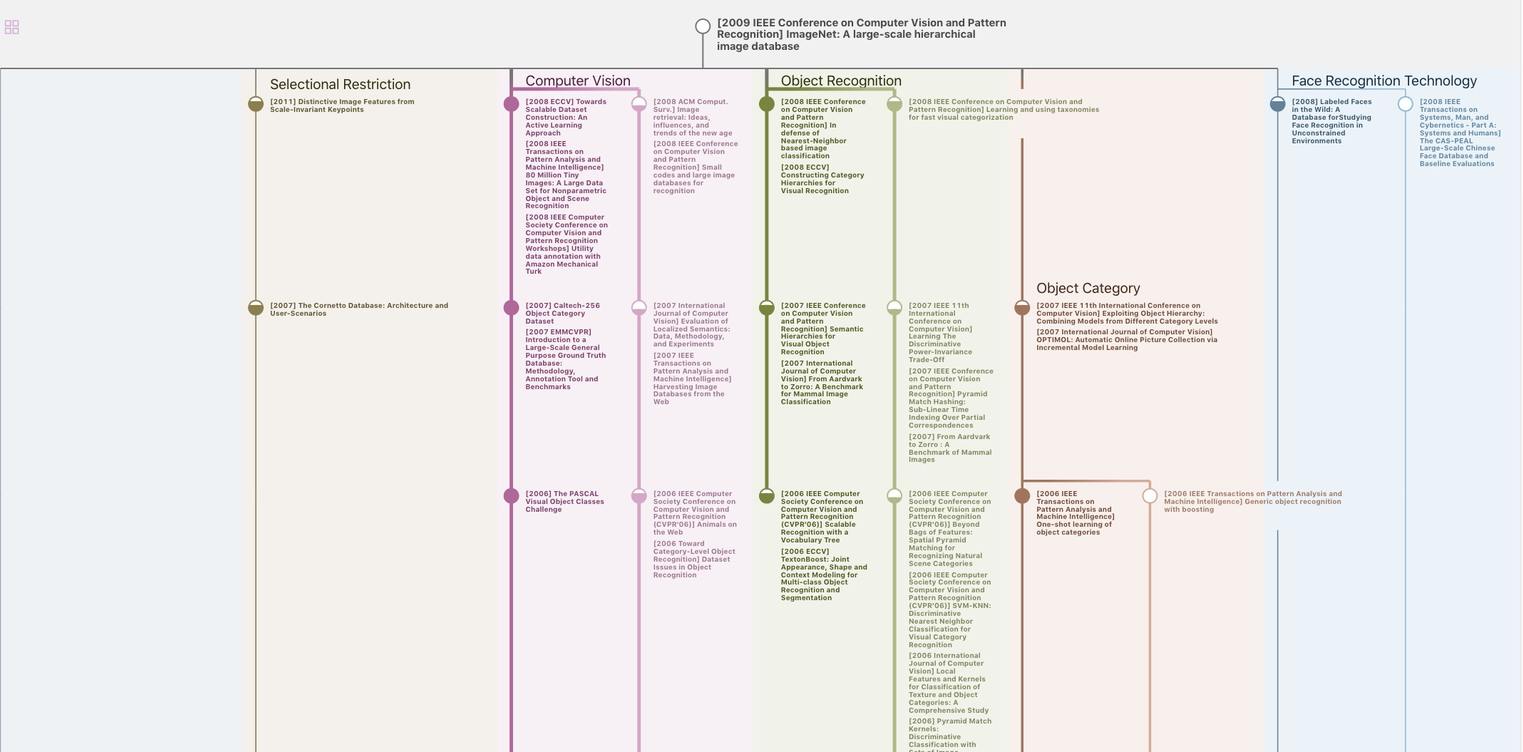
生成溯源树,研究论文发展脉络
Chat Paper
正在生成论文摘要