Forgetting Private Textual Sequences in Language Models via Leave-One-Out Ensemble
ICASSP 2024 - 2024 IEEE International Conference on Acoustics, Speech and Signal Processing (ICASSP)(2023)
摘要
Recent research has shown that language models have a tendency to memorize rare or unique token sequences in the training corpus. After deploying a model, practitioners might be asked to delete any personal information from the model by individuals' requests. Re-training the underlying model every time individuals would like to practice their rights to be forgotten is computationally expensive. We employ a teacher-student framework and propose a novel leave-one-out ensemble method to unlearn the targeted textual sequences that need to be forgotten from the model. In our approach, multiple teachers are trained on disjoint sets; for each targeted sequence to be removed, we exclude the teacher trained on the set containing this sequence and aggregate the predictions from remaining teachers to provide supervision during fine-tuning. Experiments on LibriSpeech and WikiText-103 datasets show that the proposed method achieves superior privacy-utility trade-offs than other counterparts.
更多查看译文
关键词
Language models,memorization,ensemble methods,knowledge distillation,automatic speech recognition
AI 理解论文
溯源树
样例
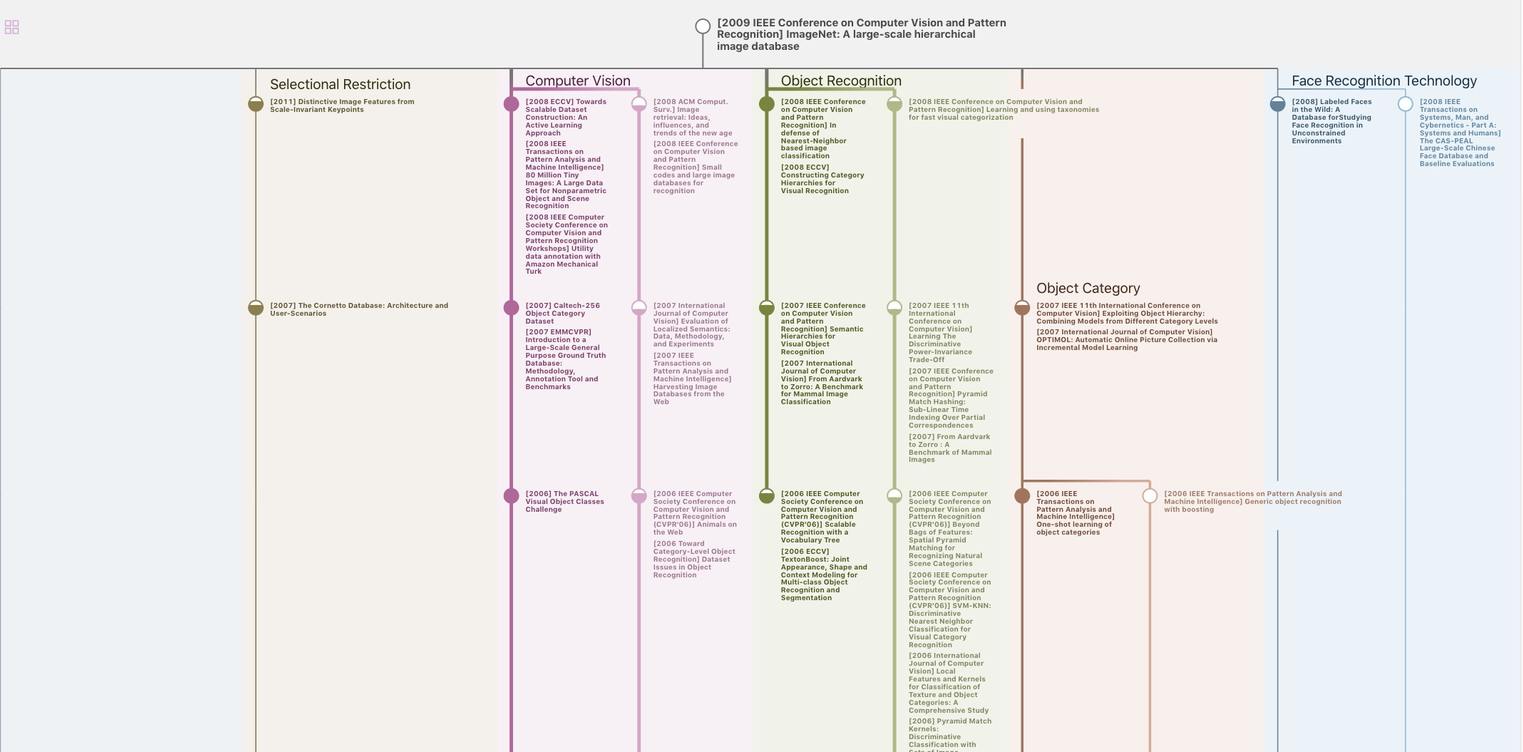
生成溯源树,研究论文发展脉络
Chat Paper
正在生成论文摘要