Multi-Task Learning for Industrial Mobile Robot Perception Using a Simulated Warehouse Dataset
2023 European Conference on Mobile Robots (ECMR)(2023)
摘要
Autonomous industrial mobile robots need advanced perception capabilities to operate safely and human-compliantly in shared working environments. To achieve a high-level understanding of the mobile robots' surroundings, this paper investigates Multi-Task Learning approaches to process multiple tasks simultaneously and potentially improve the generalization performance. Our work alleviates the scarcity of datasets that are relevant for industrial settings by introducing and making publicly available a simulated warehouse dataset (Warehous-eSIM) covering semantic segmentation, depth estimation and surface normals estimation tasks. We collect and examine numerous MTL task-balancing techniques for industrial mobile robot perception. Our experiments show that MTL methods that have shown superior performance on different computer vision datasets fail to improve over the single-task learning setup in our scenario. This implies that the performance of those approaches is very dependent on the considered dataset, which further highlights the value of introducing new relevant datasets focused on industrial mobile robot environments.
更多查看译文
关键词
Multi-task learning (MTL), industrial mobile robots, perception, warehouse dataset
AI 理解论文
溯源树
样例
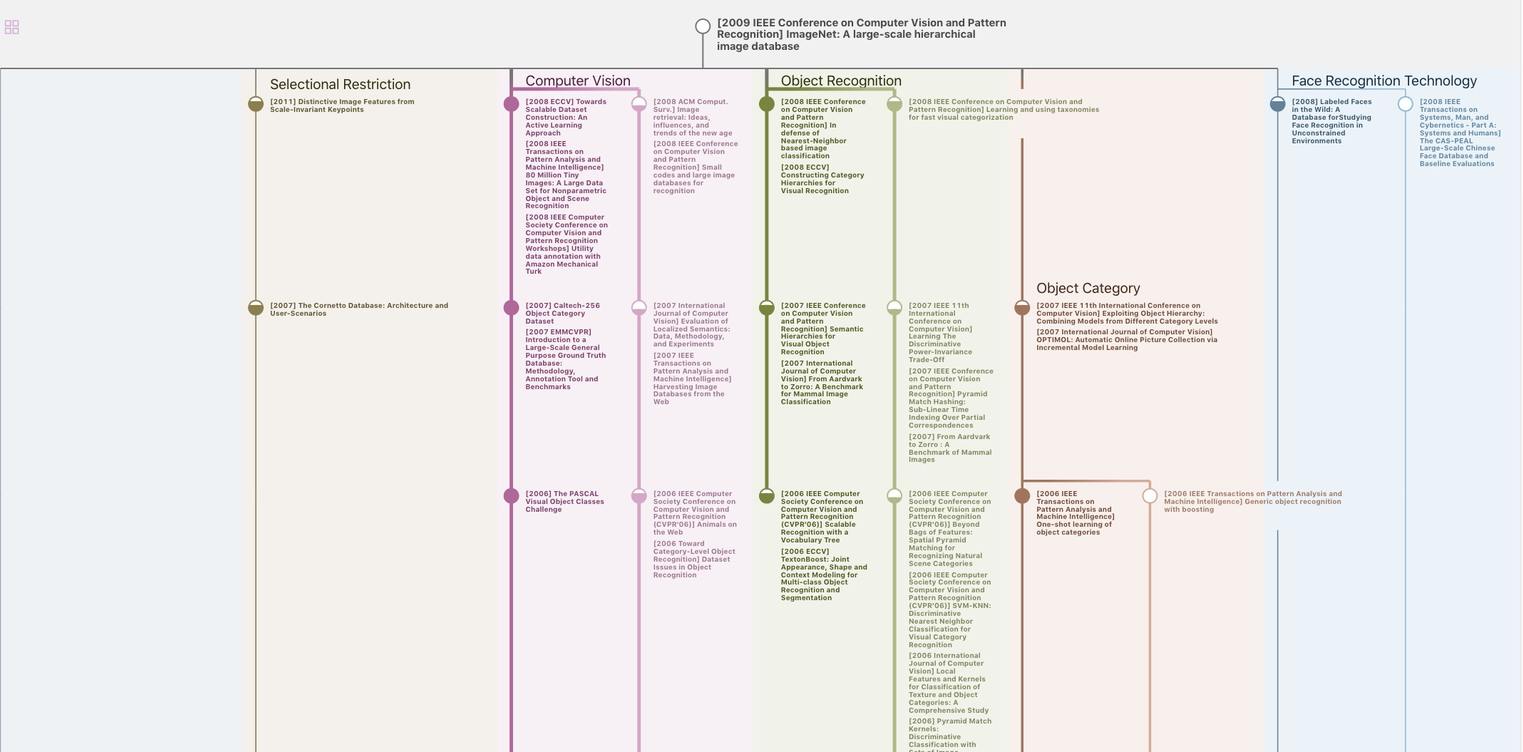
生成溯源树,研究论文发展脉络
Chat Paper
正在生成论文摘要