Multiagent Federated Deep-Reinforcemen Learning-Enabled Resource Allocation for an Air-Ground-Integrated Internet of Vehicles Network
IEEE Internet Computing(2023)
摘要
As an emerging architecture for the future 6G Internet of Vehicles (IoV), the air-ground-integration network has become a paradigm to achieve reliable interconnection everywhere. Unmanned aerial vehicles (UAVs), as low-altitude aerial platforms, can cooperate with ground infrastructures with the advantages of high flexibility and low cost. However, wireless resource allocation for vehicle-to-UAV (V2U) communications has encountered various challenges, such as air-ground spectrum sharing, dynamic topology, fast-changing channels, and time-sensitive services. In this article, we propose a multiagent federated learning and dueling double-deep Q-network (D3QN)-based resource allocation, namely, Fed-D3QN, to jointly optimize channel selection and power control to meet the low latency and reliability requirements of IoV services. Simulation results demonstrate that the proposed Fed-D3QN algorithm has good stability in the highly dynamic air-ground integration network. Additionally, it reduces the total delay of vehicle-to-infrastructure links and improves the transmission success rate of V2U links.
更多查看译文
关键词
multi-agent,air-ground
AI 理解论文
溯源树
样例
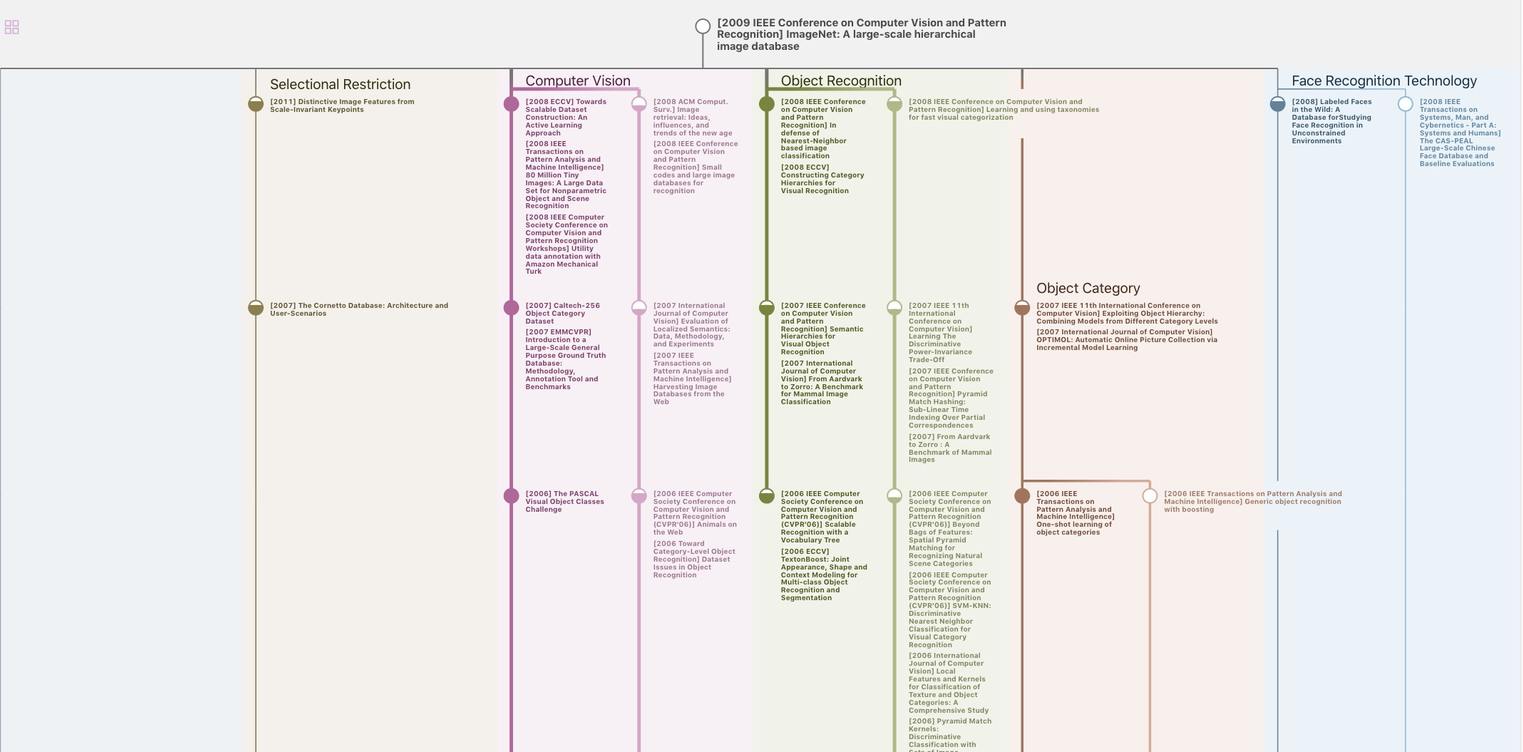
生成溯源树,研究论文发展脉络
Chat Paper
正在生成论文摘要