Correcting for heterogeneity in real-time epidemiological indicators
CoRR(2023)
摘要
Auxiliary data sources have become increasingly important in epidemiological surveillance, as they are often available at a finer spatial and temporal resolution, larger coverage, and lower latency than traditional surveillance signals. We describe the problem of spatial and temporal heterogeneity in these signals derived from these data sources, where spatial and/or temporal biases are present. We present a method to use a ``guiding'' signal to correct for these biases and produce a more reliable signal that can be used for modeling and forecasting. The method assumes that the heterogeneity can be approximated by a low-rank matrix and that the temporal heterogeneity is smooth over time. We also present a hyperparameter selection algorithm to choose the parameters representing the matrix rank and degree of temporal smoothness of the corrections. In the absence of ground truth, we use maps and plots to argue that this method does indeed reduce heterogeneity. Reducing heterogeneity from auxiliary data sources greatly increases their utility in modeling and forecasting epidemics.
更多查看译文
关键词
heterogeneity,real-time
AI 理解论文
溯源树
样例
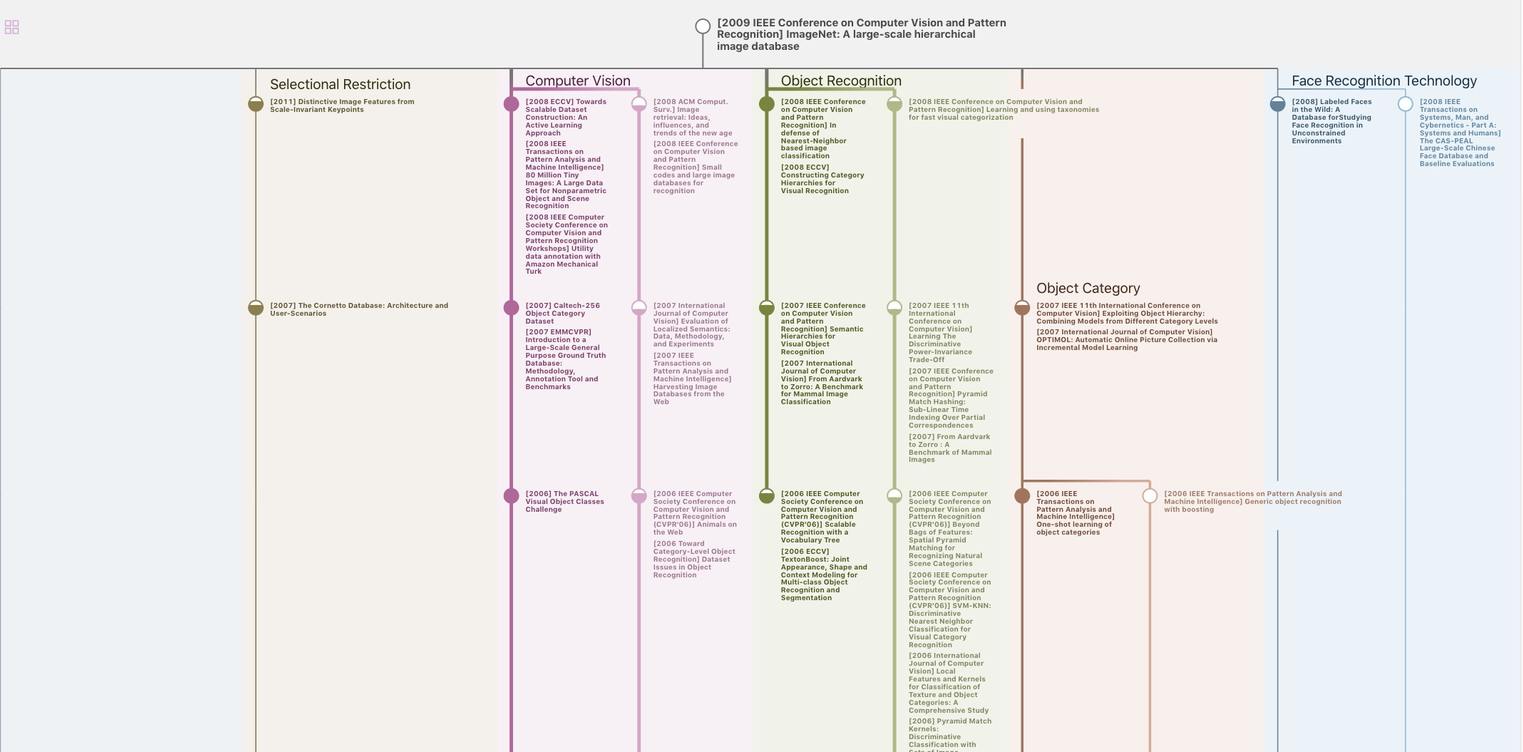
生成溯源树,研究论文发展脉络
Chat Paper
正在生成论文摘要