Human Feedback is not Gold Standard
ICLR 2024(2023)
摘要
Human feedback has become the de facto standard for evaluating the performance of Large Language Models, and is increasingly being used as a training objective. However, it is not clear which properties of a generated output this single `preference' score captures. We hypothesise that preference scores are subjective and open to undesirable biases. We critically analyse the use of human feedback for both training and evaluation, to verify whether it fully captures a range of crucial error criteria. We find that while preference scores have fairly good coverage, they under-represent important aspects like factuality. We further hypothesise that both preference scores and error annotation may be affected by confounders, and leverage instruction-tuned models to generate outputs that vary along two possible confounding dimensions: assertiveness and complexity. We find that the assertiveness of an output skews the perceived rate of factuality errors, indicating that human annotations are not a fully reliable evaluation metric or training objective. Finally, we offer preliminary evidence that using human feedback as a training objective disproportionately increases the assertiveness of model outputs. We encourage future work to carefully consider whether preference scores are well aligned with the desired objective.
更多查看译文
关键词
human evaluation,large language models,evaluation,natural language generation
AI 理解论文
溯源树
样例
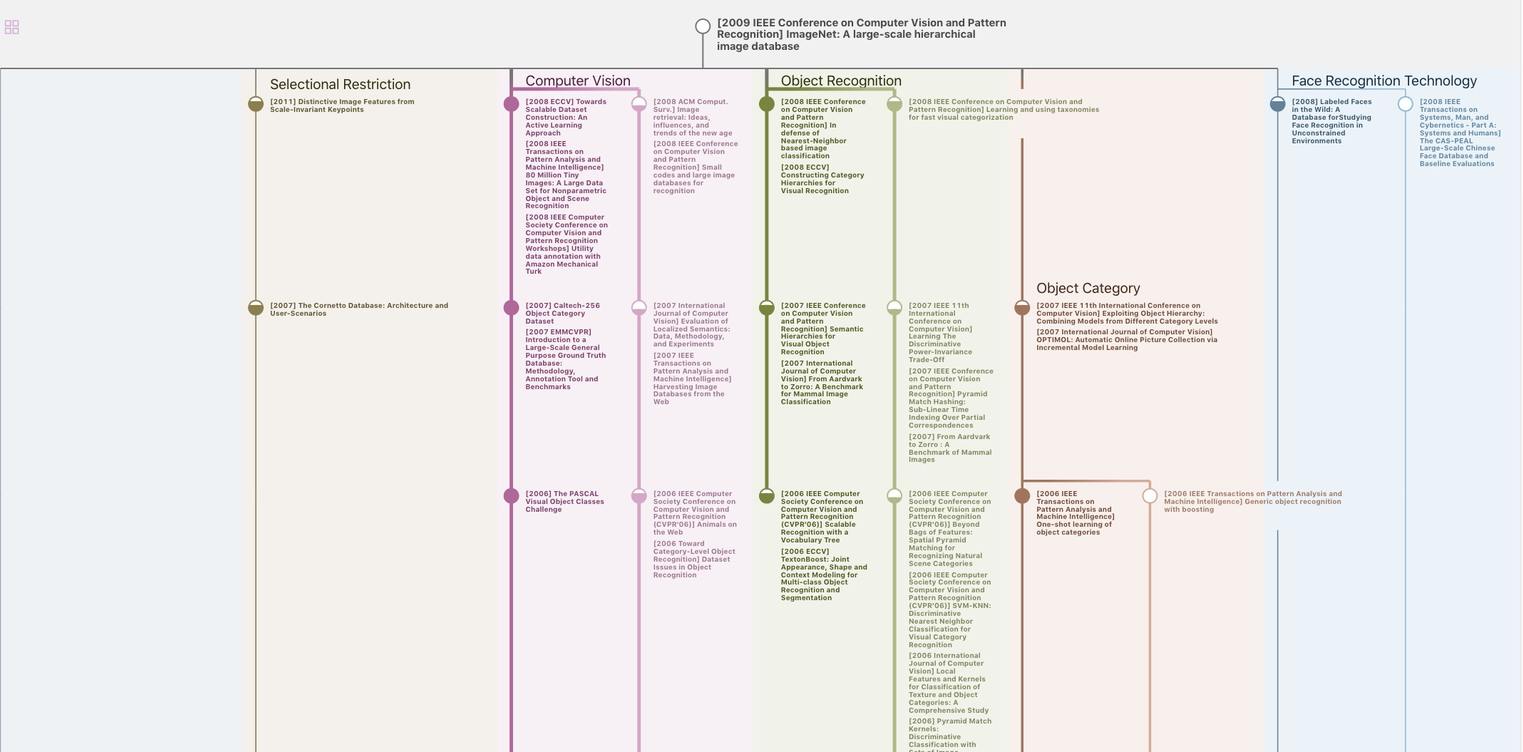
生成溯源树,研究论文发展脉络
Chat Paper
正在生成论文摘要