RealFill: Reference-Driven Generation for Authentic Image Completion
CoRR(2023)
摘要
Recent advances in generative imagery have brought forth outpainting and inpainting models that can produce high-quality, plausible image content in unknown regions, but the content these models hallucinate is necessarily inauthentic, since the models lack sufficient context about the true scene. In this work, we propose RealFill, a novel generative approach for image completion that fills in missing regions of an image with the content that should have been there. RealFill is a generative inpainting model that is personalized using only a few reference images of a scene. These reference images do not have to be aligned with the target image, and can be taken with drastically varying viewpoints, lighting conditions, camera apertures, or image styles. Once personalized, RealFill is able to complete a target image with visually compelling contents that are faithful to the original scene. We evaluate RealFill on a new image completion benchmark that covers a set of diverse and challenging scenarios, and find that it outperforms existing approaches by a large margin. See more results on our project page: https://realfill.github.io
更多查看译文
关键词
authentic image completion,reference-driven
AI 理解论文
溯源树
样例
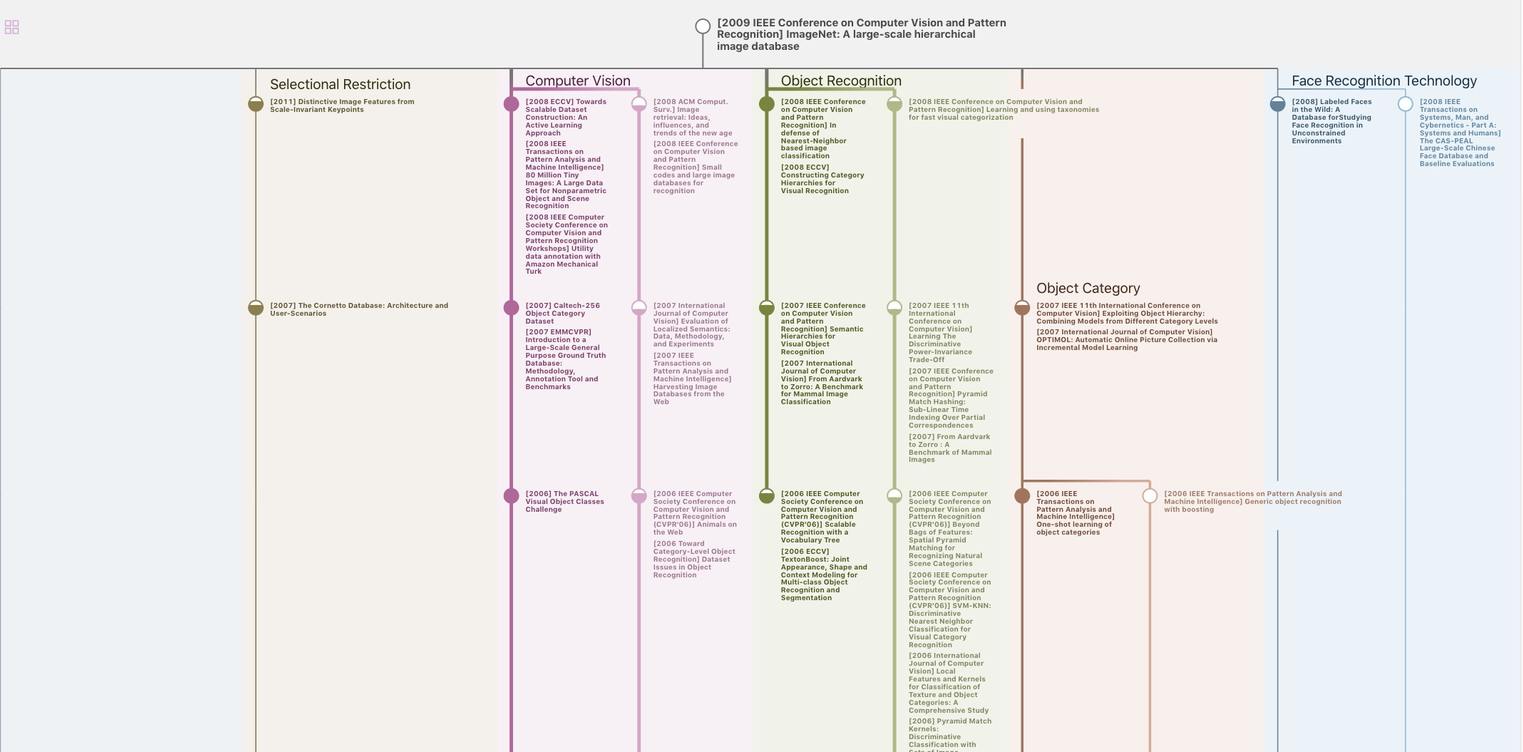
生成溯源树,研究论文发展脉络
Chat Paper
正在生成论文摘要