De Novo Generation of Chemical Structures of Inhibitor and Activator Candidates for Therapeutic Target Proteins by a Transformer-Based Variational Autoencoder and Bayesian Optimization.
Journal of chemical information and modeling(2023)
摘要
Deep generative models for molecular generation have been gaining much attention as structure generators to accelerate drug discovery. However, most previously developed methods are chemistry-centric approaches, and comprehensive biological responses in the cell have not been taken into account. In this study, we propose a novel computational method, TRIOMPHE-BOA (transcriptome-based inference and generation of molecules with desired phenotypes using the Bayesian optimization algorithm), to generate new chemical structures of inhibitor or activator candidates for therapeutic target proteins by integrating chemically and genetically perturbed transcriptome profiles. In the algorithm, the substructures of multiple molecules that were selected based on the transcriptome analysis are fused in the design of new chemical structures by exploring the latent space of a Transformer-based variational autoencoder using Bayesian optimization. Our results demonstrate the usefulness of the proposed method in terms of having high reproducibility of existing ligands for 10 therapeutic target proteins when compared with previous methods. Moreover, this method can be applied to proteins without detailed 3D structures or known ligands and is expected to become a powerful tool for more efficient hit identification.
更多查看译文
关键词
variational autoencoder,therapeutic target proteins,bayesian optimization,inhibitor,transformer-based
AI 理解论文
溯源树
样例
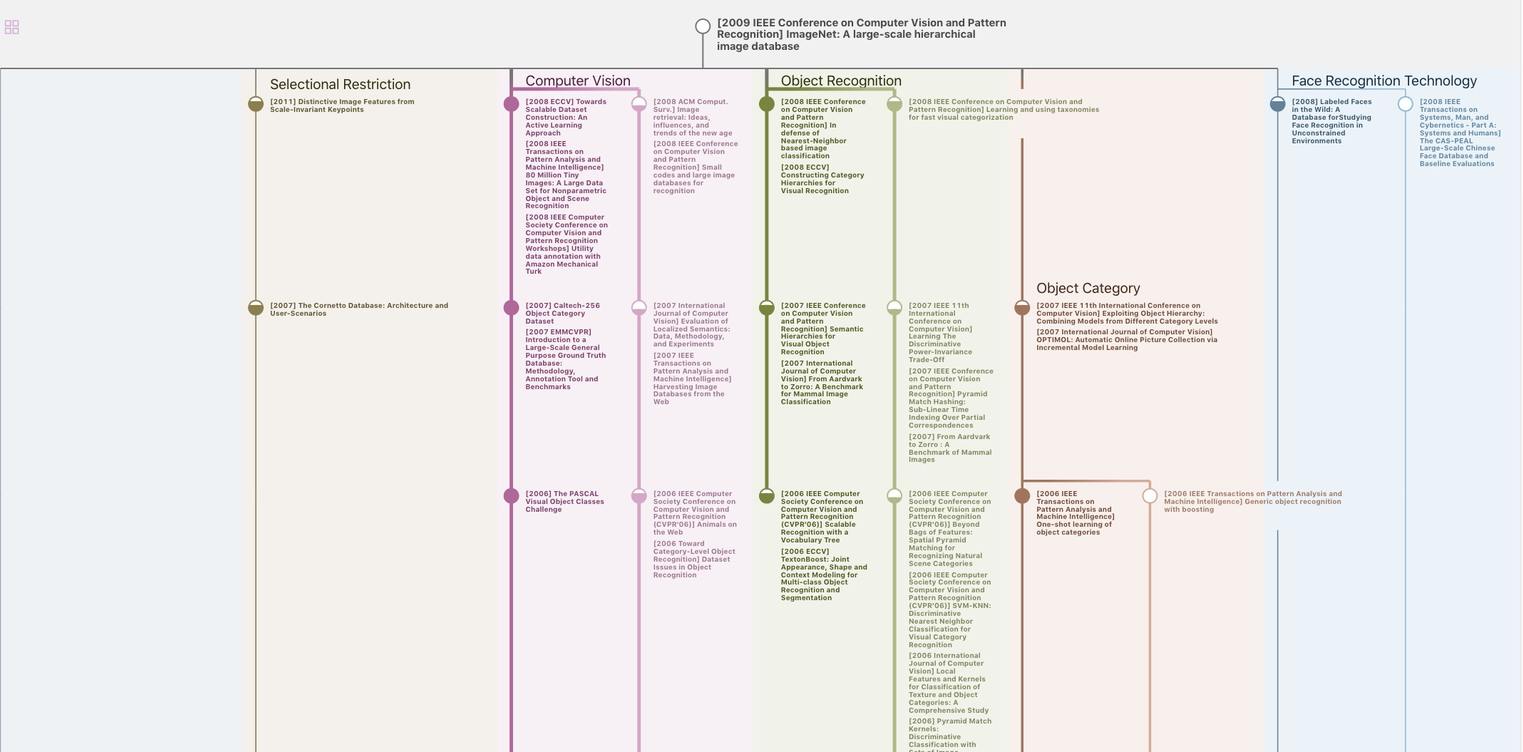
生成溯源树,研究论文发展脉络
Chat Paper
正在生成论文摘要