Digital Twin Enhanced Reinforcement Learning for Integrated Scheduling in Automated Container Terminals*
2023 IEEE 19th International Conference on Automation Science and Engineering (CASE)(2023)
摘要
Container logistics in automated container terminals (ACTs) involves multiple interconnected operational processes. The integrated scheduling of these processes is crucial for the efficiency of the system. Current reinforcement learning (RL) methods struggle to interact effectively with the real world. Digital twin (DT) presents a promising solution to this challenge by providing real-time synchronized simulation. We propose a RL method enhanced by DT to address the integrated scheduling problem in ACTs. The RL model is trained in a DT-based simulation environment, which is more accurate and real-time than traditional simulations. This approach allows for easy transfer of the RL model to a real-world environment. To demonstrate the effectiveness of this approach, we present an experiment in this paper. Our proposed method shows promising results and has the potential to increase container logistics efficiency in ACTs.
更多查看译文
关键词
Automated container terminal,digital twin,reinforcement learning,simulation optimization
AI 理解论文
溯源树
样例
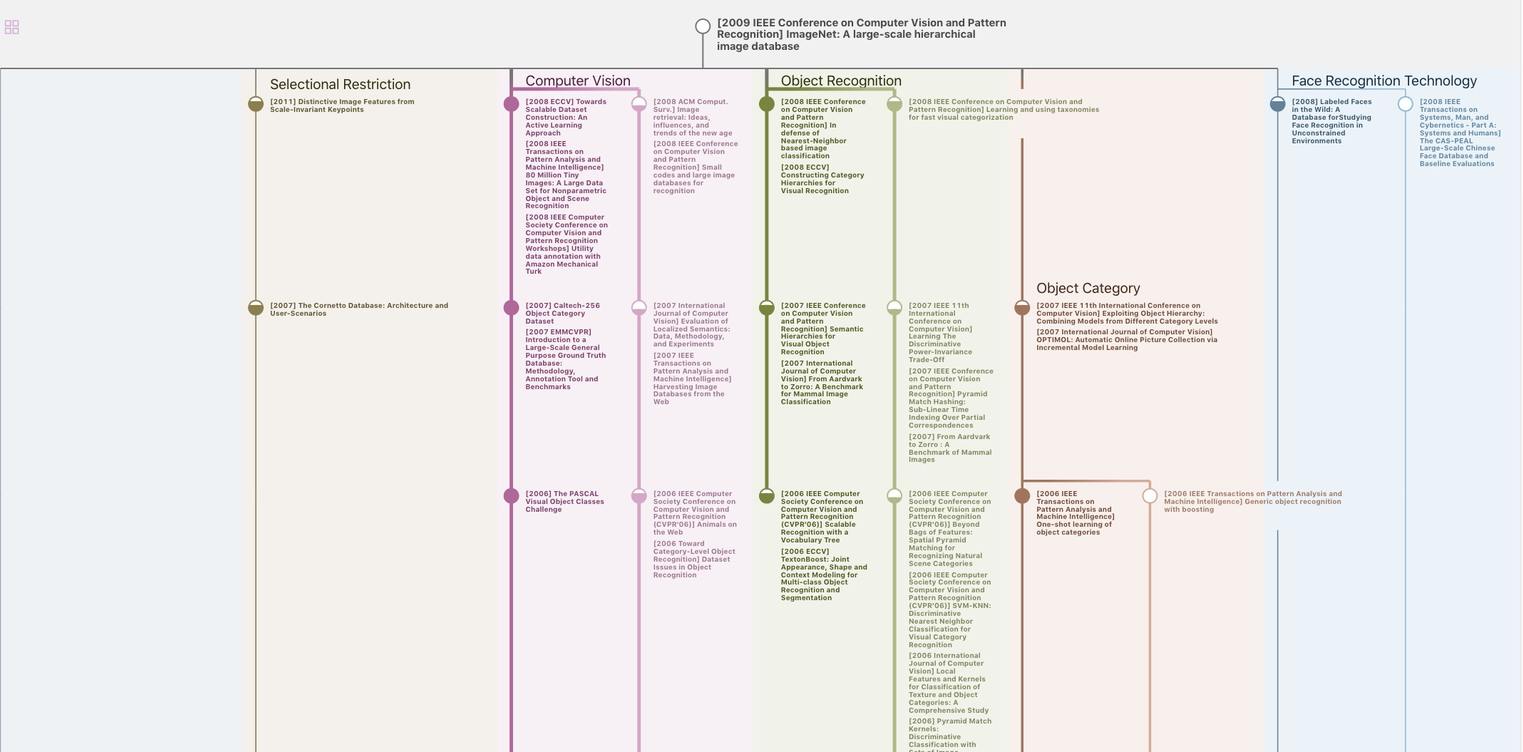
生成溯源树,研究论文发展脉络
Chat Paper
正在生成论文摘要